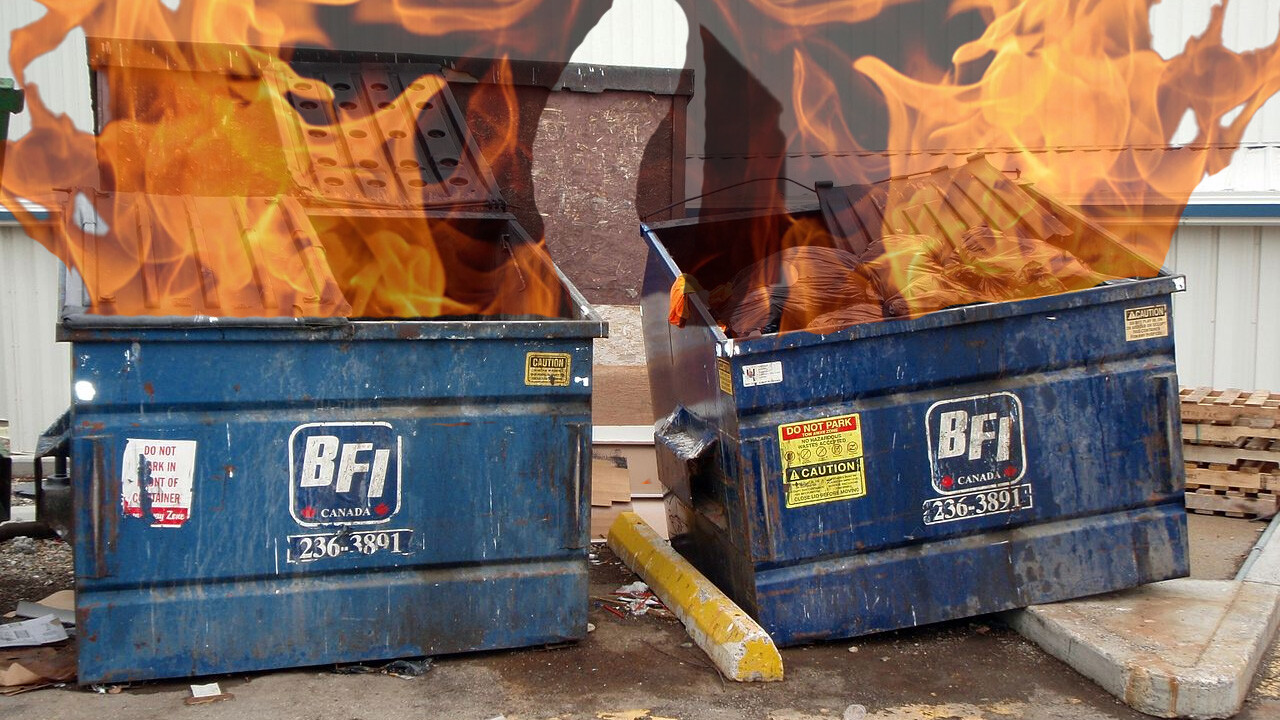
There are two realities when it comes to artificial intelligence. In one, the future’s so bright you need to put on welding goggles just to glance at it. AI is a backbone technology that’s just as necessary for global human operations as electricity and the internet. But in the other reality, winter is coming.
An “AI winter,” is a period in which nothing can grow. That means nobody’s hiring, nobody’s acquiring, and nobody’s funding. But this impending barren season is special, it won’t affect the entire industry.
In fact, most of the experts won’t even notice it. Google, OpenAI, DeepMind, Nvidia, Meta, IBM, and any university doing legitimate research have nothing to worry about. Startups with a clear, useful purpose will be fine — typical market issues notwithstanding.
The only people who need to be concerned about the coming chill are those trying to do what we’re going to refer to as “black box alchemy.”
Black box alchemy
I shudder to call any AI endeavor “alchemy,” because at least the idea of turning one metal into another has some scientific merit.
I’m talking about the wildly popular research vein wherein researchers build crappy little prediction models and then make up fake problems for the AI to be better at solving than humans.
When you write it all out in one sentence, it sounds like it should be obvious that it’s a grift. But I’m here to tell you that black box alchemy represents a huge portion of academic research right now, and that’s a bad thing.
Black box alchemy is what happens when AI researchers take something an AI is good at — such as returning relevant results when you search for something on Google — and try to use the same principles to do something that’s impossible. Since the AI can’t explain why it comes to the results it does (because the work happens in a black box which we can’t see inside) the researchers pretend they’re doing science without having to show any work.
It’s a scam that plays out in myriad paradigms ranging from predictive policing and recidivism algorithms all the way to bullshit pop facial recognition systems alleged to detect everything from a person’s politics to whether they’re likely to become a terrorist.
The part that cannot be stressed enough is that this particular scam is being perpetuated throughout academia. It doesn’t matter if you’re planning to attend a community college or Stanford, black box alchemy is everywhere.
Here’s how the scam works: researchers come up with a scheme that allows them to develop an AI model that is “more accurate” at a given task than humans are.
This is, quite literally, the hardest part. You can’t pick a simple task, such as looking at images and deciding whether there’s a cat or a dog in them. Humans will wreck the AI at this task 100 out of 100 times. We’re really good at telling cats from dogs.
And you can’t pick a task that’s too complicated. For example, there’s no sense in training a prediction model to determine which 1930s patents would be most relevant to modern thermodynamics applications. The number of humans that could win at that game is too small to matter.
You have to pick a task that the average person thinks can be observed, measured, and reported on via the scientific method, but that actually can’t.
Once you’ve done that, the rest is easy.
Gaydar
My favorite example of black box alchemy is the Stanford Gaydar paper. It’s a masterpiece in bullshit AI.
Researchers trained a rudimentary computer vision system on a database of human faces. The faces were labeled with self-reported tags indicating whether the individual pictured identified as gay or straight.
Over time, they were able to reach superhuman levels of accuracy. According to the researchers, the AI was better at telling which faces were gay than humans were, and nobody knows why.
Here’s the truth: no human can tell if another human is gay. We can guess. Sometimes we might guess right, other times we might guess wrong. This isn’t science.
Science requires observation and measurement. If there’s nothing to observe or measure, we cannot do science.
Gayness is not a ground truth. There’s no scientific measurement for gayness.
Here’s what I mean: are you gay if you experience same-sex attraction or only if you act on it? Can you be a gay virgin? Can you have a queer experience and remain straight? How many gay thoughts does it take to qualify you as gay, and who gets to decide that?
The simple reality is that human sexuality isn’t a point you can plot on a chart. Nobody can determine whether someone else is gay. Humans have the right to stay in closets, deny their own experiential sexuality, and decide how much “gayness” or “straightness” they need in their own lives to determine their own labels.
There is no scientific test for gay. And that means the Stanford team can’t train an AI to detect gayness; it can only train an AI to try and beat humans in a discrimination game that has no positive real-world use case.
Three solutions
The Stanford gaydar paper is just one of thousands of examples of black box alchemy that’s out there. Nobody should be surprised that this line of research is so popular, it’s the low-hanging fruit of ML research.
Twenty years ago, the number of high school graduates interested in machine learning was a drop in the bucket compared to how many teens are heading off to university to get a degree in AI this year.
And that’s both good and bad. The good thing is that there are more brilliant AI/ML researchers in the world today than ever — and that number is just going to keep growing.
The bad thing is that every AI classroom on the planet is littered with students who don’t understand the difference between a Magic 8-Ball and a prediction model — and there’s even less who understand why the former’s more useful for predicting human outcomes.
And that brings us to the three things every student, researcher, professor, and AI developer can do to make the entire field of AI/ML better for everyone.
- Don’t do black box alchemy. The first question you should ask before beginning any AI project related to prediction is: will this affect human outcomes? If the only science you can use to measure your project’s efficacy is to compare it to human accuracy, there’s a good chance you’re not doing great work.
- Don’t create new models with the sole purpose of surpassing the benchmarks set by previous models just because you can’t afford to curate useful databases.
- Don’t train models on data you can’t guarantee to be accurate and diverse.
I’d like to just end this article with those three tidbits of advice like some kind of smug mic drop, but it’s not that kind of moment.
The fact of the matter is that a huge portion of students are likely to struggle to do anything novel in the field of AI/ML that doesn’t involve breaking all three of those rules. And that’s because black box alchemy is easy, building bespoke databases is damn near impossible for anyone without big tech’s resources, and only a handful of universities and companies can afford to train large-parameter models.
We’re stuck in a place where the vast majority of students and would-be developers don’t have access to the resources necessary to go beyond trying to find “cool” ways to use open-source algorithms.
The only way to power through this era and into a more productive one, is for the next generation of developers to rebuke the current trends and carve a path away from the status quo — just like the current crop of pioneering AI developers did in their day.
Get the TNW newsletter
Get the most important tech news in your inbox each week.