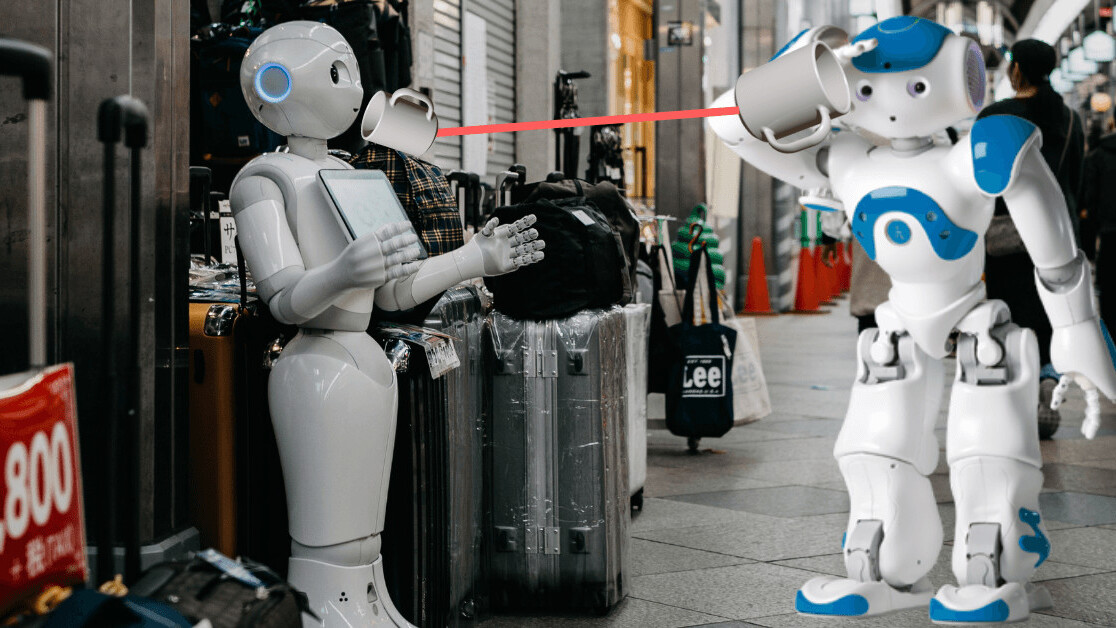
“I’m sorry, I didn’t quite get that.” Anyone who has ever tried to have a deeper conversation with a virtual assistant like Siri knows how frustrating it can be.
That’s despite the fact that AI systems like it are increasingly pushing into our lives, with new success stories on an almost daily basis. Not only do AIs now help radiologists detect tumours, they can act as cat repellent, and even detect signals of potential alien technology from space.
But when it comes to fundamental human abilities, like having a good chat, AI falls short. It simply cannot provide the humor, warmth and the ability to build coherent and personal rapport that is crucial in human conversations. But why is that and will it ever get there?
Chatbots have actually come a long way since their early beginnings, with MIT’s Eliza in the 1960s. Eliza was based on a set of carefully crafted rules that would give the impression of being an active listener and simulating a session with a psychotherapist.
Systems like Eliza were good at giving a sophisticated first impression but were easily found out after a few conversational turns. Such systems were built on efforts to collate as much world knowledge as possible, and then formalize it into concepts and how those relate to each other.
Concepts and relations were further built into grammar and lexicons that would help analyze and generate natural language from intermediate logical representations. For example, world knowledge may contain facts such as “chocolate is edible” and “rock is not edible”.
Learning from data
Today’s conversational AI systems are different in that they target open domain conversation – there is no limit to the number of topics, questions, or instructions a human can ask.
This is mainly achieved by completely avoiding any type of intermediate representation or explicit knowledge engineering. In other words, the success of current conversational AI is based on the premise that it knows and understands nothing of the world.
The basic deep learning model underlying most current work in natural language processing is called a recurrent neural network, whereby a model predicts an output sequence of words based on an input sequence of words by means of a probability function that can be deduced from data.
Given the user input “How are you?” the model can determine that a statistically frequent response is “I am fine.”
The power of these models lies partially in its simplicity – by avoiding intermediate representations, more data will typically lead to better models and better outputs.
Learning for an AI is very similar to how we learn: digest a very large training data set and compare it with known but unseen data (test set). Based on how well the AI performs against the test set, the AI’s predictive model is then adjusted to get better results before the test is repeated.
But how do you determine how good it is? You can look at the grammar of utterances, how “human like” they sound, or the coherence of a contribution in a sequence of conversational turns.
The quality of outputs can also be determined as a subjective assessment of how closely they meet expectations. MIT’s DeepDrumpf is a good example – an AI system trained using data from Donald Trump’s Twitter account and which uncannily sounds just like him, commenting on a number of topics such as healthcare, women, or immigration.
However, problems start when models receive “wrong” inputs. Microsoft’s Tay was an attempt to build a conversational AI that would gradually “improve” and become more human-like by having conversations on Twitter.
Tay infamously turned from a philanthropist into a political bully with an incoherent and extremist world view within 24 hours of deployment. It was soon taken offline.
As machines learn from us, they also take on our flaws – our ideologies, moods and political views. But unlike us, they don’t learn to control or evaluate them – they only map an input sequence to an output sequence, without any filter or moral compass.
This has, however, also been portrayed as an advantage. Some argue that the recent successes of IBM’s Project debater, an AI that can build “compelling evidence-based arguments” about any given topic, is down to its lack of bias and emotional influence. To do this, it looks up data in a large collection of documents and pulls out information to express the opposite view to the person it is debating with.
Next steps
But even if more data can help AI learn to say more relevant things, will it ever really sound human? Emotions are essential in human conversation. Recognizing sadness or happiness in another person’s voice or even text message is incredibly important when tailoring our own response or making a judgement about a situation. We typically have to read between the lines.
Conversational AIs are essentially psychopaths, with no feelings or empathy. This becomes painfully clear when we are screaming our customer number down the phone for the seventh time, in the hope that the system will recognize our agony and put us through to a human customer service representative.
Similarly, conversational AIs usually don’t understand humor or sarcasm, which most of us consider crucial to a good chat. Although individual programs designed to teach AI to spot sarcastic comments among a series of sentences have had some success, nobody has managed to integrate this skill into an actual conversational AI yet.
Clearly the next step for conversational AIs is integrating this and other such “human” functions. Unfortunately, we don’t yet have the techniques available to successfully do this. And even if we did, the issue remains that the more we try to build into a system, the more processing power it will require. So it may be some time before we have the types of computers available that will make this possible.
AI systems clearly still lack a deeper understanding of the meaning of words, the political views they represent, the emotions conveyed and the potential impact of words.
This puts them a long time away from actually sounding human. And it may be even longer before they become social companions that truly understand us and can have a conversation in the human sense of the word.
This article is republished from The Conversation by Nina Dethlefs, Lecturer of Computational Science, University of Hull; Annika Schoene, PhD Candidate, Computer Science, University of Hull, and David Benoit, Senior Lecturer in Molecular Physics and Astrochemistry, University of Hull. Read the original article.
Get the TNW newsletter
Get the most important tech news in your inbox each week.