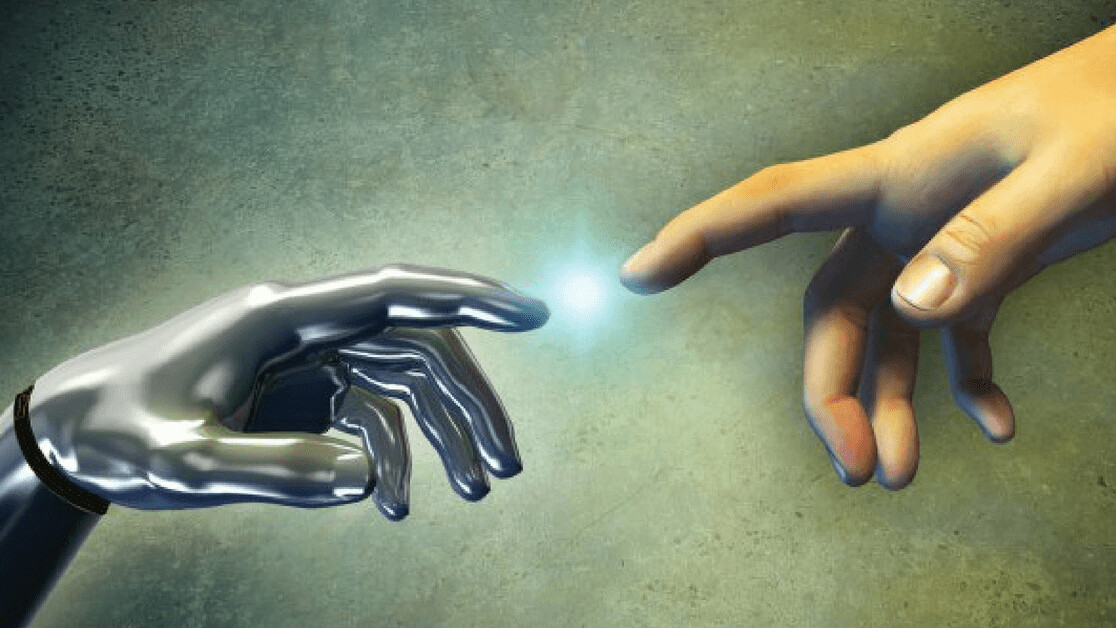
These days, it’s easy to believe arguments that artificial intelligence has become as smart as the human mind—if not smarter. Google released a speaking AI that dupes its conversational partners that it’s human.
DeepMind, a Google subsidiary, created an AI that defeated the world champion at the most complicated board game. More recently, AI proved it can be as accurate as trained doctors in diagnosing eye diseases.
And there are any number of stories that warn about a near future where robots will drive all humans into unemployment.
Everywhere you look, AI is conquering new domains, tasks and skills that were previously thought to be the exclusive domain of human intelligence. But does it mean that AI is better than the human mind?
The answer to that question is: It’s wrong to compare artificial intelligence to the human mind, because they are totally different things, even if their functions overlap at times.
Artificial intelligence is good at processing data, bad at thinking in abstract
Even the most sophisticated AI technology is, at its core, no different from other computer software: bits of data running through circuits at super-fast rates.
AI and its popular branch, machine learning and deep learning, can solve any problem as long as you can turn it into the right data sets.
Take image recognition. If you give a deep neural network, the structure underlying deep learning algorithms, enough labeled images, it can compare their data in very complicated ways and find correlations and patterns that define each type of object.
It then uses that information to label objects in images it hasn’t seen before.
The same process happens in voice recognition. Given enough digital samples of a person’s voice, a neural network can find the common patterns in the person’s voice and determine if future recordings belong to that person.
Everywhere you look, whether it’s a computer vision algorithm doing face recognition or diagnosing cancer, an AI-powered cybersecurity tool ferreting out malicious network traffic, or a complicated AI project playing computer games, the same rules apply.
The techniques change and progress: Deep neural networks enable AI algorithms to analyze data through multiple layers; generative adversarial networks (GAN) enable AI to create new data based on the data set it has trained on; reinforcement learning enables AI to develop its own behavior based on the rules that apply to an environment… But what remains the same is the same basic principle: If you can break down a task into data, AI will be able to learn it.
Take note, however, that designing AI models is a complicated task that few people can accomplish. Deep learning engineers and researchers are some of the most coveted and highly paid experts in the tech industry.
Where AI falls short is thinking in the abstract, applying common sense, or transferring knowledge from one area to another. For instance, Google’s Duplex might be very good at reserving restaurant tables and setting up appointments with your barber, two narrow and very specific tasks.
The AI is even able to mimic natural human behavior, using inflections and intonations as any human speaker would. But as soon as the conversation goes off course, Duplex will be hard-pressed to answer in a coherent way. It will either have to disengage or use the help of a human assistant to continue the conversation in a meaningful way.
There are many proven instances in which AI models fail in spectacular and illogical ways as soon as they’re presented with an example that falls outside of their problem domain or is different from the data they’ve been trained on.
The broader the domain, the more data the AI needs to be able to master it, and there will always be edge cases, scenarios that haven’t been covered by the training data and will cause the AI to fail.
An example is self-driving cars, which are still struggling to become fully autonomous despite having driven tens of millions of kilometers, much more than a human needs to become an expert driver.
Humans are bad at processing data, good at making abstract decisions
Let’s start with the data part. Contrary to computers, humans are terrible at storing and processing information. For instance, you must listen to a song several times before you can memorize it.
But for a computer, memorizing a song is as simple as pressing “Save” in an application or copying the file into its hard drive. Likewise, unmemorizing is hard for humans. Try as you might, you can’t forget bad memories. For a computer, it’s as easy as deleting a file.
When it comes to processing data, humans are obviously inferior to AI. In all the examples iterated above, humans might be able to perform the same tasks as computers. However, in the time that it takes for a human to identify and label an image, an AI algorithm can classify one million images.
The sheer processing speed of computers enable them to outpace humans at any task that involves mathematical calculations and data processing.
However, humans can make abstract decisions based on instinct, common sense and scarce information. A human child learns to handle objects at a very young age. For an AI algorithm, it takes hundreds of years’ worth of training to perform the same task.
For instance, when humans play a video game for the first time in their life, they can quickly transfer their everyday life knowledge into the game’s environment, such as staying away pits, ledges, fire and pointy things (or jumping over them).
They know they must dodge bullets and avoid getting hit by vehicles. For AI, every video game is a new, unknown world it must learn from scratch.
Humans can invent new things, including all the technologies that have ushered in the era of artificial intelligence. AI can only take data, compare it, come up with new combinations and presentations, and predict trends based on how previous sequences.
Humans can feel, imagine, dream. They can be selfless or greedy. They can love and hate, they can lie, they forget, they confuse facts. And all of those emotions can change their decisions in rational or irrational ways.
They’re imperfect and flawed beings made of flesh, which decays with time. But every single one of them is unique in his or her own way and can create things that no one else can.
AI is, at its core, is tiny bursts of electricity running through billions of lifeless circuits.
Let’s stop comparing AI with human intelligence
None of this means that AI is superior to the human brain, or vice versa. They point is, they’re totally different things.
AI is good at repetitive tasks that have clearly defined boundaries and can be represented by data, and bad at broad tasks that require intuition and decision-making based on incomplete information.
In contrast, human intelligence is good for settings where you need common sense and abstract decisions, and bad at tasks that require heavy computations and data processing in real time.
Looking at it from a different perspective, we should think about AI as augmented intelligence. AI and human intelligence complement each other, making up for each other’s shortcomings. Together, they can perform tasks that none of them could have done individually.
For instance, AI is good at perusing huge amounts of network traffic and pointing out to anomalies, but can make mistakes when deciding which ones are the real threats that need investigation.
A human analyst, on the other hand, is not very good at monitoring gigabytes of data going through a company’s network, but they’re adept at relating anomalies to different events and figuring out which ones are the real threats. Together AI and human analysts can fill each other’s gaps.
Now, what about all those articles that claim human labor is going instinct? Well, a lot of it is hype, and the facts prove that the expansion of AI is creating more jobs than it is destroying. But it’s true that it will obviate the need for humans in many tasks, just as every technological breakthrough has done in the past.
But that’s probably because those jobs were never meant for humans. We were spending precious human intelligence and labor on those jobs because we hadn’t developed the technologies to automate them yet.
As AI becomes adept at performing more and more tasks, we as humans will find more time to put our intelligence to real use, at being creative, being social, at arts, sports, literature, poetry and all the things that are valuable because the human element and character that goes into them. And we’ll use our augmented intelligence tools to enhance those creations.
The future will be one where artificial and human intelligence will build together, not apart.
This story is republished from TechTalks, the blog that explores how technology is solving problems… and creating new ones. Like them on Facebook here and follow them down here:
Get the TNW newsletter
Get the most important tech news in your inbox each week.