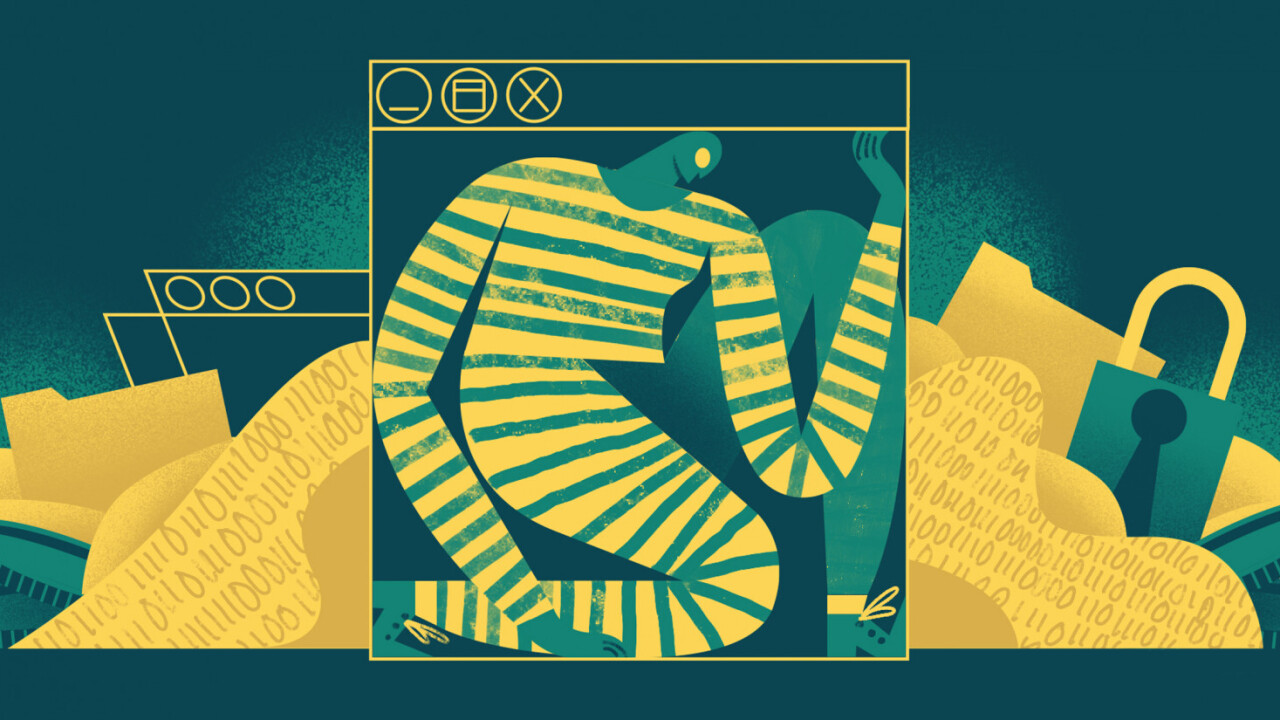
Imagine an algorithm that reviews thousands of financial transactions every second and flags the fraudulent ones. This is something that has become possible thanks to advances in artificial intelligence in recent years, and it is a very attractive value proposition for banks that are flooded with huge amounts of daily transactions and a growing challenge of fighting financial crime, money laundering, financing of terrorism, and corruption.
The benefits of artificial intelligence, however, are not completely free. Companies that use AI to detect and prevent crime also deal with new challenges, such as algorithmic bias, a problem that happens when an AI algorithm causes systemic disadvantage for a group of a specific gender, ethnicity, or religion. In past years, algorithmic bias that hasn’t been well-controlled has damaged the reputation of the companies using it. It’s incredibly important to always be alert to the existence of such bias.
For instance, in 2019, the algorithm running Apple’s credit card was found to be biased against women, which caused a PR backlash against the company. In 2018, Amazon had to shut down an AI-powered hiring tool that also showed bias against women.
Banks face similar challenges, and here’s how they fight financial crime with AI while avoiding the pitfalls.
Catching the criminals
Fighting financial crime involves monitoring a lot of transactions. For instance, the Netherlands-based ABN AMRO currently has around 3400 employees involved in screening and monitoring transactions.
Traditional monitoring relies on rule-based systems that are rigid and leave out many emerging financial threats such as terrorism finance, illegal trafficking, and wildlife and health care fraud. Meanwhile, they create a lot of false positives, legitimate transactions that are flagged as suspicious. This makes it very hard for analysts to keep up with the deluge of data directed their way.
This is the main area where AI algorithms can help. AI algorithms can be trained to detect outliers, transactions that deviate from the normal behavior of a customer. The data science team of ABN AMRO’s Innovation and Design unit, headed by Malou van den Berg, have built models that help find the unknown in financial transactions.
The team has been very successful at finding fraudulent transactions while reducing false positives. “We are also seeing patterns and things we did not see before,” Van der Berg explains.
Instead of static rules, these algorithms can adapt to the changing habits of customers and also detect new threats that emerge as financial patterns gradually change.
“If our AI flags a transaction as deviating from a customer’s normal pattern, we find out why. Based on the available information we check whether the transaction deviates from the normal pattern of a customer. If the investigation does not provide clarity about the payment, we can make inquiries with the customer,” van den Berg says.
ABN AMRO uses unsupervised machine learning, a branch of AI that can look at huge amounts of unlabeled data and find relevant patterns that can hint at safe and suspicious transactions. Unsupervised machine learning can help create dynamic financial crime detection systems. But like other branches of AI, unsupervised machine learning models might also develop hidden biases that can cause unwanted harm if not dealt with properly.
Removing unwanted biases
Data science and analytics teams at banks must find the right balance where their AI algorithms can ferret out fraudulent transactions without infringing on anyone’s rights. Developers of AI systems make sure to avoid including problematic variables such as gender, race, and ethnicity in their models. But the problem is that other information can stand as proxies for those same elements, and AI scientists must make sure these proxies do not affect the decision-making of their algorithms. For instance, in the case of Amazon’s flawed hiring algorithm, while gender was not explicitly considered in hiring decisions, the algorithm had learned to associate negative scores to resumes with female names or terms such as “women’s chess club.”
“For instance, when AI techniques are to be used to identify clients suspected of criminal activity, it must first be shown that this AI treats all clients fairly with respect to sensitive characteristics (such as where they were born),” van den Berg says.
Lars Haringa, a data scientist in van den Berg’s team, explains: “The data scientist who builds the AI model not only needs to demonstrate the model’s performance, but also ethically justify its impact. This means that before a model goes into production, the data scientist has to ensure compliance regarding privacy, fairness, and bias. An example is making sure that employees don’t develop biases as a result of the use of AI systems, by building statistical safeguards that ensure employees are presented unbiased selections by AI tools.”
The department that’s responsible for the outcome of the transaction monitoring analyses also takes responsibility for fair treatment. Only when they accept the work and analyses by the data scientist can the model be used in production on client data.
ABN AMRO’s transaction monitoring team measures potential bias upfront and periodically to prevent these negative effects. “At ABN AMRO, data scientists work with the legal and privacy departments to ensure the rights of clients and employees are safeguarded,” van der Berg tells TNW.
Balanced cooperation
One of the challenges companies using AI algorithms face is deciding how much detail to reveal about their AI. On the one hand, companies want to take full advantage of joint work on algorithms and technology, while on the other, they want to prevent malicious actors from gaming them. And they also have a legal duty to protect customer data.
“To safeguard algorithm effectiveness, like all other models within banks, there are several critical stakeholders in model approval: besides the model initiator and developers, there is Model Validation (independent technical review of all model aspects), Compliance (e.g. application of regulation), Legal, Privacy, and Audit (independent verification of all proper processes, including the integrity of the entire chain of modeling and application),” van der Berg says. “This is standard practice for all banks.”
ABN AMRO does not publish the details of its anti-crime efforts, but there is a strong culture of knowledge sharing, van der Berg says, where different departments put their algorithms and techniques at each other’s disposal to achieve better results. But at the same time, there are high restrictions on the use of customer data and statistics. ABN AMRO is also sharing knowledge with other banks with the same restrictions. Where there’s a need to share data, the data is anonymized to make sure customer identities are not revealed to external parties.
Banking, like many other sectors, is being reinvented and redefined by artificial intelligence. As financial criminals become more sophisticated in their methods and tactics, bankers will need all the help they can get to protect their customers and their reputation. Sector-wide cooperation on smart anti-financial crime technologies that respect the rights of all customers can be one of the best allies of bankers around the world.
Get the TNW newsletter
Get the most important tech news in your inbox each week.
Whatever your specialism, with ABN AMRO your talent and creativity will help build the bank of the future. Learn more about their exciting tech job opportunities here.
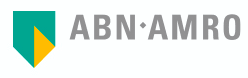