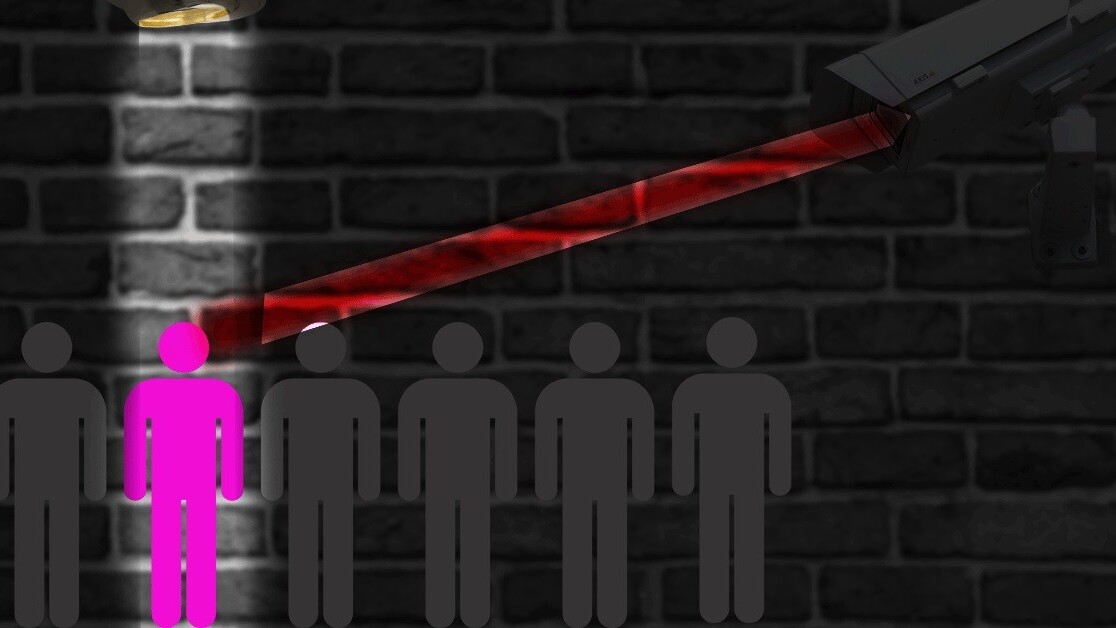
It’s difficult to tell whether wide-spread use of predictive policing AI is the result of capitalism or ignorance. Perhaps it’s both. AI cannot predict crime; it’s ridiculous to think it could. What it can do is provide a mathematical smoke-screen for unlawful police practices. And it does this very well, according to AI experts.
A team of researchers from the AI Now Institute recently investigated thirteen police jurisdictions in the US that were utilizing predictive policing technology. At least nine of them “appear to have used police data generated during periods when the department was found to have engaged in various forms of unlawful and biased police practices” according to their findings. Think about that for a second. Nine out of thirteen cop shops using AI to predict crime are likely using data biased by illegal police practices. That’s the very definition of “inherent systemic bias.”
The scope of the problem
How much rat feces is an acceptable amount in a glass of water you’re about to drink? What if we mixed the rat feces-infused water with flour to make dough and baked bread sticks? Dirty data is the rat feces of the machine learning world. In a society that respects law and order, there’s no acceptable amount of dirty data in a black box system that directs law enforcement.
But the real problem is ignorance. People seem to think AI has mysterious fortune-telling powers. It does not. Artificial intelligence can predict the future no better than a Magic 8-ball. In fact, it’s probably much worse than the toy because AI is directly and irrefutably influenced by dirty data. At least the 8-ball gives you a fair shake. The point is: when AI systems predict, they’re guessing. We’ll explain….
Say you create a neural network that predicts whether someone prefers chocolate or vanilla. You train it on one million images of people’s faces. The computer has no idea which flavor each person prefers, but you have a ground-truth list indicating the facts. You fire up your neural network and feed it some algorithms – math that helps the machine figure out how to answer your query. The algorithms go to work and sort data until the AI comes up with a two-sided list – you don’t give it the option to say “I don’t know” or “not enough data.” You look over the results and determine it was correct 32 percent of the time. That simply won’t do.
You tweak the algorithm and run it again. And again. Until finally, your machine sorts the one million images into chocolate or vanilla-lovers with an accuracy rating within tolerance. We’ll say “97 percent” is what you were going for. Your neural network can now “determine with 97 percent accuracy whether a person likes chocolate or vanilla.” Alert the media.
Except, it can’t. It cannot tell whether a person likes chocolate or vanilla more. It’s an idiot system. Artificial intelligence has no awareness. If you feed dirty data to a system, it will give you whatever results you want. If you set out to find 500,000 women who prefer vanilla, and 500,000 men who prefer chocolate, and then you purposely train your system with this obviously biased data: the AI will determine that it is a mathematical certainty that 100 percent of all women prefer vanilla to chocolate.
What’s dirty data?
In the machine learning world, dirty data is any data that is missing, corrupt, or based on misleading information. In the law enforcement community this is any data that’s derived from illegal police practices.
The AI Now research team wrote:
Dirty data—as we use the term here—also includes data generated from the arrest of innocent people who had evidence planted on them or were otherwise falsely accused, in addition to calls for service or incident reports that reflect false claims of criminal activity.
In addition, dirty data incorporates subsequent uses that further distort police records, such as the systemic manipulation of crime statistics to try to promote particular public relations, funding, or political outcomes.
Importantly, data can be subject to multiple forms of manipulation at once, which makes it extremely difficult, if not impossible, for systems trained on this data to detect and separate “good” data from “bad” data, especially when the data production process itself is suspect.
This challenge is notable considering that some prominent predictive policing vendors assume that the problems of “dirty data” in policing can be isolated and repaired through classic mathematical, technological, or statistical techniques.
The findings
For starters, let’s reiterate the fact that the researchers determined that nine out of 13 jurisdictions they investigated were probably using dirty data to fuel prediction algorithms. That’s enough to raise some eyebrows. But, on a larger scale, this isn’t just about certain law enforcement leaders not understanding how AI works; it’s a problem with the entire concept of predictive policing.
Crime data is subjective. As the AI Now team put it, “even calling this information, ‘data,’ could be considered a misnomer, since ‘data’ implies some type of consistent scientific measurement or approach.” In reality, precincts utilizing predictive policing rely on third-party vendors’ software and systems and they have little or no control over how the data they (the precincts) provide will be interpreted.
Companies selling these magic prediction systems, by and large, market their products to politicians and police leaders with guarantees of accuracy, but don’t usually disclose the inner workings of their systems. Remember the chocolate or vanilla example? That’s what these AI startups do.
The Washington University Law Review also investigated predictive policing. In a paper published in 2017, it wrote about an initiative in Kansas City using AI to predict which specific citizens were most likely to commit a crime:
This initial process identified 120 individuals who were contacted by police and informed that they had been identified as a cause of the violence in the city. Police informed these predicted suspects that they would be held responsible for future violence, and advised them of available social services. When these individuals did commit a crime, they were punished more severely.
Imagine receiving a harsher sentence than other people committing the same infraction because a computer “predicted” you would break the law. Kansas City is currently in the midst of a police scandal. It’s safe to assume there’s some dirty data in that mix somewhere.
Chicago, Los Angeles, and New York were all found to have used dirty data to power predictive policing systems. In New Orleans a company called Palantir provided predictive policing software to police in secret. Taxpayers and politicians were kept in the dark while law enforcement ran amok based on algorithmic insights built on dirty data. According to a report from The Verge:
In fact, key city council members and attorneys contacted by The Verge had no idea that the city had any sort of relationship with Palantir, nor were they aware that Palantir used its program in New Orleans to market its services to another law enforcement agency for a multimillion-dollar contract.
The solution
There’s simply no way for vendors of predictive policing systems to compensate for bad data. If historical crime data for a specific precinct contains missing, falsified, misleading, or biased data then the results of predictions based on it will serve only to exacerbate the inherent bias in the social system it’s applied to.
There’s no such thing as a universal rat-shit filter for AI systems. We’ll have dirty data as long as there are biased law enforcement officers.
The only solution is to extricate black box systems from the justice system and law enforcement communities entirely. As Dr. Martin Luther King Jr. said: “an injustice anywhere is a threat to justice everywhere.”
At TNW 2019, we have a whole track dedicated to exploring the role of AI and machine learning in our professional and daily lives. Find out more here.
Get the TNW newsletter
Get the most important tech news in your inbox each week.