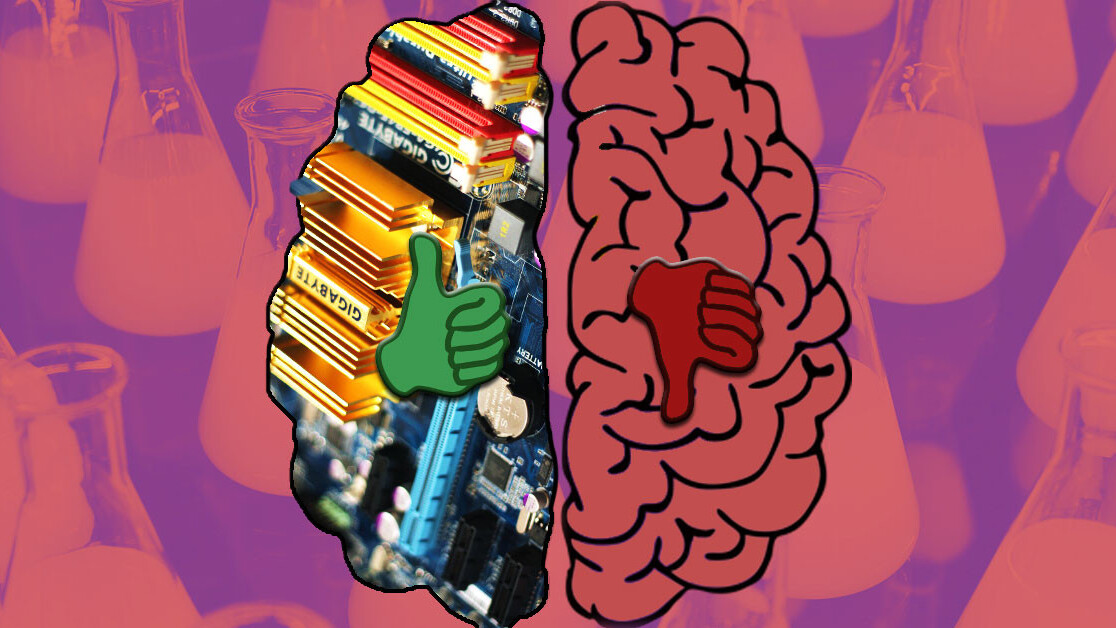
Machines don’t actually have bias. AI doesn’t ‘want’ something to be true or false for reasons that can’t be explained through logic. Unfortunately human bias exists in machine learning from the creation of an algorithm to the interpretation of data – and until now hardly anyone has tried to solve this huge problem.
A team of scientists from Czech Republic and Germany recently conducted research to determine the effect human cognitive bias has on interpreting the output used to create machine learning rules.
The team’s white paper explains how 20 different cognitive biases could potentially alter the development of machine learning rules and proposes methods for “debiasing” them.
Biases such as “confirmation bias” (when a person accepts a result because it confirms a previous belief) or “availability bias” (placing greater emphasis on information relevant to the individual than equally valuable information of less familiarity) can render the interpretation of machine learning data pointless.
When these types of human mistakes become baked-in parts of an AI — meaning our bias is responsible for the selection of a training rule that shapes the creation of a machine learning model– we’re not creating artificial intelligence: we’re just obfuscating our own flawed observations inside of a black box.
According to the paper, this is all new territory:
Due to lack of previous research, our review transfers general results obtained in cognitive psychology to the domain of machine learning. It needs to be succeeded by empirical studies specifically aimed at the machine learning domain
The landscape of personal responsibility is changing as more AI-powered systems come online. Soon most vehicles will be operated by machines and a large number of surgeries and medical procedures will be conducted by robots. That’s going to put AI developers front and center when tragedy strikes and people look for someone to blame.
The researchers propose a debiasing solution to each cognitive bias they examined. For many of the problems the solution was as simple as changing the way data is represented. The team hypothesizes, for example, changing the output of algorithms to use more natural numbers than ratios could substantially reduce the potential for misinterpreting certain results.
Unfortunately there’s no easy fix for the overall problem. Most of the time we don’t know that we’re being biased. We believe we’re being clever or intuitive – or we just don’t think about it. And there’s far more than just 20 different cognitive biases that machine learning programmers need to be concerned with.
Even when the algorithms are perfect and the outputs are immutable, our cognitive biases make our interpretation of data unreliable at best. Everyone has these biases to one degree or another – which makes it concerning that there’s been so little research on how they effect data interpretation.
According to the team:
To our knowledge, cognitive biases have not yet been discussed in relation to interpretability of machine learning results. We thus initiated this review of research published in cognitive science with the intent to give a psychological basis to changes in inductive rule learning algorithms, and the way their results are communicated. Our review identified twenty cognitive biases, heuristics and effects that can give rise to systematic errors when inductively learned rules are interpreted.
It’s important that researchers around the world build on this work and discover methods to avoid cognitive bias in machine learning entirely. Otherwise AI is nothing more than an amplifier for human BS. And bad science is bound to make bad robots.
The Next Web’s 2018 conference is almost here, and it’ll be ??. Find out all about our tracks here.
Get the TNW newsletter
Get the most important tech news in your inbox each week.