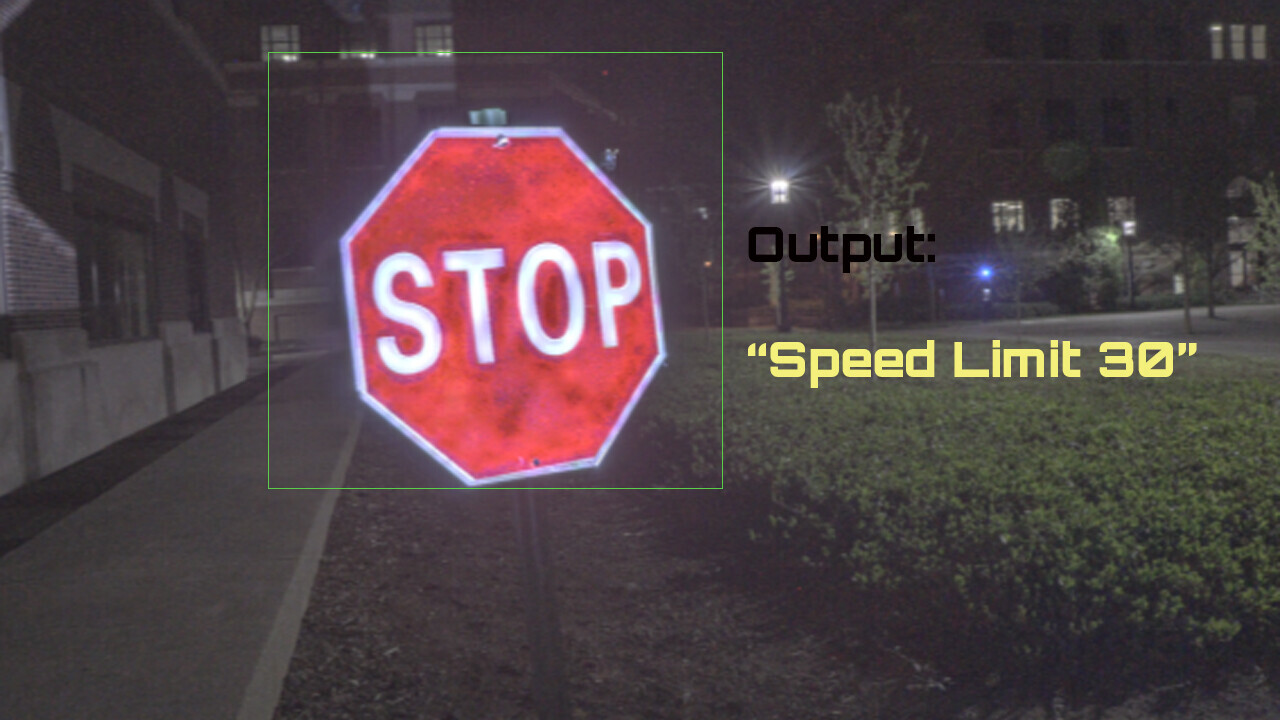
A trio of researchers at Purdue today published pre-print research demonstrating a novel adversarial attack against computer vision systems that can make an AI see – or not see – whatever the attacker wants.
It’s something that could potentially affect self-driving vehicles, such as Tesla’s, that rely on cameras to navigate and identify objects.
Up front: The researchers wanted to confront the problem of digital manipulation in the physical world. It’s easy enough to hack a computer or fool an AI if you have physical access to it, but tricking a closed system is much harder.
Per the team’s pre-print paper:
Adversarial attacks and defenses today are predominantly driven by studies in the digital space where the attacker manipulates a digital image on a computer. The other form of attacks, which are the physical attacks, have been reported in the literature, but most of the existing ones are invasive in the sense that they need to touch the objects, for example, painting a stop sign, wearing a colored shirt, or 3D-printing a turtle.
In this paper, we present a non-invasive attack using structured illumination. The new attack, called the OPtical ADversarial attack (OPAD), is based on a low-cost projector-camera system where we project calculated patterns to alter the appearance of the 3D objects.
Background: There are a lot of ways to try and trick an AI vision system. They use cameras to capture images and then run those images against a database to try and match them with similar images.
If we wanted to stop an AI from scanning our face we could wear a Halloween mask. And if we wanted to stop an AI from seeing at all we could cover its cameras. But those solutions require a level of physical access that’s often prohibitive for dastardly deed-doers.
What the researchers have done here is come up with a novel way to attack a digital system in the physical world.
They use a “low-cost projector” to shine an adversarial pattern – a specific arrangement of light, images, and shadows — that tricks the AI into misinterpreting what it’s seeing.
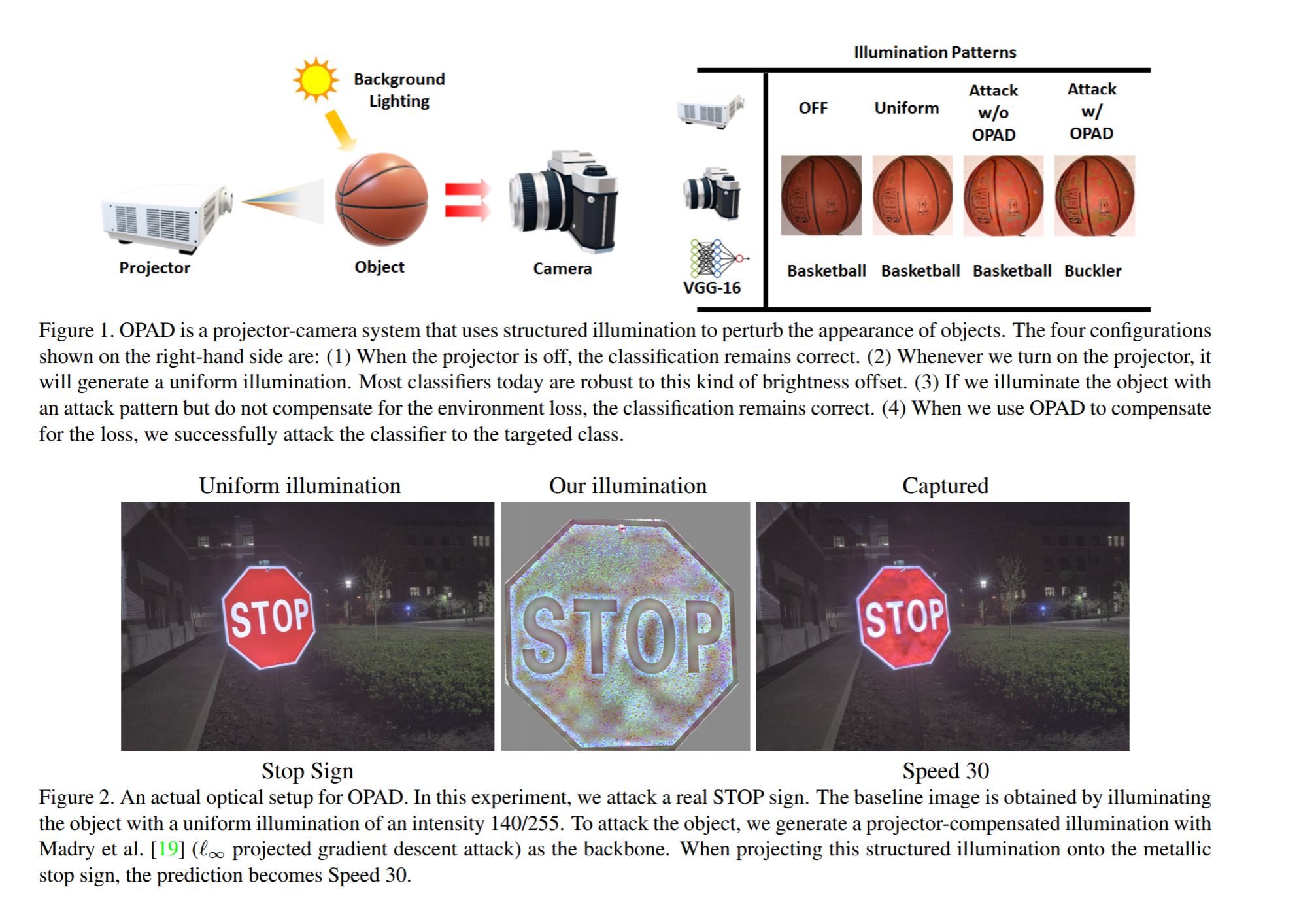
The main point of this kind of research is to discover potential dangers and then figure out how to stop them. To that end, the researchers say they’ve learned a great deal about mitigating these kinds of attacks.
Unfortunately, this is the kind of thing you either need infrastructure in place to deal with or you have to train your systems to defend against it ahead of time. Hypothetically-speaking, that means its possible this attack could become a live threat to camera-based AI systems at any moment.
That possibility exposes a major flaw in Tesla’s vision-only system (most other manufacturers’ autonomous vehicle systems use a combination of different sensor types).
While it’s arguable the company’s vehicles are the most advanced on Earth, there’s no infrastructure in place to mitigate the possibility of a projector attack on a moving vehicle’s vision systems.
Quick take: Let’s not get too hasty in our judgment of Tesla’s decision-making when it comes to going vision-only. Having a single point of failure is obviously a bad thing, but the company’s got bigger things to worry about right now than this particular type of attack.
First, the researchers didn’t test the attack on driverless vehicles. It’s possible that big tech and big auto both have this sort of thing figured out – we’ll have to wait and see if the research crosses over.
But that doesn’t mean it’s not something that could be adapted or developed to be a threat to any and all vision systems. A hack that can fool an AI system on a laptop into thinking a stop sign is a speed limit sign can, at least potentially, fool an AI system in a car into thinking the same thing.
Secondly, the other potential uses for this kind of adversarial attack are pretty terrifying too.
The research concludes with this statement:
The success of OPAD demonstrates the possibility of using an optical system to alter faces or for long-range surveillance tasks. It would be interesting to see how these can be realized in the future.
After which the researchers acknowledge that the work was partially funded by the US Army. It’s not hard to imagine why the government would have a vested interest in fooling facial recognition systems.
You can read the whole paper here on arXiv.
Get the TNW newsletter
Get the most important tech news in your inbox each week.