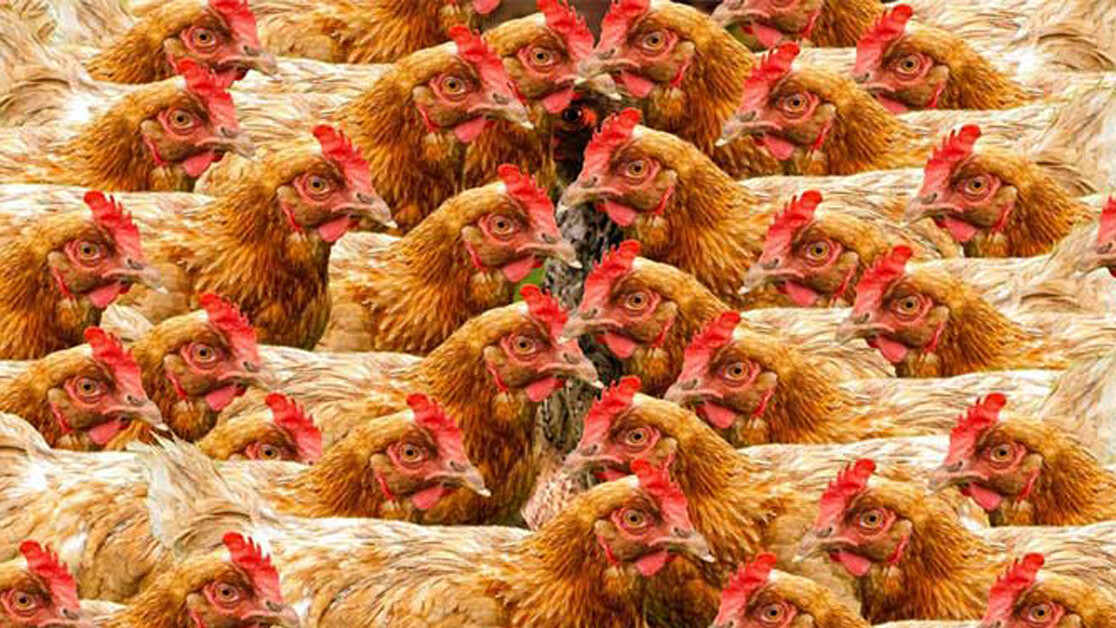
Researchers have developed an algorithm that uses satellite imagery to rapidly pinpoint industrial livestock farms, manifold faster than humans can do by manually analyzing maps. Now, the researchers think their new analytical tool could help regulators to identify farms that may be breaking environmental laws.
The research, led by a team from Stanford University, was premised on the fact that intensive animals farms are notoriously difficult to monitor and regulate in the United States – while also being a huge source of freshwater pollution.
Over 40 percent of the country’s livestock now comes from concentrated animal feeding operations (CAFOs), defined as sites where more than 1000 animals are farmed in confined spaces for 45 days or more. These intensive sites collectively produce 335 million tons of waste annually, which frequently enters natural water bodies, untreated. Agriculture is now the leading cause of water pollution in the US – and CAFOs are a big part of that.
These farms are governed by laws: CAFOs discharging effluent into water bodies are required to apply for a permit, which imposes limits that the farm is expected to abide by. But many farms that should apply for discharge permits simply don’t. As a yardstick, it’s estimated that roughly 60 percent of CAFOs in the United States don’t hold permits. And what worsens this problem is that there’s no reliable list of these farms in the United States. So regulators who want to locate CAFOs have to do so manually, which means people poring over maps for weeks.
This “information deficit” makes it difficult to monitor farms and enforce the country’s environmental laws, the researchers say. But that’s what they hope to remedy with their new tool.
The researchers developed a computer model to visually analyze satellite imagery of North Carolina state, and trained it to identify structures across the landscape that represented CAFOs where pigs or poultry are farmed. They decided to focus particularly on poultry farms, because these can contribute just as much waste as pig farms, but they operate largely without permits in North Carolina. It’s highly possible, therefore, that their environmental impact goes unregistered.
By chewing through the visual data, the algorithm ultimately identified an additional 580 poultry farms in North Carolina that hadn’t been identified in the original, manually-derived dataset. That’s an increase of 15 percent. In principle, this means that several hundred farms that previously weren’t known to environmental regulators could now be brought under their watch.
This is significant also because the algorithm accomplished it in under two days, whereas the researchers estimated it would have taken a team of people six weeks to accomplish the same thing by manually tagging CAFOs on a map.
The model also showed it could make estimates about changes to the landscape over time, using industrial feed mills as indicators. Feed mills are essential structures that CAFOs depend on to supply food for their livestock. So it’s logical that once a huge new mill goes up, there will be an increase in the number of surrounding CAFOs. By analyzing the land area around an industrial feed mill, the algorithm was able make reliable estimates about the number of new CAFOs that opened up after new structures went up. This kind of analysis could help regulators keep tabs on the extent and speed with which CAFOs spread across a particular region. In general, the algorithm could also highlight CAFOs that are closer to water bodies, and which therefore pose a higher pollution risk.
It’s clear, however, that identifying CAFOs only solves part of the problem of agriculture’s rampant water pollution in the US, because it’s one step away from identifying the pollution itself. But for now, the researchers say that the algorithm’s crucial benefit is that by so dramatically speeding up the identification process, it frees up weeks of time which could be better-spent monitoring farms, or prosecuting errant polluters. They estimate that manually searching maps of the whole US would take a roughly seven years – an untenably long time. Compared to that, the researchers insist that “our approach promises to save considerable resources.”
Now that they’ve proved its value in North Carolina, they believe the tool could also make an impact in other parts of the world, with a goal of improving agricultural transparency. That may be especially true in countries that have seen recent, rapid increases in industrial agriculture – where pollution may similarly be going unnoticed and unchallenged.
This article was originally published on Anthropocene Magazine by Emma Bryce. You can read it here.
TNW Conference 2019 is coming! Check out our glorious new location, inspiring line-up of speakers and activities, and how to be a part of this annual tech bonanza by clicking here.
Get the TNW newsletter
Get the most important tech news in your inbox each week.