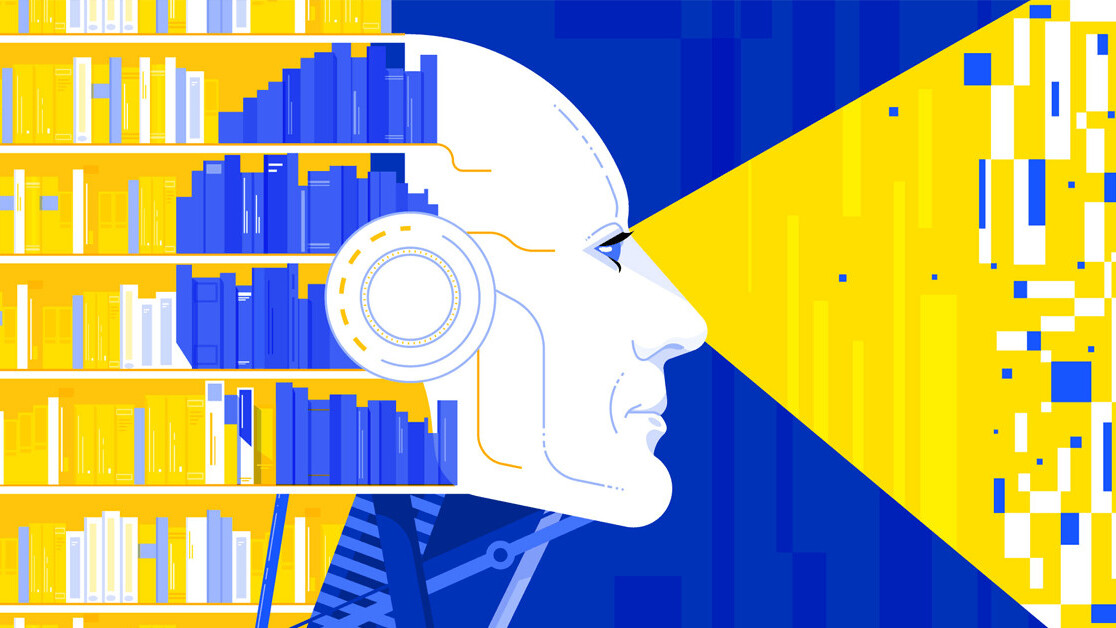
The die is very much cast when it comes to the growth of machine learning. With the expansion of tech companies like Google, Amazon and Uber, artificial intelligence-based research and products is a growth industry that’s only just getting started.
Research from the International Data Commission forecasts that spending on AI and ML will rise from $12 billion in 2017 to $57.6 billion by 2021. The skyrocketing research funding into these fields is mirrored by the resulting patents being filed, with machine learning patents increasing by 34% between 2013 and 2017.
As the number of commercial products that are built on these architectures increases, so will the demand for engineers and researchers to work on them. This translates into handsome salaries at some of the world’s leading tech companies.
At the time of writing, the average salary for a machine learning engineer in the United States was listed on some employment sites at around $138,000. There is concern from some in the field of academia that high starting salaries and assorted perks could result in a brain drain from universities, with candidates who would previously have continued their research at established institutions being poached into careers.
In a Guardian article from November 2017, Maja Pantic, professor of affective and behavioural computing at Imperial in London, noted that one of her students dropped his PhD in its final year to go and work at Apple for a six-figure salary. “It’s five times the salary I can offer,” Paja said. “It’s unbelievable. We cannot compete. The creme de la creme of academia has been bought and that is worrying.”
Just how prevalent this is, though, is a matter of some debate. To get a better idea of the state of the machine learning field in relation to its industrial demand, Binary District Journal spoke to Chelsea Finn, PhD candidate at Berkeley. A respected machine learning academic, she will join the Stanford faculty in 2019, and has also worked at Google Brain.
Upsides and Downsides
Chelsea believes demand is outstripping supply at the moment. A particular skill set in demand is the intersection of machine learning with real robot hardware – with the ability to run experiments on physical robots.
“A lot of people are a bit afraid of working with real systems because they can take a fair amount of time to get set up,” Chelsea says, “and these might be people who prefer much faster prototyping in simulated domains.”
However, Chelsea believes that the matter of researchers forgoing the traditional extended academic pathways in favour of joining tech companies is an area that is not necessarily black and white.
“I think there are benefits and downsides to what is currently happening,” she says. “I think that the benefits are that academics can build better connections with industry labs and this can mean that there is better access for some academics to industry-level compute and industry-level data resources, but I also think that there are certainly downsides.
“Many schools have faculty that are spending more time in industry and less time in academia. This leads to fewer faculty that are available to teach classes. It also leads to faculty potentially having more conflicts of interest and they’re not necessarily an independent academic entity that’s trying to conduct research independently of any companies’ interests, and now have an affiliation with a company.”
A Rising Tide Raises All Ships
That being said, Chelsea is quick to note that she does see the benefit of working with companies on a part-time basis. There are certain situations in machine learning research where, to effectively make progress, you need the large-scale computing and robotic hardware that are only really available in larger tech companies’ R&D labs.
Having a role in tackling those projects is an appealing scenario. These are quite specialised problems though, and Chelsea points out that in her experience, the level of compute in the university labs that she has worked in (and will work in at Stamford) is more than up to the task.
Chelsea is not just furthering her own research, but also looking for ways to secure the continued growth of machine learning.
“As a member of the machine learning community and the research community, it’s really easy to notice that it’s not a diverse community in terms of a number of different metrics – in terms of race, gender and economic background,” Chelsea says. “I hope to make a positive change and a positive impact on the diversity of the community through various outreach efforts.”
In 2017, Chelsea co-organised the first BAIR Camp – a week-long program in partnership with AI for All that invited underprivileged high school students from the Bay Area to learn more about the fields of machine learning and artificial intelligence. She believes that getting students not only interested, but also directly involved in the fields of machine learning and artificial intelligence, is an important step in securing the future of these fields of R&D.
“The goal is to broaden the diversity of the field, but it’s not just that. It’s so that the technologies that are developed are beneficial not just for the people in the field but for the broader demographics of the world,” says Chelsea.
An Issue of Demand and Supply
Clearly, the scope for and demand for machine learning expertise is only going to continue increasing. Speaking from a position of academia and with experience at the company level, Chelsea Finn points out that the issue with machine learning scalability isn’t a simple case of academics being poached by the allure of the private sector. The issue is that, as an area of research and resulting careers, machine learning has to reach a wider set of demographics who are then able to participate in it.
Because of the close links between academic institutions and the major tech firms, we are increasingly seeing examples of educational resources being offered by companies. Google has developed an education platform on the fields of AI and machine learning, allowing universal access to scholarly material and hands-on projects.
These resources form an important supplement to burgeoning AI projects and startups. Clearly, it is short-sighted to see tech giants like Google as lumbering behemoths, hoovering up the resources and expertise of the machine learning fields.
The issue of demand outweighing supply is a natural result of the success and progress of machine learning development. However, greater collaboration between academia and companies, combined with a greater push for earlier and more inclusive education in these fields, is a positive step in ensuring the industry’s continued scalability.
Life Imitating Art
In a slightly dystopian move, depending on your perspective, the UK police force has been dabbling with machine learning. Of particular note is software that can supposedly make predictions regarding an individual’s proclivity for future crime. A report from RUSI found that there is a ‘concerning’ lack of regulatory framework currently in place to halt the progress of such systems.
If this all seems a little too ‘Minority Report’ for your liking, you’re not alone. There have been numerous calls for complete transparency of the programme. RUSI also found that there are significant concerns over the “efficacy and efficiency of different systems, their cost-effectiveness, their impact on individual rights and the extent to which they serve valid policing aims.” Without such transparency, how are we to know if this is a panacea or just phrenology for the Instagram generation.
John Murray is a tech correspondent focusing on machine learning at Binary District , where this article was originally published.
TNW Conference 2019 is coming! Check out our glorious new location, inspiring line-up of speakers and activities, and how to be a part of this annual tech bonanza by clicking here.
Get the TNW newsletter
Get the most important tech news in your inbox each week.