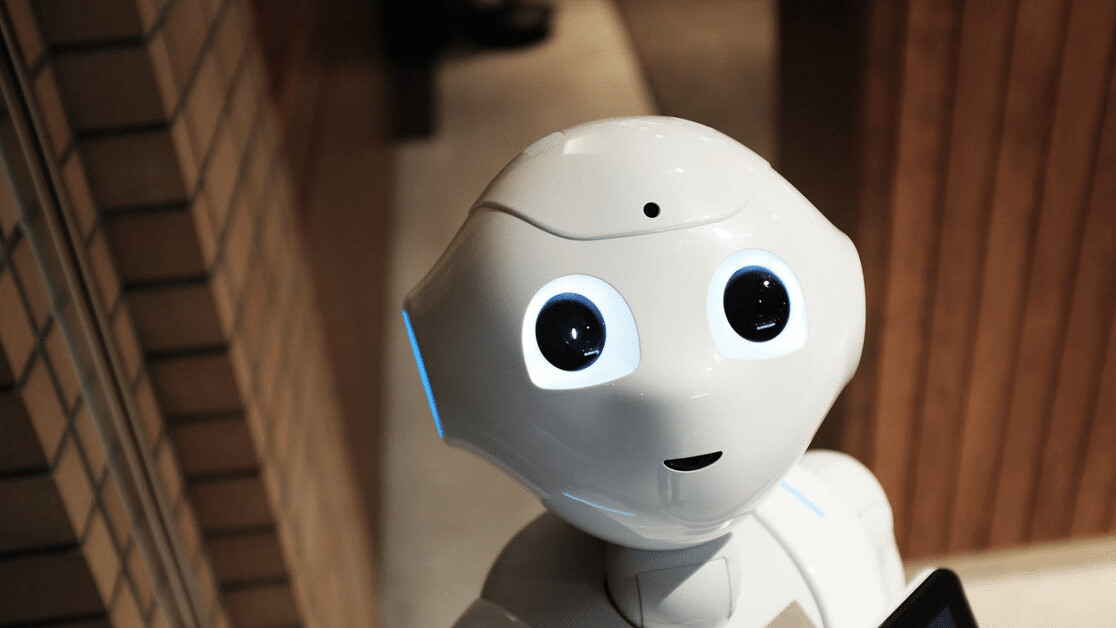
There are many parallels between self-driving car and artificial intelligence. They are goals that, the closer we get to them, the more difficult they seem. In 1956, scientists John McCarthy and Marvin Minsky proposed that a “2-month, 10-man study of artificial intelligence” would unlock the secrets of the human mind. We would soon be able to precisely describe every aspect of learning or any other feature of intelligence in a way “that a machine can be made to simulate it.”
More than six decades later, our AI systems are still struggling to replicate the basic cognitive functions of a human child, let alone the general problem-solving capabilities of an adult mind. We never managed to create human-level AI—instead, we moved the goal posts and split AI into narrow, general and super artificial intelligence.
We can observe a similar trend in self-driving cars. A couple of years ago, the media was abuzz with the promise of self-driving cars. By many accounts, 2019 was supposed to be the year we would become used to seeing cars with empty driver’s seats on roads.
But we’re well into 2019, and self-driving cars remain an elusive target. They are still test projects in limited, fenced areas. They still make stupid mistakes. And the same people who were heralding the coming of self-driving cars are now acknowledging that it will be years before we can remove humans from behind the steering wheel.
(Cashier-less stores might be in for a similar reality check.)
To many, general AI and self-driving cars are impossible goals, failed projects that are only a waste of time and money. And in a sense, they might be right. We might never reach them.
But a closer look shows us that we still need people who conceive and aim for these impossible goals.
Will we ever create general AI?
General AI still has its proponents. One of the most relevant is OpenAI, the research lab founded by Elon Musk and Sam Altman that has been behind several remarkable projects.
OpenAI’s mission statement reads: “OpenAI’s mission is to ensure that artificial general intelligence (AGI)—by which we mean highly autonomous systems that outperform humans at most economically valuable work—benefits all of humanity. We will attempt to directly build safe and beneficial AGI, but will also consider our mission fulfilled if our work aids others to achieve this outcome.”
Not everyone, however, agree with that assessment, and the our current AI technologies work very differently from the human mind.
Many of the world’s top AI scientists believe that human-level AI is a useless goal, and we already have plenty of useful narrow AI applications and technologies we should focus on instead of trying to replicate human intelligence.
One of my favorite quotes in this regard is from Peter Norvig, AI pioneer and director of research at Google. “We know how to build real intelligence—my wife and I did it twice, although she did a lot more of the work,” Norvig said in an interview with Forbes. “We don’t need to duplicate humans. That’s why I focus on having tools to help us rather than duplicate what we already know how to do. We want humans and machines to partner and do something that they cannot do on their own.”
But the real question should be, should we try to build human-level AI at all, even if it proves to be impossible? To that, I would say yes. AI’s history shows that while we are still very far from replicating the artificial version of the human brain, the process has taught us a lot about the laws of intelligence and reasoning, and has given us many other powerful tools that have augmented our abilities.
Interestingly, this brings me to another quote from Norvig. In his famous book Artificial Intelligence: A Modern Approach, Norvig says, “[W]ork in AI has pioneered many ideas that have made their way back to mainstream computer science, including time sharing, interactive interpreters, personal computers with windows and mice, rapid development environments, the linked list data type, automatic storage management, and key concepts of symbolic, functional, declarative, and object-oriented programming.”
All of these important tools would not have existed (or at least it would have taken much longer to invent them) had it not been for people who had been chasing the wild dream of thinking machines and general AI.
Likewise, when you look at all the advanced AI technologies we have today, they are strongly related to the goal of recreating the human mind. Neural networks are named and designed after the physical structure of the human brain; machine learning is inspired by the way humans learn through experience and repetition; expert systems tried to encompass the knowledge and logic of different domains in the ways that humans work.
These and many other AI techniques are not an exact recreation of their human counterparts, but they have helped solve very important problems, such as predicting cancer, detecting objects in pictures, translating text between different languages, and converting speech to text.
The quest for autonomous driving has made our roads safer
Again, we can see parallel developments in the self-driving car industry. For the moment, driverless cars are limited to test projects in areas where roads are not too busy, the weather and lighting conditions are relatively stable, and there’s a detailed digital map of the area. And wherever you see driverless cars on roads, there’s a human driver behind the steering wheel, ready to jump in if the car makes a mistake, such as accelerating toward a lane separator.
This is far from the original vision of having cars that could drive on any road, and as safely (or even safer) than human drivers.
There’s no telling when we’re going to have level-5 self-driving cars in cities, the type of driverless car that has no steering wheel and needs no human intervention at all.
But in its short history, the self-driving car industry has already delivered many important achievements. Even though we don’t have driverless cars that can operate in the open and unpredictable environments that humans are used to, we have developed plenty of useful technologies such as auto-parking, lane assist, blind-spot warnings and drowsiness detection.
These are incremental improvements, but each of them are making driving a little bit safer and will help save thousands of lives every year.
The current technology is also ready to be deployed in environments that are more controlled, such as factories and industrial facilities. An example is autonomous forklifts, which are developing into a promising market, especially since the supply of skilled operators is lagging behind the demand. There are also use cases for low-speed shuttles in low-traffic neighborhoods or inside closed complexes.
But the race to create autonomous vehicles has also brought advances in other fields, such as lidars, computer vision, sensor technology and digital mapping. These are all infrastructural technologies that have many other uses in domains that are not necessarily related to vehicles.
We still need to shoot for the stars
The history of mankind is marked with wild dreams and reality checks. The people who wanted to imitate bird flight never created flapping wings, but helped us discover the laws of flight and invent airplanes. A German scientist who was exploring telepathy never achieved his goal, but instead invented the electroencephalogram (EEG), one of the most important tools in studying the brain and diagnosing diseases related to the nervous system.
Likewise, we still need people who try to invent the impossible, whether it’s artificial general intelligence, fully autonomous vehicles or cashier-less stores. We might never reach those dreams, but what we achieve in the process is no less rewarding.
This story is republished from TechTalks, the blog that explores how technology is solving problems… and creating new ones. Like them on Facebook here and follow them on Twitter.
Get the TNW newsletter
Get the most important tech news in your inbox each week.