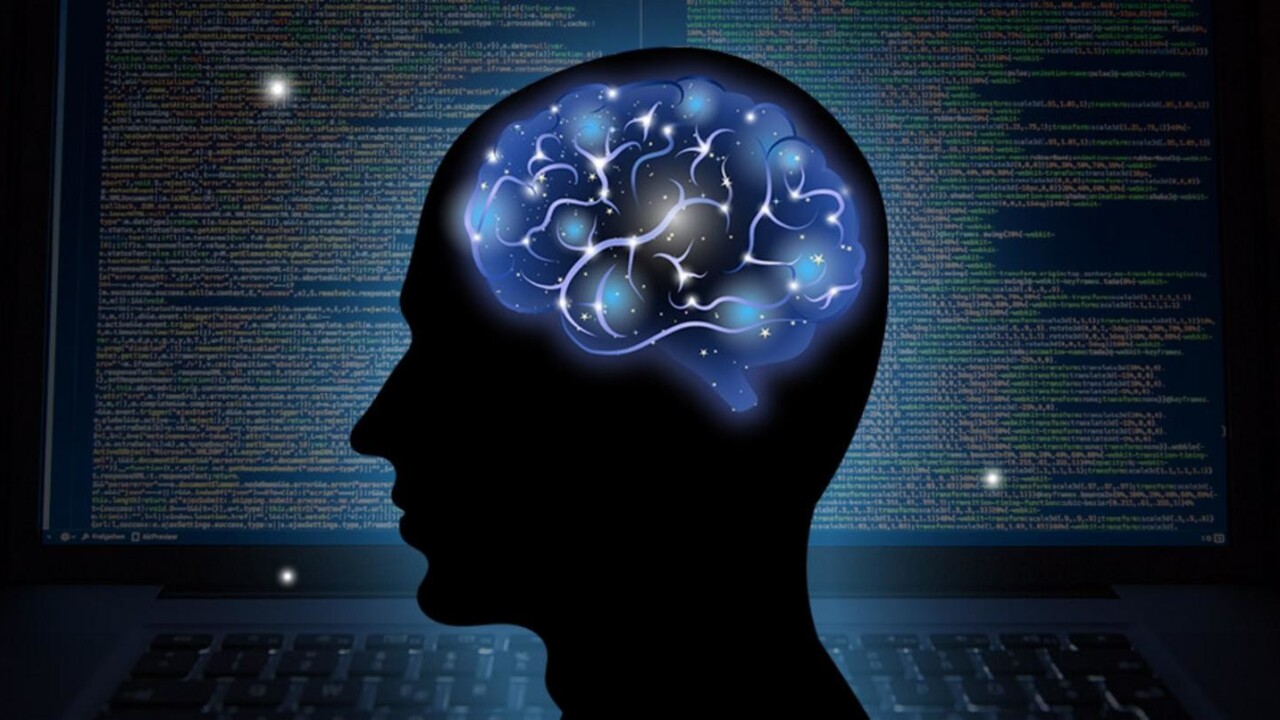
Scientists at Columbia and Lehigh Universities have effectively created a method for error-correcting deep learning networks. With the tool, they’ve been able to reverse-engineer complex AI, thus providing a work-around for the mysterious ‘black box’ problem.
Deep learning AI systems often make decisions inside a black box – meaning humans can’t readily understand why a neural-network chose one solution over another. This exists because machines can perform millions of tests in short amounts of time, come up with a solution, and move on to performing millions more tests to come up with a better solution.
The researchers created DeepXplore, software that exposes flaws in a neural-network by tricking it into making mistakes. Co-developer Suman Jana of Columbia University told EurekAlert:
You can think of our testing process as reverse engineering the learning process to understand its logic
The researchers’ method for solving the black box issue involves throwing a series of problems at the system that it can’t quite deal with. Incremental increases or decreases in lighting, for example, might cause an AI designed to steer a vehicle to be unable to decide which direction to go.
Through clever optimization DeepXplore is able to trigger up to a 100 percent neuron activation within a system – basically activating the entire network to sweep for errors and try to cause problems. It’s a “break everything” approach that quality assurance testers can appreciate.
One limitation: it’s not fool-proof. It can’t self-certify that your AI is error-free, though it is far superior to random testing or “adversarial testing,” according to the teams research.
Trying to figure out how an advanced neural network came to a specific conclusion would be like personally counting an accurate number of apples to “prove” a calculator’s assertion that one million multiplied by two equals two million. When it comes to that kind of thing: AI does it better.
The researchers at Columbia and Lehigh are doing impressive work, and according to co-developer Kexin Pei, of Columbia University:
We plan to keep improving DeepXplore to open the black box and make machine learning systems more reliable and transparent. As more decision-making is turned over to machines, we need to make sure we can test their logic so that outcomes are accurate and fair.
Figuring out how to debug and error-check the most advanced neural networks would go a long way towards the integration of AI into fields like healthcare, transportation, security, and defense.
Isolating and eliminating the biases that cause AI to reach conclusions that either endanger life or discriminate, is one of the biggest challenges facing machine-learning developers.
The AI Now Institute recently cautioned important government facilities against the use of black box AI systems until such a time as we can figure them out.
Thanks to DeepXplore that time is arriving faster than anyone could have predicted.
We reached out to the folks behind DeepXplore to find out more, and will update as necessary.
Get the TNW newsletter
Get the most important tech news in your inbox each week.