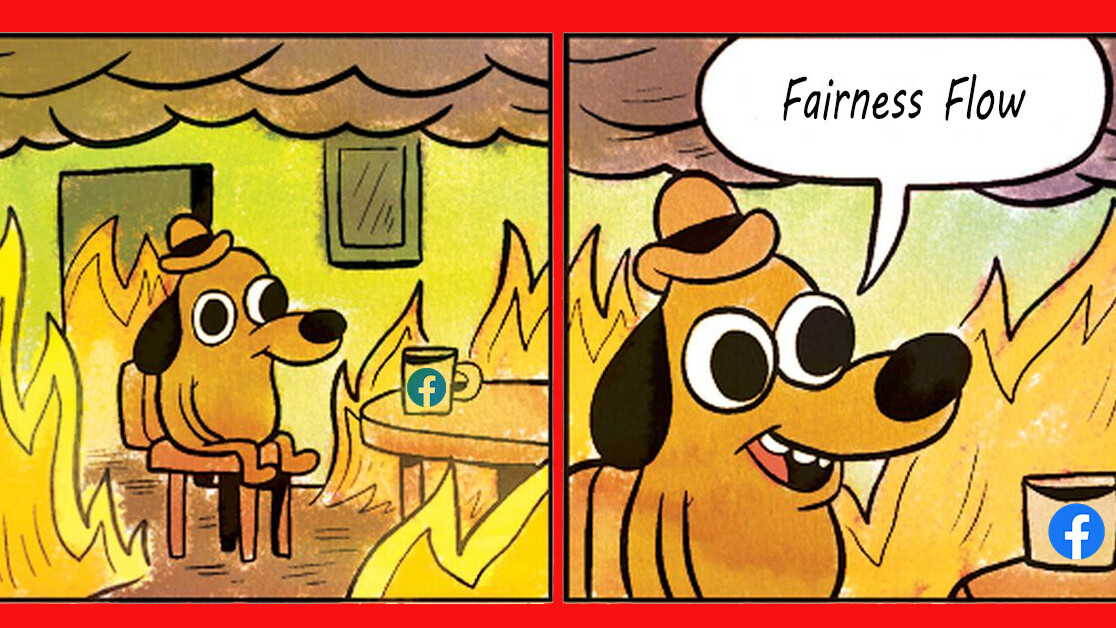
Facebook today posted a blog post detailing a three-year-old solution to its modern AI problems: an algorithm inspector that only works on some of the company’s systems.
Up front: Called Fairness Flow, the new diagnostic tool allows machine learning developers at Facebook to determine whether certain kinds of machine learning systems contain bias against or towards specific groups of people. It works by inspecting the data flow for a given model.
Per a company blog post:
To measure the performance of an algorithm’s predictions for certain groups, Fairness Flow works by dividing the data a model uses into relevant groups and calculating the model’s performance group by group. For example, one of the fairness metrics that the toolkit examines is the number of examples from each group. The goal is not for each group to be represented in exactly the same numbers but to determine whether the model has a sufficient representation within the data set from each group.
Other areas that Fairness Flow examines include whether a model can accurately classify or rank content for people from different groups, and whether a model systematically over- or underpredicts for one or more groups relative to others.
Background: The blog post doesn’t clarify exactly why Facebook’s touting Fairness Flow right now, but its timing gives a hint at what might be going on behind the scenes at the social network.
MIT Technology Review’s Karen Hao recently penned an article exposing Facebook’s anti bias efforts. Their piece makes the assertion that Facebook is motivated solely by “growth” and apparently has no intention of combating bias in AI where doing so would inhibit its ceaseless expansion.
Hao wrote:
It was clear from my conversations that the Responsible AI team had failed to make headway against misinformation and hate speech because it had never made those problems its main focus. More important, I realized, if it tried to, it would be set up for failure.
The reason is simple. Everything the company does and chooses not to do flows from a single motivation: Zuckerberg’s relentless desire for growth.
In the wake of Hao’s article, Facebook’s top AI guru, Yann LeCun, immediately pushed back against the article and its reporting.
No it doesn't.
Apparently, one can write about AI fairness without paying attention to journalistic fairness.
One of those cases where one knows the reality, reads an article about it, and go "WTF?"— Yann LeCun (@ylecun) March 12, 2021
Facebook had allegedly timed the publishing of a research paper with Hao’s article. Based on LeCun’s reaction, the company appeared gobstruck by the piece. Now a scant few weeks later, we’ve been treated to a 2,500+ word blog post on Fairness Flow, a tool that addresses the exact problems Hao’s article discusses.
[Read: Facebook AI boss Yann LeCun goes off in Twitter rant, blames talk radio for hate content]
However, addresses might be too strong a word. Here’s a few snippets from Facebook’s blog post on the tool:
- Fairness Flow is a technical toolkit that enables our teams to analyze how some types of AI models and labels perform across different groups. Fairness Flow is a diagnostic tool, so it can’t resolve fairness concerns on its own.
- Use of Fairness Flow is currently optional, though it is encouraged in cases that the tool supports.
- Fairness Flow is available to product teams across Facebook and can be applied to models even after they are deployed to production. However, Fairness Flow can’t analyze all types of models, and since each AI system has a different goal, its approach to fairness will be different.
Quick take: No matter how long and boring Facebook makes its blog posts, it can’t hide the fact that Fairness Flow can’t fix any of the problems with Facebook’s AI.
The reason bias is such a problem at Facebook is because so much of the AI at the social network is black box AI – meaning we have no clue why it makes the output decisions it does in a given iteration.
Imagine a game where you and all your friends throw your names in a hat and then your good pal Mark pulls one name out and gives that person a crisp five dollar bill. Mark does this 1,000 times and, as the game goes on, you notice that only your white, male friends are getting money. Mark never seems to pull out the name of a woman or non-white person.
Upon investigation, you’re convinced that Mark isn’t intentionally doing anything to cause the bias. Instead, you determine the problem must be occurring inside the hat.
At this point you have two decisions: number one, you can stop playing the game and go get a new hat. And this time, you try it out before you play again to make sure it doesn’t have the same biases.
Or you could go the route that Facebook’s gone: tell people that hats are inherently biased, and you’re working on new ways to identify and diagnose those problems. After that, just insist everyone keep playing the game while you figure out what to do next.
Bottom line: Fairness Flow is nothing more than an opt-in “observe and report” tool for developers. It doesn’t solve or fix anything.
Get the TNW newsletter
Get the most important tech news in your inbox each week.