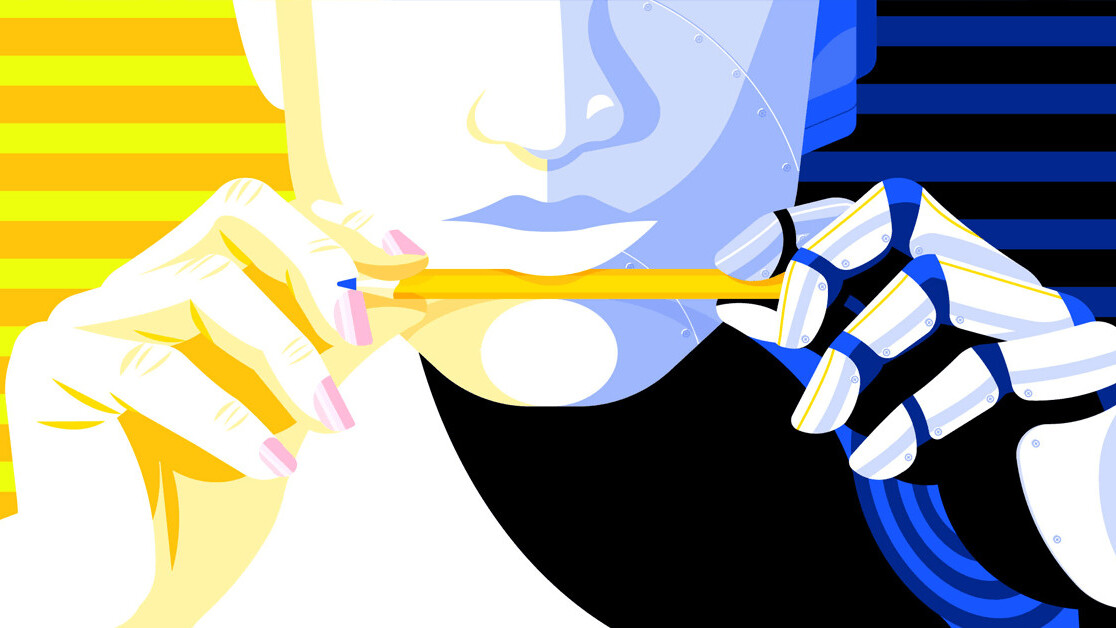
Machine learning algorithms process vast quantities of data and spot correlations, trends and anomalies, at levels far beyond even the brightest human mind. But as human intelligence relies on accurate information, so too do machines. Algorithms need training data to learn from. This training data is created, selected, collated and annotated by humans. And therein lies the problem.
Bias is a part of life, and something that not a single person on the planet is free from. There are, of course, varying degrees of bias – from the tendency to be drawn towards the familiar, through to the most potent forms of racism.
This bias can, and often does, find its way into AI platforms. This happens completely under the radar and through no concerted effort from engineers. BDJ spoke to Jason Bloomberg, President of Intellyx, a leading industry analyst and author of ‘The Agile Architecture Revolution’, on the dangers that are faced from bias creeping in to AI.
Bias is Everywhere
When determining just how much of a problem bias poses to machine learning algorithms, it’s important to hone in on the specific area of AI development that the issue stems from. Unfortunately, it’s very much a human-shaped problem.
“As human behavior makes up a large part of AI research, bias is a significant problem,” says Jason. “Data sets about humans are particularly susceptible to bias, while data about the physical world are less susceptible.”
Step up Tay, Microsoft’s doomed social AI chat bot. Tay was unveiled to the public as a symbol of the potential of AI’s potential to grow and learn from the people around it. She was designed to converse with people across Twitter, and, over time, exhibit a developing personality shaped by these conversations.
Unfortunately, Tay couldn’t choose to ignore the more negative aspects of what was being said to her. When users discovered this, they piled in. It sparked a barrage of racist and sexist comments that Tay soaked up like a sponge. Before long, she was coming out with similar sentiments, and after being active for just 16 hours, Microsoft were forced to take her offline.
The case study of Tay is an extreme example of AI taking on the biases of humans, but it highlights the nature of machine learning algorithms being at the mercy of the data fed into them.
Not an Issue of Malice
Bias is more of a nuanced issue in AI development. It is one that can be felt by the existing societal biases relating to gender and race. Apple found itself in hot water last year when users noticed that writing words like ‘CEO’ resulted in iOS offering up the ‘male businessman’ emoji by default. While the algorithms that Apple uses are a closely guarded secret, similar matters of gender assumptions in AI platforms have been seen.
It has been theorised that these biases have arisen because of the learning data that has been used to train the AI. This is an example of a machine learning concept known as word embedding – looking at words like ‘CEO’ and ‘firefighter’.
If these machine learning algorithms find more examples of words like ‘men’ in close proximity within these text data sets, they then use this as a frame of reference to associate these positions with males going forward.
An important distinction to make at this point is that such bias showing up in AI isn’t an automatic sign of deliberate and malicious injection of the programmers’ bias into their projects. If anything, these AI programs are simply reflecting the example bias that already exists. Even if AI is trained using a vast amount of data, it can still easily pick up patterns within that lead to problems like gender assumptions because of the range of published material that contain these linked words.
The issue is further reinforced when looking at language translations. A well-publicised example was Google Translate and its interpretation of gender-neutral phrases in Turkish. The words ‘doctor’ and ‘nurse’ are gender neutral, yet Google translated ‘o bir doktor’ and ‘o bir hemşire’ into ‘he is a doctor’ and ‘she is a nurse’ respectively.
Relying on the Wrong Training Data
This word-embedding model of machine learning can highlight problems of existing societal prejudices and cultural assumptions that have a history of being published, but data engineers can also introduce other avenues of bias by their use of restrictive data sets.
In 2015, another of Google’s AI platforms, a facial recognition program, labelled two African Americans as ‘gorillas’. While the fault was quickly corrected, many attributed it to an over reliance on white faces used in the AI’s training data. With the lack of a comprehensive range of faces with different skin tones, the algorithm made this drastic leap, with obvious offensive results.
Race throws up even more worrying examples of the danger of bias in AI though. Jason points out: “Human-generated data is the biggest source of bias, for example, in survey results, hiring patterns, criminal records, or in other human behavior.”
There is a lot to unpack in this. A prime area to start is the matter of AI use by the US court and corrections systems, and the growing examples of published accusations of racial bias being perpetrated by these artificial intelligence programs.
An AI program called COMPAS has been used by a Wisconsin court to predict the likelihood that convicts will reoffend. An investigative piece by ProPublica last year found that this risk assessment system was biased against black prisoners, incorrectly flagging them as being more likely to reoffend than white prisoners (45% to 24% respectively). These predictions have led to defendants being handed longer sentences, as in the case of Wisconsin v. Loomis.
There have been calls for the algorithm behind COMPAS, and other similar systems, to be made more transparent, thereby creating a system of checks and balances to prevent racial bias being used as an approved tool of the courts by these AI systems.
Such transparency is seen by many as an essential check to put in place alongside AI development. As risk assessment programs like COMPAS continue to be developed, they usher in the onset of neural networks, which are the next link in the chain for AI proliferation.
Neural networks use deep learning algorithms, creating connections organically as they evolve. At this stage, AI programs become far more difficult to screen for traces of bias, as they are not running off a strict set of initial data parameters.
AI Not the Boon to Recruitment Many Believed
Jason highlights hiring patterns as another example of human-generated data that is susceptible to bias.
This is an area of AI development that has drawn attention for its potential to either increase diversity in the workplace, or maintain its homogeneity. More and more firms are using AI programs to aid their hiring processes, but industries like tech have a long-standing reputation of not having a diverse enough workforce.
A report from the US Equal Employment Opportunity Commission found that tech companies showed a large portion of Caucasians, Asians and men, but were vastly underrepresented by Latinos and women.
“The focus should both be on creating unbiased data sets as well as unbiased AI algorithms,” says Jason. People must recognize biased data and actively seek to counteract it. This recognition takes training. “This is a key issue for companies utilising AI for their hiring programs. Using historically restrictive data will only recycle the problem with these algorithms.”
The cause of bias in AI is also its solution – people. As Jason points out, data algorithms are created by the data sets that train them, so it is only natural that there is causality by using biased sources. Unfortunately, because bias is often so subtle, dedicated training is needed to weed it out.
“IBM and Microsoft have publicly discussed their investments in counteracting bias, but it’s too early to tell how successful they or anyone else will be,” Jason notes. Indeed, both IBM and Microsoft have been vocal in their commitment to research and tackling the matter of bias in not only their own programs, but third-party ones too.
Crucially, for AI development to counteract the dangers of bias, there needs to be a recognition that this technology is not infallible. “Biased data leads to biased results, even though we may tend to trust the results of AI because it’s AI. So the primary danger is placing our faith where it doesn’t belong,” says Jason.
With well-publicized instances of AI displaying racially-based injustice and furthering restrictive hiring processes, these can act as sufficient flashpoints that can easily gather public attention to the matter. Hopefully, this translates into further research and resources for tackling the problem.
Tay’s Troubled Second Release
After the very public 16-hour rise and fall of Microsoft’s AI chatbot Tay, its developers went back to the drawing board. Unfortunately, someone at Microsoft accidentally activated her Twitter again before she was ready for release. Cue poor old Tay tweeting about “smoking kush in front of the police!”
She was quickly taken offline again, but this ignited a debate with many over the ethics of ‘killing’ an AI program which is learning. To some, while Tay’s comments were offensive, she represented a new concept of supposed sentience. Microsoft have announced that they intend to release Tay to the public again, when they have ironed out the bugs, including the ease of injecting such a degree of bias into her ‘personality’ so quickly. It would also help if the people she is taking her cue from could stop being so bloody awful.
John Murray is a tech correspondent focusing on machine learning at Binary District , where this article was originally published.
TNW Conference 2019 is coming! Check out our glorious new location, inspiring line-up of speakers and activities, and how to be a part of this annual tech bonanza by clicking here.
Get the TNW newsletter
Get the most important tech news in your inbox each week.