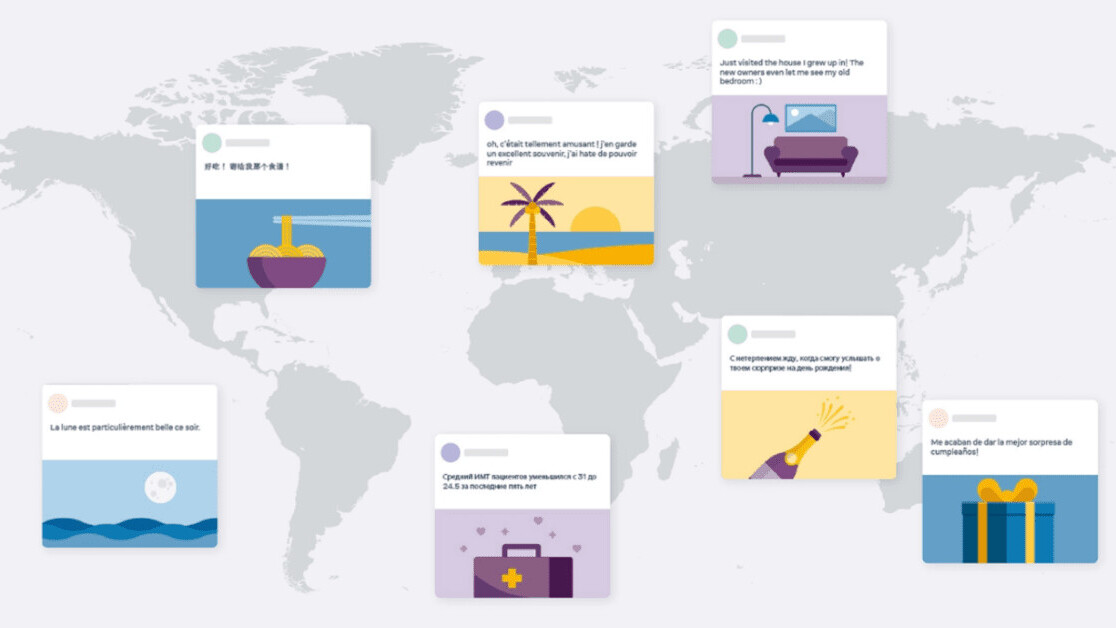
Facebook has open-sourced an AI model that can translate between any pair of 100 languages without first translating them to English as an intermediary step.
The system, called M2M-100, is currently only a research project, but could eventually be used to translate posts for Facebook users, nearly two-thirds of whom use a language other than English.
“For years, AI researchers have been working toward building a single universal model that can understand all languages across different tasks,” said Facebook research assistant Angela Fan in a blogpost.
“A single model that supports all languages, dialects, and modalities will help us better serve more people, keep translations up to date, and create new experiences for billions of people equally. This work brings us closer to this goal.”
[Read: Researchers use AI to translate text found on ancient clay tablets]
The model was trained on a dataset of 7.5 billion sentence pairs across 100 languages that were mined from the web. Facebook says all of these resources are open source and use publicly available data.
To manage the scale of the mining, the researchers focused on language translations that were most commonly requested and avoided the rarer ones, such as Sinhala-Javanese.
They then grouped the languages into 14 different groups, based on linguistic, geographic, and cultural similarities. This approach was chosen because people in countries with languages that share these characteristics would be more likely to benefit from translations between them.
For instance, one group included common languages in India, such as Hindi, Bengali, and Marathi. All the possible language pairs within each group were then mined.
The languages of different groups were connected through a small number of bridge languages. In the example of the Indian language group, Hindi, Bengali, and Tamil served as bridge languages for Indo-Aryan languages.
The team then mined training data for all combinations of these bridge languages, which left them with a dataset of 7.5 billion parallel sentences corresponding to 2,200 translation directions.
For languages lacking quality translation data, the researchers used a method called back-translation to generate synthetic translations that can supplement the mined data.
This combination of techniques resulted in the first multilingual machine translation (MMT) model that can translate between any pair of 100 languages without relying on English data, according to Facebook.
“When translating, say, Chinese to French, most English-centric multilingual models train on Chinese to English and English to French, because English training data is the most widely available,” said Fan. “Our model directly trains on Chinese to French data to better preserve meaning.”
The model has not yet been incorporated in any products, but tests suggest it could support a wide variety of translations on Facebook, where people post content in more than 160 languages. The company says it outperformed English-centric systems by 10 points on the BLEU metric for assessing machine translations.
Get the TNW newsletter
Get the most important tech news in your inbox each week.