In biomedical research alone, an estimated $2 billion may have evaporated last year over papers that were retracted due to doctored images. Now, a growing body of researchers are turning to AI to catch crooked scientists in the act, and hopefully solve this decade-old issue.
On September 10, a controversial paper was published in the Scientific Reports journal claiming that a homeopathic treatment formulated from the poison ivy plant could ease pain in rats. While the uproar was initially due to the fierce debate surrounding the efficacy of homeopathy, it intensified when it turned out that the paper had doctored images.
This was not the first case a research paper on a controversial topic had turned out to have falsified images. Last month, we reported the case of an anti-vaccination paper being retracted from Journal of Inorganic Biochemistry because it had fake images.
Next findings: 2 duplicated panels in the same figure, representing two distinct anatomical sites. This looks like an error. pic.twitter.com/tR4q9cFWvG
— Elisabeth Bik (@MicrobiomDigest) August 28, 2017
Image falsification in academic papers can be anything from that of microscopic view of materials, cells, tissues, or gel bands representing the concentration of chemicals, or even the images of graphs based on research data.
From the same publisher.
Just live tweeting what I find when I am scanning scientific papers for these types of duplications. pic.twitter.com/lSck6av1Gd— Elisabeth Bik (@MicrobiomDigest) August 27, 2017
How bad can it be, right?
The scourge of image forgery in academic research papers is quite prevalent.
Two weeks back, a database of 18,000 retracted research papers (dating as far as the 1970s) was launched, making it the largest of its kind. Of these, 317 papers were retracted for image falsification – which is about 1.7 percent of the overall papers.
A searchable database of more than 18,000 retracted journal articles was released to the public last week. A @ScienceMagazine analysis shows that a recent increase in retractions reflects not an epidemic of fraud, but a community trying to police itself. https://t.co/gXheRCfmHL pic.twitter.com/2Fy6tAgcIY
— AAAS (@aaas) October 31, 2018
An earlier study of 20,000 research papers by Stanford microbiologist Elisabeth Bik also revealed that about 2 percent of all the papers were worth retracting for image falsification.
Lets put that in perspective. Arjun Raj – associate professor of Bioengineering in University of Pennsylvania – noted that the science behind an average biomedical research paper cost around $300,000 – $500,000. And Lancet reported that US researchers published nearly 152,000 papers in 2012 – the year Raj came up with the estimate.
So even if we take the lower limit, the cost of all the biomedical science papers published by US researchers in 2012 would have been close to $50 billion.
If 2 percent of those papers needed retraction for image forgery, the US may have wasted close to $1 billion in 2012 because of the Machiavellian means of its researchers. That’s a figure greater than what the country loses every year to the Nigerian prince scam.
As the global scientific output doubles every nine years, the negative margin might’ve gotten even bigger since 2012.
Has image tampering always been this bad?
Some researchers believe that the problem has gotten worse over the years.
Mike Rossner is the founder of the company Image Data Integrity (IDI) that helps institutions in their internal investigations on suspected image manipulation, and assists journals that do not have in-house image detectives. Speaking to TNW he said:
Data from the United States Office of Research Integrity (ORI) indicates that the percentage of cases that they handled involving image manipulation increased after the release of Photoshop (in 1990 for Mac and in 1996 for PC).
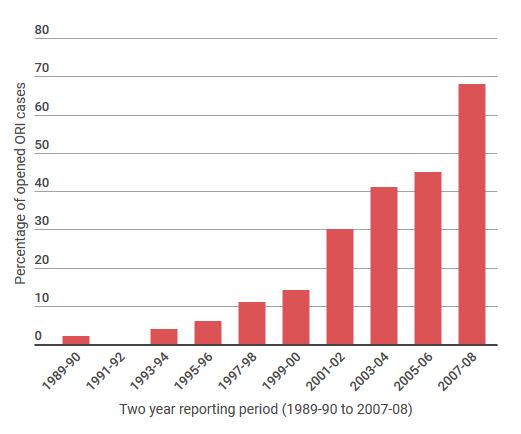
The surge in use of fraudulent images in the early 90s may have been due to journals still following a paper-based workflow when researchers started using computers to draft manuscripts.
Fighting software with software
However, the fight against image doctoring in academia is evolving now with the help of image forensics software customized for academic research.
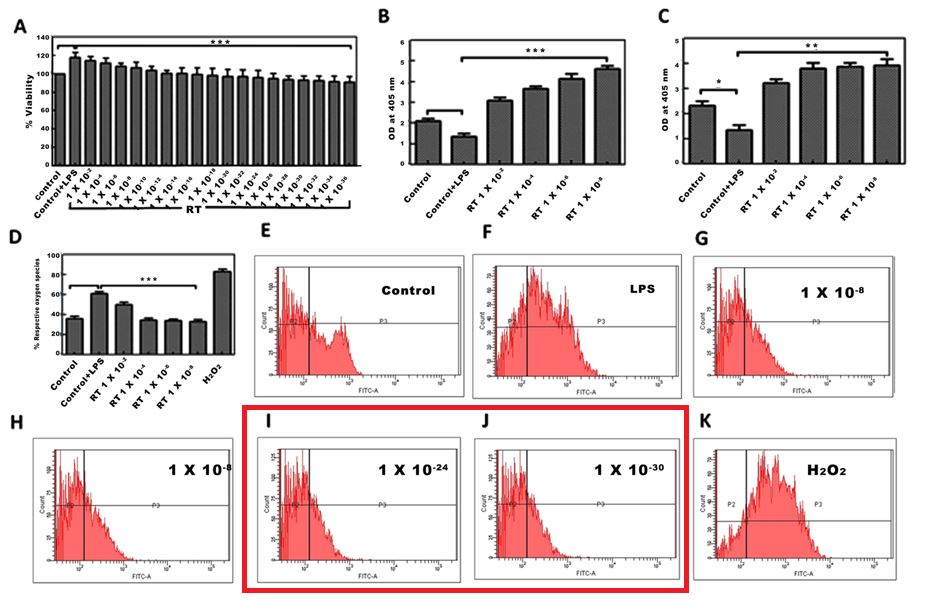
For instance, the homeopathy paper mentioned earlier was flagged thanks to a software used by biologist Enrico Bucci who also runs a research integrity solutions company – Resis. The company’s software detected that two different experiments with wildly different concentrations of the proposed remedy had eerily identical graphs.
In another case reported by Nature, a team led by Daniel Acuna – a machine-learning researcher at Syracuse University in New York – used an algorithm they developed to crunch through hundreds of thousands of biomedical papers, searching for duplicate images. The team’s findings published on the preprint bioRxiv reported that 9 percent of the searched images looked suspicious.
Acuna’s application scans images for anomalies like rotated parts, changed colors, and inappropriate reuse. Using these signals, the software automatically reports if a certain tampering was intentional and, therefore, more likely to be fraudulent.
And Elisabeth Bik, who as mentioned earlier, uses the freely available photo forensics software 29a.ch to expose some papers with fake images on her Twitter account.
If you want to get a glimpse of how these forensics software help spot tampered images, you can check out this website by Humboldt-Elsevier Advanced Data and Text Centre.
Will the process ever be truly autonomous?
However, even if a software-based approach has been widely spoken about for almost a decade now, companies using such applications rarely publish their results with software. Even press releases of busts solely using such applications are rare, leading one to wonder if the process of detecting fake images in research papers still needs a human eye.
Rossner also believes that a software-based approach needs to be backed by human oversight. Speaking to TNW he said:
The development of software to detect image manipulation has the potential to increase the number of journals that screen images. It is important to note, however, that the use of software does not eliminate the need for human intervention. The output from the software will have to be evaluated by a human.
One area were the development of software has the potential to make a large impact is the detection of image duplication against a large database of articles – like the Turnitin plagiarism software does for text. Such large scale comparisons are not possible to do using visual inspection techniques.
What is the future of this industry like?
This is where Acuna’s team seeks to cash in with its machine learning expertise. He aims to scale up the algorithm to analyze large databases.
Acuna believes that humans in the verification system can help reduce false positives, but scaling up with software is essential to make it faster. He noted in his interview with TNW:
When you are comparing one image against a set of other images that’s where the problem comes. You have to remember each image, or compare one image very carefully against all the other ones you have. So if you are doing it manually, how can, say, you check an image in a paper with all the images in the papers written by the same author?
So my work is to find out if a certain image of a cell in paper has already been used in a database of scientific papers.
The future of this growing industry may take two forms according to Acuna. In one scenario, companies may provide customized applications to journal editors who can then use these to make their own analysis of incoming papers. “This could be similar to how anti-plagiarism software work,” he said.
Another way, he added, is for image integrity analysis companies to allot their own human and computer resources for journals to conduct image integrity checks.
Bucci, whose company debunked the homeopathy paper, also believes that this could be the course of evolution of the industry. He mentioned in his email to TNW:
There are already some signals that this might happen soon, e.g. the collaboration between Elsevier and the Humboldt University, as well as the hiring of image analysts by isolated (but powerful) scientific journals.
In both cases, however, I think there will be room for smaller business and single experts in the field because there is a need from public research institutions to both face potential allegations against their own researchers and to prevent them by screening manuscripts to be submitted.
As automated image analysis software evolves into an enterprise, fraudulent researchers may find it harder to get away with their schemes. Then perhaps, there may come yet another sophisticated tool that they would misuse to fake images, keeping this cat-mouse game alive.
Get the TNW newsletter
Get the most important tech news in your inbox each week.