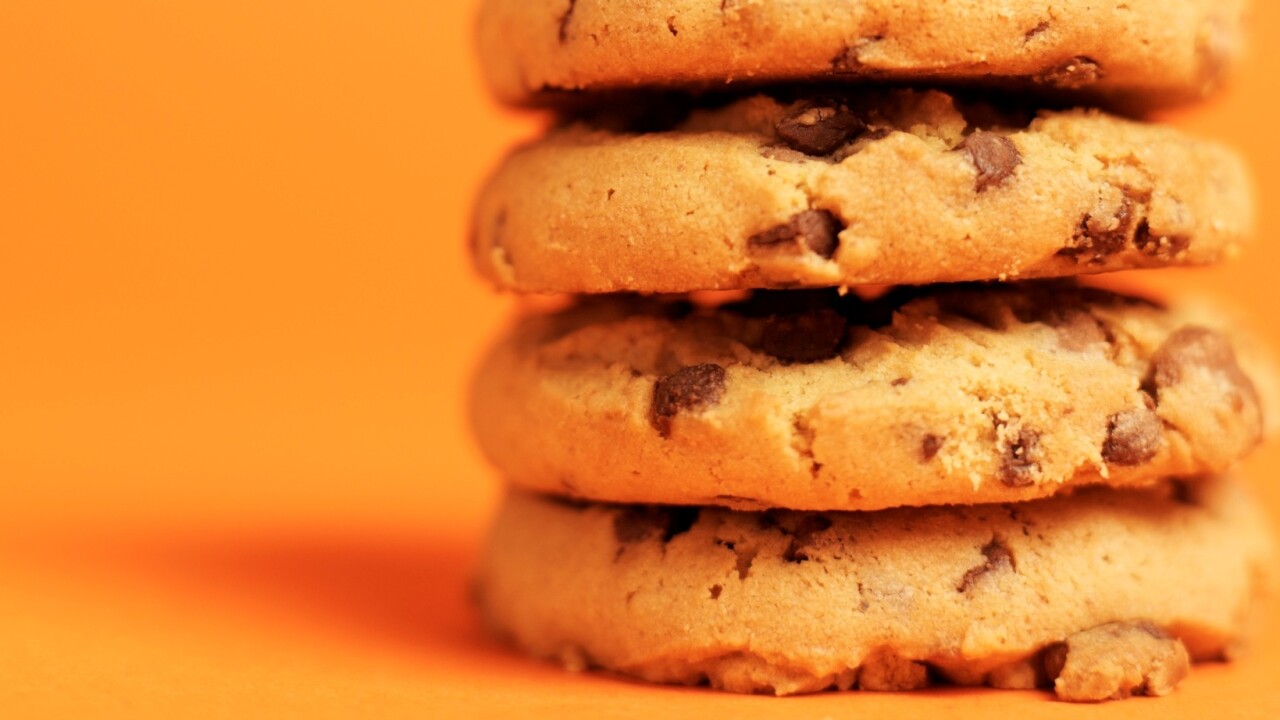
Jonathan Lakin is the CEO of Intent HQ, an audience intelligence company that helps turns social data into revenue with customised user experiences.
Most online advertising and audience targeting mediums – ones that are used to target the ads you see on Web pages – are cookie-based. This means that although a large profile can be built about an individual, it is all based on behavioural data.
This should be fine, right? Your past behaviour on the Web is likely to be similar to future behaviour.
That sounds like a fair assumption, but it hides a lot of inaccuracy. Think about the reasons you search for something online. They could range from work research to gift buying for your 13-year-old niece over this past Christmas. The data will show what you are doing, but not why.
Behaviour versus interests
Can we expect an algorithm to understand the reasons behind your online behaviour, in order to get an accurate picture of who you are? Yes, but not based solely on behavioural data.
The best way to get an accurate picture is to focus on your interests; you may be searching for a Justin Bieber album, but if we know Justin’s music is not one of your interests we can start to understand why you’re looking for him, and why you probably won’t do it again in the near future.
We can also start to create a Web experience that is based on your interests, and not your 13-year-old niece’s.
Cookies and the filter bubble
One of the negative impacts of the cookie driven, behaviour-based personalised Web, as it has existed so far, is the filter bubble effect.
If your personalised Web experience is based purely on your past behaviour, you are going to get more of what you’ve seen before. As a user, you are doomed to repeat yourself.
The only way out of the bubble is to create some kind of serendipity, and that cannot be achieved with the data that cookies give us.
Serendipity and interests
Have you ever found yourself looking at your Netflix recommendations or Last.fm play queue, wanting to tell the service that you are more than the product of an algorithm?
You may have watched three or four sombre documentaries, but that isn’t to say you can’t appreciate a light-hearted comedy every now and again. And just because you were very into ambient electronica last week, doesn’t mean you wouldn’t like to hear some hard rock, depending on the occasion.
Creating serendipitous Web experiences
Social networks represent by far the largest amount of time spent on the Web. Here we share vast volumes of data on our interests. By tapping into this data, it is possible to create serendipitous Web experiences.
Simply knowing what your interests are is not enough, as there are still limitations that could lead to a filter bubble effect.
As human beings, we have a very complex understanding of the affinity between topics. In order for a machine to make these kinds of connections, it needs to have a human-like understanding of these affinities.
This means going beyond simple relationships like ‘1,000 people who liked Ernest Hemingway also liked F Scott Fitzgerald’, towards relationships like: ‘Hemingway was a writer of a similar era and style to Fitzgerald’.
This kind of data can be used to create a complete, real picture of an individual that identifies complex affinities between interests. A picture of an individual who can like Metallica and TLC, or might enjoy “Shawshank Redemption” and “A Bug’s Life.”
A large part of this is the ability to understand and interpret context, what something means to you at one moment may well be different at another moment in time. Understanding context requires the ability to understand the relationships between things, which is why social networks are built on graph databases, where understanding relationships is more important than transactions.
Google is using all of its data assets, from email to search to maps, to develop a better understanding of context, and you can see this in its latest algorithm update, Hummingbird.
Using Google’s voice search on your mobile device, hit the microphone icon and say ‘Chelsea Football Club’, the phone will tell you (verbally as well as in text) the team’s latest score and next fixture. Hit the icon again and ask ‘where do they play?’ and it will say ‘Stamford Bridge’. Google is using its huge wealth of data here to predict what you might actually want to know, and make the kind of connections a human might when you ask a second question.
Another approach for developers is to use Wikipedia. As the largest database of fully referenced human curated (relatively) reliable information ever created, it is a fantastic basis for an algorithm with a human like understanding.
Looking at the way Wikipedia’s own pages link to and reference each other creates a very near complete replication of human-like understanding of topic affinity.
This kind of data, when married to the personal interest data shared on social networks, can be used to go far beyond the kind of Web experiences that cookie-based data has ever allowed us to create.
Get the TNW newsletter
Get the most important tech news in your inbox each week.