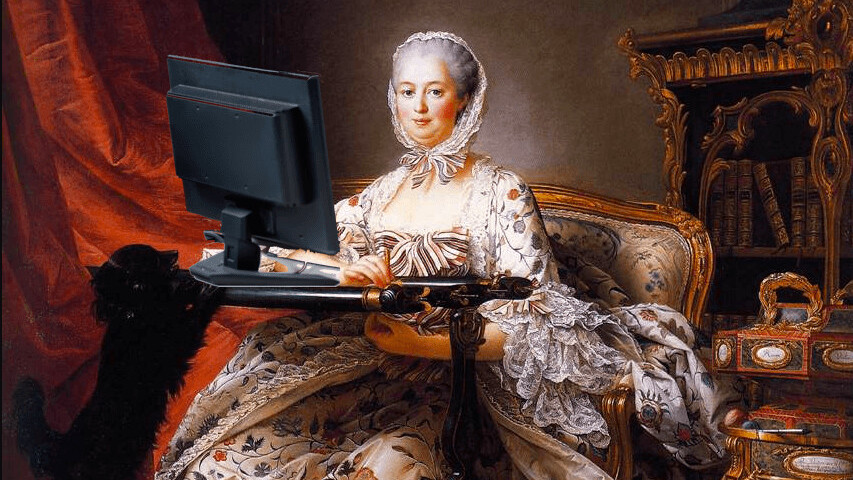
Fun fact: Google Translate seems to think there are no women coders. See for yourself:
Reversing the translation, however, means going from a neutral form to a gendered form. In other words: Google Translate needs to decide on a pronoun itself. And that’s when gender bias kicks in. The algorithm is trained to give the answer it deems most likely (more about that later), so picks “he” over “she.” Try the same thing with “he’s a babysitter” or “he’s a nurse” and you will get the opposite result.
It’s just one of the many examples of gender bias that have come to light over the past few years. An online ad for a high-paying executive job was shown to men 1,816 times and just 311 times to women. And if you do a Google Image search for “successful person” the list of search results will mainly include men. White men, that is, because the problem isn’t limited to gender — racial and religious biases exist as well.
Machines aren’t biased, of course — humans are. So how does bias sneak into our software? The trouble starts with the data that is used to train machine learning algorithms. In case of image recognition, the algorithm learns by processing an enormous amount of pictures. Google, like many other tech companies, made use of face database LFW (Faces in the Wild) to measure algorithmic performance. When LFW was analyzed in 2014, the results revealed 83 percent of the people in the pictures were white and nearly 78 percent were male. In other words: The gap exists because the machine is mostly trained with photos of white men.
A similar thing happens with natural language processing (NLP). Just like how image recognition algorithms learn by looking at large amounts of pictures, machine reading algorithms are trained by processing large amounts of text. If the algorithm is fed with thousands of stories about male programmers but only reads five hundred stories about female programmers, it will create a stronger association between the words “man” and “programmer” than the female equivalent.
Man is to Computer Programmer as Woman is to Homemaker
Researchers from Boston University, who trained an algorithm with a bunch of Google News stories, were able to identify this bias. They let the algorithm process an analogy: “man is to computer programmer as woman is to X.” Natural language processing relies on word embedding to make sense of words: Think of word embedding as adding context, by creating relationships between words based on how and how often they’re used together.
So when you ask “man is to king as woman is to X,” a properly trained algorithm will spit out the answer “queen.” Asking the algorithm to find the female counterpart of male computer programmers, however, resulted in the answer “homemaker.”
To Alejandra Leon Moreno, who’s the Lead Digital Architect at Philips, gender bias is an issue that should be taken seriously. “When we’re looking at NLP, for instance, Philips is currently developing a system to do sentiment analysis based on customer reviews and customer calls. When bias sneaks into voice recognition technology, the consequence is that female voices, as well as foreign accents, are harder to understand for the software.”
If this means Philips must make do with less accurate feedback about their lady shavers and blow dryers, surely, the marketing department won’t be happy — but it wouldn’t be an issue of public concern. But because the company’s main focus is on health care, using software that is biased — whether this it has to do with gender, age or ethnicity — just isn’t acceptable. “That would lead to a future where some people receive better and faster healthcare than others,” says Leon Moreno. “And that’s a scenario we need to avoid at any cost.”
In an attempt to actively fight unsavory bias in technology, some AI experts proposed we need to implement some sort of AI watchdog; a third party that can investigate claims by people who feel discriminated against by technology. Although an independent initiative, such an organization already exists. The Algorithmic Justice League, founded by Joy Buolamwini, lets users report cases of “coded gaze,” as she calls the phenomenon.
Another, more structural solution, would be to have more women working in tech — battling bias in the algorithms by reducing bias in the workplace. Women shouldn’t just to learn how to code but need to be elevated into leadership positions more frequently as well. And we need to do it fast, says AI researcher Fei-Fei Li in this interview with Backchannel: “If we don’t get women and people of color at the table — real technologists doing the real work — we will bias systems. Trying to reverse that a decade or two from now will be so much more difficult, if not close to impossible.”
So how to go about this? Organize yet another ‘women in tech’ conference? Host the umpteenth TED talk on the subject? Although Leon Moreno supports these initiatives, she believes change needs to happen on a more basic level — during school. “Girls should learn at a young age that coding is nothing to be afraid of. It can be fun and creative, and definitely isn’t ‘too hard’ for them to learn.” They could also benefit from having more female role models, she thinks. “I was lucky enough to have great mentors, but they were all men.”
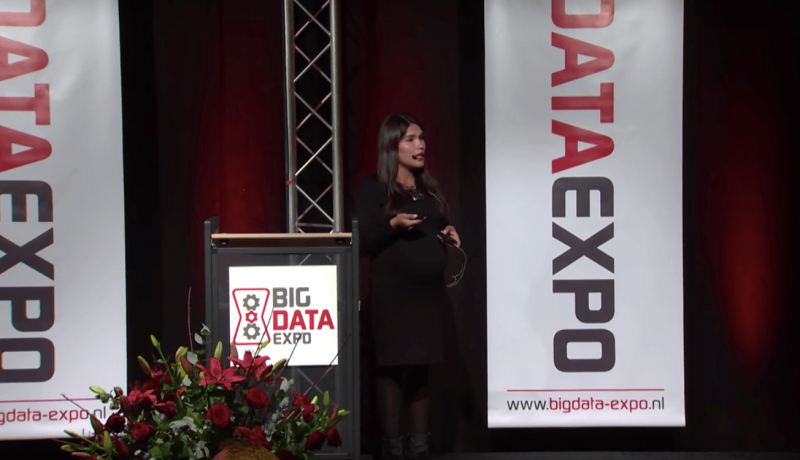
Still, that didn’t stop her from getting where she is today. Leon Moreno grew up in Peru, studied Software Engineering in Australia, and then made a career for herself in Australia and the Netherlands. But she did experience some pushback on the way, she says; things that wouldn’t have happened if she were a man: “During an interview, I was told I was very qualified for the job. It was just that they were looking for someone ‘stronger.’ When I asked them to be more specific, they couldn’t give me a clear answer. In those situations, I know how to read between the lines.”
Working for Philips, she never felt underappreciated. And thanks to its flexible working culture, employees can attain a good work-life balance. Leon Moreno works in Amsterdam and Eindhoven and lives in The Hague. “If you have kids, like me, being able to work remotely can be a real life-saver.”
Although reaching higher levels of inclusion is an important company goal for Philips, right now, Leon Moreno’s team at Philips is still predominantly male. “I understand we can’t reach full equality overnight — but I am hoping for a 50/50 divide one day,” she concludes. “That’s the dream.”
Get the TNW newsletter
Get the most important tech news in your inbox each week.