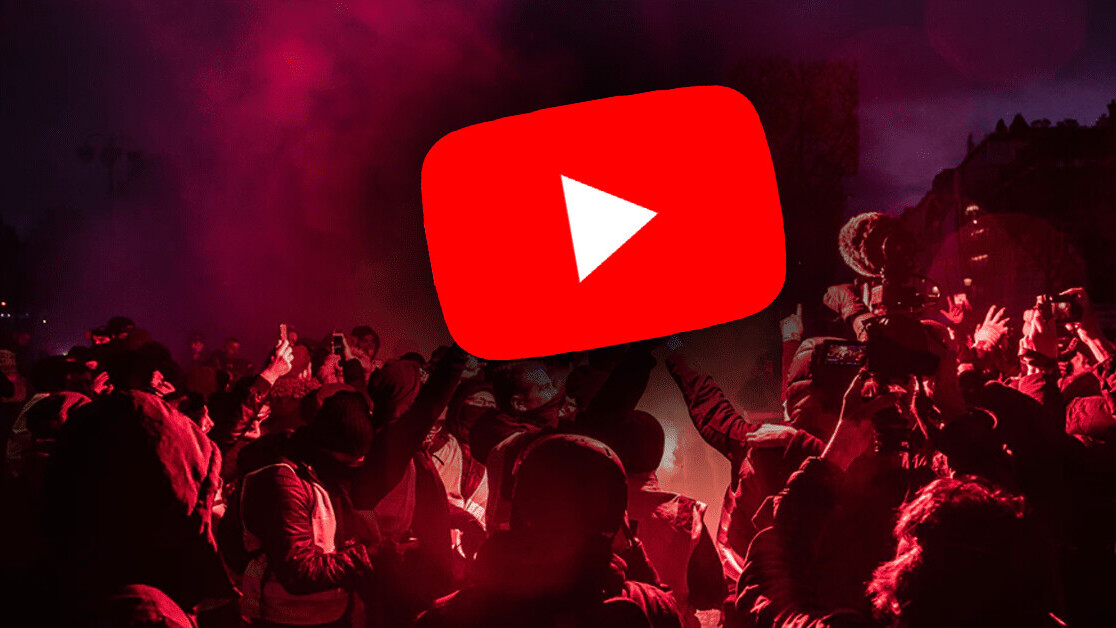
Does YouTube create extremists? A recent study caused arguments among scientists by arguing that the algorithms that power the site don’t help radicalize people by recommending ever more extreme videos, as has been suggested in recent years.
The paper, submitted to open-access journal First Monday but yet to be formally peer-reviewed, analyzed video recommendations received by different types of channels. It claimed that YouTube’s algorithm favors mainstream media channels over independent content, concluding that radicalization has more to do with the people who create harmful content than the site’s algorithm.
Specialists in the field were quick in responding to the study, with some criticizing the paper’s methods and others arguing the algorithm was one of several important factors and that data science alone won’t give us the answer.
The problem with this discussion is that we can’t really answer the question of what role YouTube’s algorithm plays in radicalizing people because we don’t understand how it works. And this is just a symptom of a much broader problem. These algorithms play an increasing role in our daily lives but lack any kind of transparency.
It’s hard to argue that YouTube doesn’t play a role in radicalization. This was first pointed out by technology sociologist Zeynep Tufekci, who illustrated how recommended videos gradually drive users towards more extreme content. In Tufekci’s words, videos about jogging lead to videos about running ultramarathons, videos about vaccines lead to conspiracy theories, and videos about politics lead to “Holocaust denials and other disturbing content”.
This has also been written about in detail by ex-YouTube employee Guillaume Chaslot who worked on the site’s recommendation algorithm. Since leaving the company, Chaslot has continued trying to make those recommendations more transparent. He says YouTube recommendations are biased towards conspiracy theories and factually inaccurate videos, which nevertheless get people to spend more time on the site.
In fact, maximising watchtime is the whole point of YouTube’s algorithms, and this encourages video creators to fight for attention in any way possible. The company’s sheer lack of transparency about exactly how this works makes it nearly impossible to fight radicalization on the site. After all, without transparency, it is hard to know what can be changed to improve the situation.
But YouTube isn’t unusual in this respect. A lack of transparency about how algorithms work is usually the case whenever they are used in large systems, whether by private companies or public bodies. As well as deciding what video to show you next, machine learning algorithms are now used to place children in schools, decide on prison sentences, determine credit scores and insurance rates, as well as the fate of immigrants, job candidates and university applicants. And usually we don’t understand how these systems make their decisions.
Researchers have found creative ways of showing the impact of these algorithms on society, whether by examining the rise of the reactionary right or the spread of conspiracy theories on YouTube, or by showing how search engines reflect the racist biases of the people who create them.
Machine-learning systems are usually big, complex, and opaque. Fittingly, they are often described as black boxes, where information goes in, and information or actions come out, but no one can see what happens in between. This means that, as we do not know exactly how algorithms like the YouTube recommendation system operate, trying to work out how the site works would be like trying to understand a car without opening the bonnet.
In turn, this means that trying to write laws to regulate what algorithms should or shouldn’t do becomes a blind process or trial and error. This is what is happening with YouTube and with so many other machine learning algorithms. We are trying to have a say in their outcomes, without a real understanding of how they really work. We need to open up these patented technologies, or at least make them transparent enough that we can regulate them.
Explanations and testing
One way to do this would be for algorithms to provide counterfactual explanations along with their decisions. This means working out the minimum conditions needed for the algorithm to make a different decision, without describing its full logic. For instance, an algorithm making decisions about bank loans might produce an output that says that “if you were over 18 and had no prior debt, you would have your bank loan accepted”. But this might be difficult to do with YouTube and other sites that use recommendation algorithms, as in theory any video on the platform could be recommended at any point.
Another powerful tool is algorithm testing and auditing, which has been particularly useful in diagnosing biased algorithms. In a recent case, a professional resume-screening company discovered that its algorithm was prioritizing two factors as best predictors of job performance: whether the candidate’s name was Jared, and if they played lacrosse in high school. This is what happens when the machine goes unsupervised.
In this case, the resume-screening algorithm had noticed white men had a higher chance of being hired, and had found correlating proxy characteristics (such as being named Jared or playing lacrosse) present in the candidates being hired. With YouTube, algorithm auditing could help understand what kinds of videos are prioritized for recommendation – and perhaps help settle the debate about whether YouTube recommendations contribute to radicalization or not.
Introducing counterfactual explanations or using algorithm auditing is a difficult, costly process. But it’s important, because the alternative is worse. If algorithms go unchecked and unregulated, we could see a gradual creep of conspiracy theorists and extremists into our media, and our attention controlled by whoever can produce the most profitable content.
This article is republished from The Conversation by Chico Q. Camargo, Postdoctoral Researcher in Data Science, University of Oxford under a Creative Commons license. Read the original article.
Get the TNW newsletter
Get the most important tech news in your inbox each week.