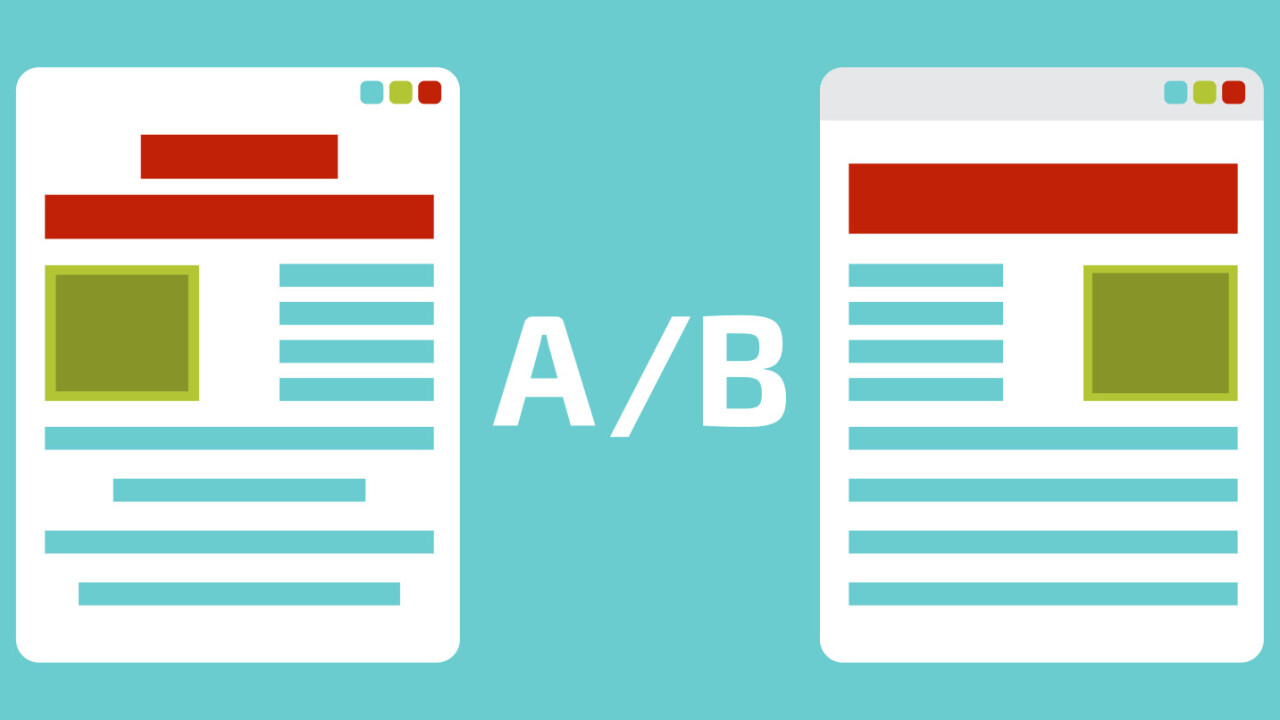
In this series of blog posts I’d love to shed some light onto how we approach marketing at The Next Web through Web analytics, Search Engine Optimization (SEO), Conversaion Rate Optimization (CRO), social media and more. This second blog post focuses on the process that we set up for A/B testing at The Next Web. Read the first blog post on heat maps.
Before I start I’d like to give a short explanation as to why we do A/B testing at The Next Web and what this process looked like in the past.
Why do we test at The Next Web? We want to understand what motivates and triggers users to stay engaged more (or less) with TNW.
Who runs testing at The Next Web? Our marketing team currently has two to three team members involved in our A/B testing program. Our Web analyst runs the day-to-day operations of our testing program and is supported by our analytics & research intern. When we need additional support, our developers and the rest of the team pitch in with new ideas.
Our testing program began a year ago with one test a month, run, coded and analysed by just me. There wasn’t a lot of time available, due to our very small marketing team, and as a result testing was not a top priority as we had multiple other channels to attend to. When we hired our Web analyst at the beginning of the year it freed up time and allowed us to improve testing.
Currently we run 15-20 tests each month, but we had to build up to this number. But we believe that if we continue improving at this rate, we will have run more than 200 A/B tests this year. So what does our process look like?
Know your goals & KPIs
All projects at The Next Web, like TNW Academy, TNW Deals, Index.co etc., aim to increase unique visitors and pageviews, but we do have other “main goals” across the projects, like increasing revenue for TNW Deals for which we have to create individual templates.
Testing seems simple but it is in fact vital to do, as customizing our testing plans for specific needs greatly helped in scaling our efforts. Once we defined the goals for each project, we defined templates for the site (for the blog this would be the homepage, category pages, article pages, author pages, search etc). We create so many because we need to find out which templates are the most important so later on we can prioritize them.
For most pages we track the basic engagement metrics on Google Analytics: bounce rate, time on page, etc. However, for many of these pages Google Analytics does not trace all elements that are important to us so we defined click-through rate (CTR) areas and elements like related stories, share buttons, sidebar, popular v.s. latest etc. They have different click-through rates and we want to know how changing one element will affect the others. That’s why we’re monitoring changes on secondary goals.
All of our goals and KPIs are measured through Google Analytics. In a later blog post I will talk more about how we did our web analytics set up.
Lifetime v.s. significance: Now that we know what kind of KPIs we have for certain pages, we not only know what we need to focus on but we can also calculate the time needed for a test to run.
If you know the number of visitors on a certain page and the click-through rate/conversion rate for a certain objective you can calculate (using this tool) if your lifecycle has enough significance and the right power level. In our case, because of the traffic + CTR on certain elements, it means that for most pages we can run multiple tests at the same time due to our traffic numbers. For article pages, for example, we can run three tests on desktop and three tests on mobile per week, and still have a 95+ percent significance + 90+ percent power level.
Know your users
For a successful marketing project, we find it important to become thoroughly acquainted with the user. We investigate what triggers engagement, our KPIs, and the different segment of users important to us.
To discern whether or not a user is of interest to your company, you must consider the following:
- new or returning visitors
- device categories (desktop, mobile, tablet),
- traffic sources (SEO, Facebook, direct),
- templates (homepage, article, author, search)
If you have enough traffic, you can also run tests on these segments and analyze the differences in behaviour between user types. This means the more users you have, the more tests you can run as you divide your traffic based on user segments; running one test on article pages for new users, running one test on article pages for returning visitor, running one test on Facebook users, and so on. For most of our tests, we run a bigger sample size and then drill down into our audience traffic sources – making sure that these segments are already defined within the hypothesis of an experiment.
Identifying opportunities & experiments > brainstorm
If you want to run around 200 tests a year it’s important to overshoot this number when creating A/B ideas and make extras as well, though we’re obviously not only testing for quantity over quality. If you do this, you can prioritize tests and only run those with the highest potential impact. That’s why we usually have a backlog of around 30-40 tests ready to go at any time.
60-80 percent of these ideas are generated by the team working on our A/B testing program, the rest are created with the help of the whole company, from the social media team to our CEO Boris. Non-marketing team members aren’t required to come up with the strict definition of a test they want to run– it’s up to our team to make sure it’s formatted the correct way. Whenever they come up with an idea we make sure it ends up in our backlog and then supply the supporting data.
Many opportunities for are also generated during our quarterly meetings with our CEO. More on this later.
Defining & Hypothesizing opportunities
An important part of A/B testing is ensuring that we have discovered the right definition, hypothesis, templates and user segments to run the experiment on. We do this by checking what kind of an impact it has on a certain user segment, but also on the element that we’d like to test. If we change an element for only mobile users, it’s useless to run the test on desktop visitors too.
In addition, we usually already have a defined hypothesis of what we expect to get out of the test both in results and in new information. A hypothesis helps us to measure our expectations against the reality. Therefore, we usually know what we expect to learn from a test, and how the test will help us better understand the user.
Testing documentation
To make sure we can keep track of all the experiments, their schedule and the ideas behind the, we keep all tests systematically documented. In a future blog post I’d like to give you a rundown of the tools we built for this, but for now we’ll give you a sneak peek. This is the information we require for our testing documentation:
- Experiment ID: a number identifying the test we run, used for identifying the test in Google Analytics.
- Experiment Name
- Device category: Desktop, Desktop + Mobile, Mobile, Tablet, Tablet + Mobile.
- Owner: who created the idea and is responsible for the test?
- Template
- Objective metric
- Experiment status: Hypothesis, Building, Running, Analysed, Finished.
- Begin date
- Run time: 7, 14, 21 or 28 days.
- Implemented date: the date a certain experiment was implemented if it has a winning variant.
- Description: a short explanation of what our test entails.
- Hypothesis
- Measuring plan: a short explanation on how we track the experiment.
When collecting the data for experiments we also save 2 extra fields for variants within an experiment: a url to the screenshot of the variant and if it was a winner or not.
It’s a lot of data to record, but all of this information ensures that after six months we can look back and see what kind of tests we’ve run in a certain period. Thorough documentation is vital for bigger companies running a testing program with bigger teams.
Prioritizing opportunities
If you’ve accumulated a lot of ideas, it is important that you only focus on the tests that could have the biggest impact on your business goals.
As you increase engagement across the board it makes it easier to know which parts of the site to focus on. In our case, prioritising is quite easy; since we have the most traffic on our article pages, we put most of our time in running tests on these pages.
Still, we don’t (and can’t) over-prioritize. Since we run as many as 8-10 tests a week, we move quickly through the backlog after a brainstorm. All the resulting ideas are put in order according to the overall potential impact and an estimate of how many times it would need to implemented. We also consider if implementing it would require back-end development, but don’t score them at this point.
Designing, Coding & QA of Variations
Once we know what kind of tests we’d like to run we’re able to assess what we need to design and code. All of our ‘designing’ is directly done through the Web inspector of a browser to make sure we have the code to implement it later, which saves a lot of time. This has been a huge help, but we’re still trying to fine-tune the process even more.
This is how we do it:
Designing: As previously mentioned, plus we also add screenshots of elements from our tests. This makes it easier to come up with the design for an experiment, as previously designed elements evolve. If we don’t like the design, we then talk to our designers and they come up with a better idea on how to visualize it.
Coding: Coding is done through a browser Web inspector. Since we run our experiments with jQuery, it’s easy to add or change current DOM elements on our pages. If we need a new data element we’ll load it from the back-end and hide the element to make sure it won’t interfere with the original variant.
Q&A: It’s hard to make sure our tests are working across dozens of different devices and browser versions. That’s why most of our experiments are tested in the most popular browsers, and if those run properly then we’ll move on to check other browsers.
If the experiments work in there, we’ll stop Q&A to set up our tests as fast as possible.
Currently our process isn’t very intensive, but we also don’t come across that many broken tests, luckily.
Running experiments
Thi is probably the easiest step in our whole process. When a test is designed, coded and ready for testing, it’s implemented via Google Tag Manager. Our tests all have the same coding structure and with that it’s plain sailing -we upload it in GTM and publish the tests to the targeted pages.
Technical set up: We run all of our testing through Google Tag Manager with custom JavaScript to make the needed variants and to check if a user is already in a certain variant of an experiment. Tests are activated by using filters to target the user segments v.s. pages. For example: Desktop – Article Pages. If a certain test requires data from our back-end then code is inserted and hidden in the body to make sure it can be used by a variant but won’t have any impact on original variants.
This set up gives us complete flexibility in creating tests and targeting users and also allows us to save maximally on the costs of using an external platform.
We’ll announce tests on Slack so our team is aware of the changes. If the proverbial stuff hits the fan, then either our editorial or development team know to who to reach out to, to make sure we can stop these issues as soon as possible. Not much more to say about this step, except that we’ll have to wait for the results of a test obviously to get to the next steps.
Tip: Sharing the tests that you’re going to run makes sure that team members feel more involved and come up with ideas themselves, which then makes it easier to identify opportunities.
Analyzing experiment variations
After the experiment has run its lifecycle (7, 14, 21, or 28 days– we’ll never run a test longer than 4 weeks), it’s time to start analyzing the tests.
As we save the variants of a test in a custom dimension we can easily create reports for the performance of the test in Google Analytics. We know the main objective for a test (for example: increasing shares, clicks on related stories) so this, together with it’s significance, is the first thing we check.
If the test is either a ‘winner’ or a ‘loser’ we’ll get into more detail to see what kind of impact it has on either device categories or different user types. If there’s no big difference in either one of them (new v.s. returning or device categories) we’ll probably already have enough information to start implementing the winning variant.
We also look at other aspects of the test, like if a certain order of share buttons has negative impact on CTR on related stories, for example. As these tests all have their own goals in our setup, we can easily see what the differences are for the secondary goals of our test. We don’t test them on significance but it prevents us from analyzing broken tests that didn’t work in certain browsers or for certain segments of users.
Implementing winners
As X percent of our tests has a winning variant it means that we also have to implement a lot of winning variants. Luckily we have an awesome development team that completely supports our marketing team’s requirements.
With that help, we’re able to not only push tests live via a tag manager but also push code to our live servers, which allows us to run a winning variant for 100 percent of users within an hour. By pushing winners live as fast as possible, our program will have the highest potential impact.
I have to give some credit to our platform, WordPress, as it makes development really easy. Implementing new features or styles and having all our data in GIT makes it simple to cooperate with our developers. Is a variant too complicated for us to implement? We’ll ask one of our developers to pick it up, which usually results in it being implemented within a day or two.
Sharing results: winners & losers
In addition to announcing all of our tests when they start running, we also provide the team with a weekly update on the tests that have finished and been analyzed. We then share both the winners and the losers. This is done via the #marketing channel on our Slack account to reach the whole team (as people outside of the marketing team join the channel as well).
In our testing documentation we also mark the test as either a ‘winner’ or a ‘loser’ to make sure we keep track of our success percentage, currently X percent. This number is important for us as we like to come up with new ideas on how to make our return on testing higher. More successful tests means higher engagement.
We also have a quarterly meeting with our CEO Boris to make sure he’s up to date on the tests and ideas we have for the upcoming quarter.
In this meeting, we mostly focus on the bigger tests and the impact they could have on our goals and the design of the blog. Usually Boris comes up with a lot of new ideas based on where he wants to see improvement, and provides us with left-field suggestions too.
What has happened in the last 6 months?
We didn’t reach our current number of tests on day number one – it’s taken time to grow it to this point.
Based on the presentation I gave at Optimizely’s Opticon conference in June, I wrote a blog post covering how we scaled our testing program from one test a month to 10 tests a week.
What’s next?
Hopefully this post gave you a lot of insights on how we set up our testing program at The Next Web. But how are we going to improve our set up in the future and how can you help with that as an expert or user?
There are a lot of ways we can improve our process, some of the current ideas are:
- Run testing on even more templates: pages with relatively fewer pageviews are currently not included in our testing program, but could also have a potential impact on our engagement and goals.
- Create design style guides: as we have to deal with a lot of front-end code from time to time it could be useful to know what kind of code is being used where and how it reacts to certain changes.
- Slack integration: currently we already share our tests via Slack with the rest of our team. But automating this in our analytics tool would save us at least a couple of minutes for each test. So we might push our testing progress automatically via their API in the future.
- Bigger team: it’s pretty hard to keep up with coding over 10 tests a week with a small team. We’ll likely add more resources to the team by the end of the year to prepare for our goals for next year.
- Back-end testing: we’d like to run more testing from the back-end, to have even a better user experience, to decrease the flickering of pages but also to make it even easier to track the progress for our KPIs, and to allow us to do more multi-armed bandit testing.
- Personalization and behavioral targeting: we already have a lot of data on user behavior. In the upcoming year, you’ll likely see more tests based on this data.
- Your help: we’re always open for feedback on what you like and don’t like at The Next Web. It’s the basis for a lot of analysis and tests we do. I welcome you to share your ideas.
Read next: Marketing the TNW way #1: Heat maps
Next in this series? What kind of A/B tests did we run at what was their outcome?
Featured image credit – Shutterstock
This is a #TNWLife article, a look into life and work at The Next Web.
Get the TNW newsletter
Get the most important tech news in your inbox each week.