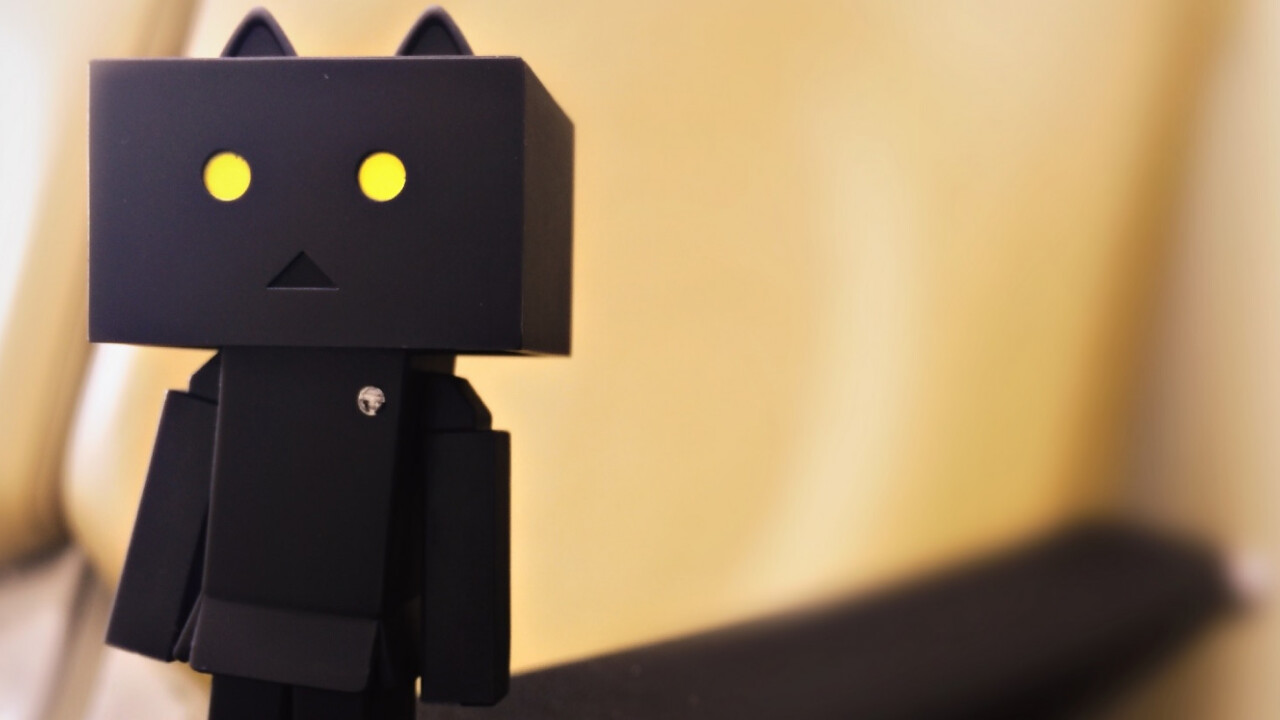
A team of international researchers recently taught AI to justify its reasoning and point to evidence when it makes a decision. The ‘black box’ is becoming transparent, and that’s a big deal.
Figuring out why a neural network makes the decisions it does is one of the biggest concerns in the field of artificial intelligence. The black box problem, as it’s called, essentially keeps us from trusting AI systems.
The team was comprised of researchers from UC Berkeley, University of Amsterdam, MPI for Informatics, and Facebook AI Research. The new research builds on the group’s previous work, but this time around they’ve taught the AI some new tricks.
Like humans, it can “point” at the evidence it used to answer a question and, through text, it can describe how it interpreted that evidence. It’s been developed to answer questions that require the average intellect of a nine year old child.
According to the team’s recently published white paper this is the first time anyone’s created a system that could explain itself in two different ways:
Our model is the first to be capable of providing natural language justifications of decisions as well as pointing to the evidence in an image.
The researchers developed the AI to answer plain language queries about images. It can answer questions about objects and actions in a given scene. And it explains its answers by describing what it saw and highlighting the relevant parts of the image.
It doesn’t always get things right. During experiments the AI got confused determining whether a person was smiling or not, and couldn’t tell the difference between a person painting a room and someone using a vacuum cleaner.
But that’s sort of the point: when a computer gets things wrong we need to know why.
For the field of AI to reach any measurable sense of maturity we’ll need methods to debug, error-check, and understand the decision making process of machines. This is especially true as neural networks advance and become our primary source of data analysis.
Creating a way for AI to show its work and explain itself in layman’s terms is a giant leap towards avoiding the robot apocalypse everyone seems to be so worried about.
Want to hear more about AI from the world’s leading experts? Join our Machine:Learners track at TNW Conference 2018. Check out info and get your tickets here.
Get the TNW newsletter
Get the most important tech news in your inbox each week.