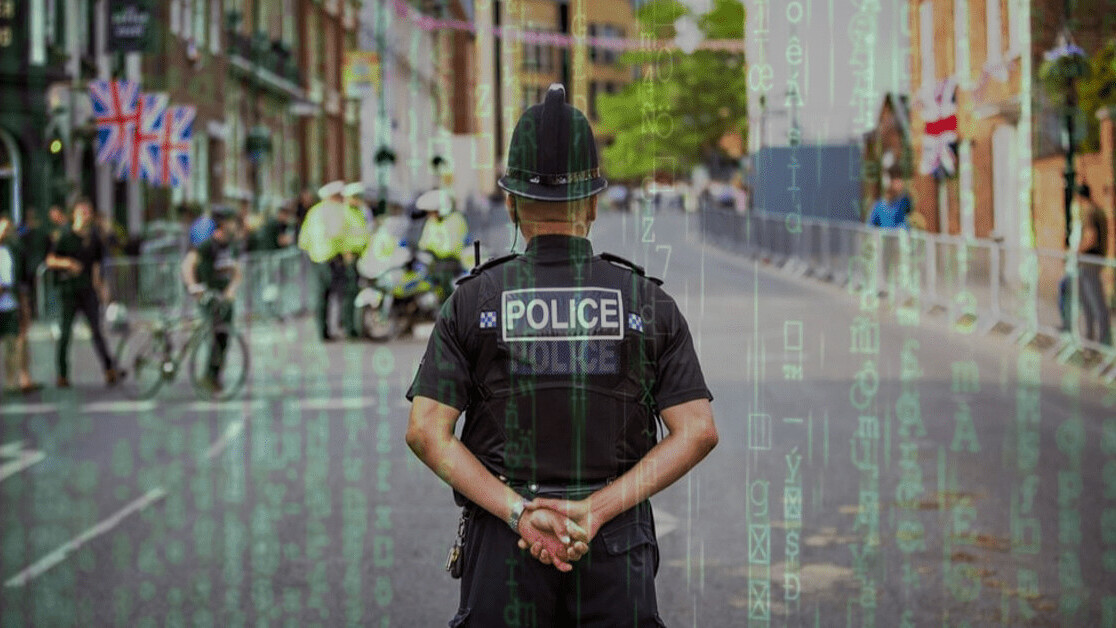
The debate over the police using machine learning is intensifying – it is considered in some quarters as controversial as stop and search.
Stop and search is one of the most contentious areas of how the police interact with the public. It has been heavily criticized for being discriminatory towards black and minority ethnic groups, and for having marginal effects on reducing crime. In the same way, the police use of machine learning algorithms has been condemned by human rights groups who claim such programs encourage racial profiling and discrimination along with threatening privacy and freedom of expression.
Broadly speaking, machine learning uses data to teach computers to make decisions without explicitly instructing them how to do it. Machine learning is used successfully in many industries to create efficiency, prioritize risk and improve decision making.
Although they are at a very early stage, the police in the UK are exploring the benefits of using machine learning methods to prevent and detect crime, and to develop new insights to tackle problems of significant public concern.
It is true that there are potential issues with any use of probabilistic machine learning algorithms in policing. For instance, when using historic data, there are risks that algorithms, when making predictions, will discriminate unfairly towards certain groups of people. But if the police approach the use of this technology in the right way, it should not be as controversial as stop and search and could go a long way towards the police being more effective in preventing and solving crimes.
A modern-day policing challenge
Consider the case of the recent public concern about drill music videos and their unique lyrical content being allegedly used to inspire, incite and glorify serious violence.
Drill music has, over the past few years, spread to major cities in the UK. Social media platforms such as YouTube and Instagram have, at the same time, witnessed a significant increase in drill music videos uploaded online. Many of the videos, which feature male rappers wearing face masks, using violent, provocative and nihilistic language, receive millions of views.
The most senior police officer in the UK, Commissioner Cressida Dick, has publicly criticized drill music videos, stating they are used to glamorize murder and serious violence and escalate tensions between rival street gangs.
Many people disagree with the police blaming of drill music. Supporters of this music genre argue that murder and violence are not a new phenomena, and should not be considered causal to drill artists who rap about the harsh realities of their lived experiences. Some academics are also concerned that the current police approach “is leading to the criminalization of everyday pursuits” and that “young people from poor backgrounds are now becoming categorized as troublemakers through the mere act of making a music video”.
Nevertheless, to the police, this is an important issue: they have a statutory responsibility to protect life and manage risk to the public. As such, detecting harmful online content which, for example, might contain a threat to a person’s life, is both a contemporary operational policing problem, and an intractable technological problem that the police need to be able to solve.
Developing machine learning tools
Police officers manually viewing large amounts of videos to identify and discern harmful and criminal content from legitimate creative expression is hugely inefficient. As such, it should be automated. Yes, there are presently significant technical challenges for machine learning algorithms to understand such unique lyrical content. But this type of problem, for researchers, does fit neatly into the growing machine learning field of natural language processing. This is a field that uses computational techniques to understand human language and speech.
More broadly, there is a lack of research about the social impact of the police using machine learning to prevent and detect crime. So in the meantime, to avoid controversy the police should not rely on opaque off-the-shelf “black box” machine learning models that have not been tested in an operational policing context to automate the analysis of large amounts of data. Black box models are rightly controversial because they do not show their internal logic nor the processes used to make decisions.
A better way forward is for the police to work with experts and build machine learning models specifically designed for policing purposes that make better use of data to tackle problems, such as those inherent with drill music videos. Durham Constabulary, for example, have recently worked with scientists from the University of Cambridge to develop an algorithmic risk assessment tool to help with decisions about future offending when a person is arrested by the police.
In this way, machine learning tools can be established on widely accepted scientific principles – with a level of transparency that can be used to galvanize public support in ways that stop and search has been unable to do.
Concerns over transparency
In a recent report, the British defense and security think tank RUSI raised more specific concerns about the concept of the police using machine learning algorithms to make predictions and support decision making. Notably, it talks about the concept of “algorithmic transparency” and the difficulty for non-experts to understand how complex statistical models are used to make decisions.
The report does make an important point: if machine learning is used in any form of criminal justice setting, non-experts should be able to understand how decisions have been made and determine whether the outcomes are accurate and fair.
All things considered, the principal of the police using machine learning to identify risk and support decision making, is not – and should not – be considered as a new form of totalitarianism that seeks to erode democratic rights, prevent free speech, marginalize black and minority ethnic groups.
With rising crime in the UK now being the most significant issue facing the British public after Brexit, machine learning – within an appropriate ethical, regulatory and public trust framework – should have a place in the modern-day policing toolbox to prevent crime and protect the public.
This article is republished from The Conversation by Paul McFarlane, Senior Teaching Fellow in Security and Crime Science, UCL under a Creative Commons license. Read the original article.
Get the TNW newsletter
Get the most important tech news in your inbox each week.