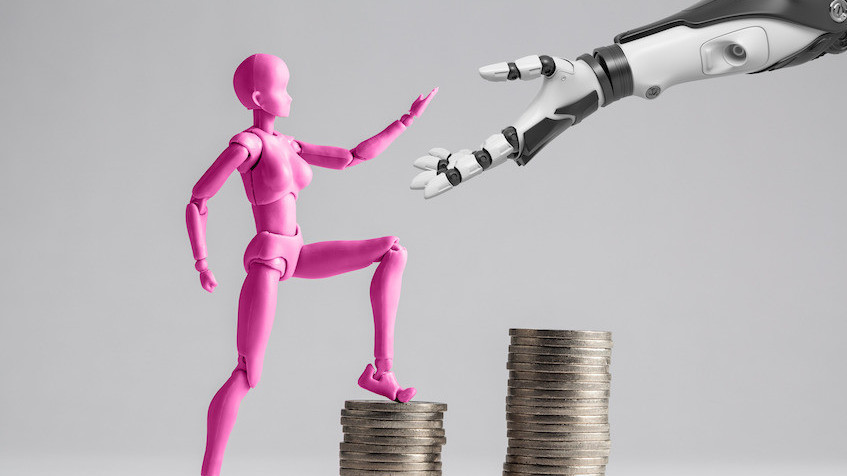
202 years. That’s how long it will take to close the gender gap unless we speed up the process, says a recent report by the World Economic Forum.
And looking at the statistics, this makes sense. A recent study by Pew Research found that the gender pay gap has narrowed but stayed relatively stable since… the 80s.
Somehow, we’ve learned how to build self-driving cars and smartphones with more computing power than the first computers used in space travel, but we still haven’t figured out how to pay men and women equally.
Luckily, social and government-led pressure is mounting. Movements like the Women’s March and #metoo are bringing discrimination into the spotlight, while many countries including Denmark, the UK, France, and Germany are now requiring companies to report on their gender pay gap every year.
It’s not just society and governments. With so much evidence of the bottom line benefits, diversity can bring to a company, more and more are looking to close their pay gap in order to attract more diverse talent.
With so much will and incentive to finally close the gap, why are we still not making gains? The emergence of new technologies may be able to give us the answer.
Why we’re not making progress
In a strange turn of events, Google recently reported it found a pay discrepancy which would actually have resulted in male engineers being paid less in 2019. Rather than it being a difference in salary, the discrepancy resulted in a larger percentage of discretionary funds being allocated to female engineers. As a result, they corrected this mistake ahead of time by increasing the expected wages of thousands of male Googlers.
Coming from a company which is now facing a potential class-action lawsuit from current and former female engineers and an investigation by the US Department of Labor for discrimination in pay and promotion, the new report is certainly raising eyebrows (and eye-rolls in some cases).
Google’s data only compares employees within the same pay grade. According to the New York Times, one of the plaintiffs bringing a lawsuit against the company claimed she was hired in at a lower level than male engineers with the same experience. Critics argue it’s likely the discrepancy actually comes from more experienced women being hired in at lower levels and then allocated more discretionary pay to compensate for the clear mistake in leveling.
It’s examples like these which clearly show the cracks in our data when it comes to solving the gender pay gap.
One of the biggest problems companies face is seeing the symptoms of the gap but not actually having the insights to identify the causes within their unique structural makeup. At the moment, most companies have their payroll and HR data on two separate systems meaning that discrepancies resulting from issues in their hiring or promotion structures aren’t accounted for.
Because of this lack of insights, we’re seeing a lot of companies spend massive amounts of time and resources on strategies that simply aren’t working. But AI and machine learning may be able to provide a more microscopic view of what’s actually happening.
Can AI and machine learning help close the gap?
Zara Nanu, CEO of Gapsquare, a software service that’s helping companies address the gender pay gap through its AI and machine learning technology, explained her view.
Oftentimes, companies will go through an almost manic push to solve their gender pay gap problems quickly. For example, some will try offering high starting salaries to attract more female applicants in the short run but, ultimately, if inequalities still exist in your leveling and later promotion and pay structures, this won’t result in a long term solution.
“Instead, we need to help companies understand how those discrepancies can be covered in a more sustainable way that doesn’t disadvantage either gender. It’s not about stealing a slice of the pie; it’s about building the pie together equally,” she explained.
Gapsquare’s technology allows companies to run their payroll and HR data through one system. Using AI and machine learning technology, they’re able to merge and analyze the data together providing expertise in three key areas:
- Identifying any existing pay discrepancies based on gender but also ethnicity, disability or any other employee characteristics
- Insights into why these gaps exist based on a combination of data and academic expertise from the backend
- Opportunities to take more data-driven decisions to narrow these gaps
As Nanu explained, a company’s data is often based on “complex reward and compensation structures with over a thousand different elements of pay.” With Gapsquare’s software, companies can look at both outright salary based inequalities but also, for example, discrepancies which could arise from less visible factors like housing and car allowances.
With this information, companies can zero in on which departments or at which levels glass ceilings exist and use data-driven decisions to create real sustainable change.
“Gender equality can be a very emotional and political topic within a company. You get people engaging in debates about whether they should introduce quotas or targets or other methods with very little action actually happening in the end. Whereas, with the software, we have the numbers and a graph and an explanation of why the numbers are what they are. This makes it a lot easier for managers to start taking data-driven decisions and moving things along,” she remarked.
The irony of using AI to tackle bias
Ironically, the AI field itself has one of the biggest gender gaps. Currently, only 22% of the AI workforce is made up of women. According to Nanu, “the gender pay gap is three times higher in AI. This is a major problem as this technology is actually shaping our future.”
At just 17, Sara Conejo Cervantes has become a spokeswoman for AI and gender, having spoken at the UN AI for Good Summit and the AI Europe Stakeholder Summit this past year. She explained that the looming gender pay gap is already top of mind for her and other girls looking to get into the field.
“If I go into the field and I have a colleague who is being paid more just because of their gender, I would feel like I’m losing out on something. It creates a path where women feel they have to prove themselves to be able to take on that role.”
The massive gender gap in the field is already showing its effect on the AI-powered tech we’re using today. For example, voice recognition bots like Siri, Alexa, Google Home, and Cortana all feature female voices as their primary setting.
“There’s a study that shows if you speak to Alexa or Siri in a passive-aggressive or even abusive way they usually brush off what you say or respond with a flirtatious remark.”
Just like in other applications of AI, we’re starting to see this issue more and more as it enters the field of HR Tech. Amazon attempted to use AI to create a fast moving recruitment tool, only to find it learned to penalize female applicants by allocating lower scores to resumes with the word “women’s” as in “women’s chess club captain” or those who attended women’s only colleges.
The problem is that AI and machine learning technology uses historical data to learn and make decisions. Ultimately, if your past data is biased, the outcomes will be too. As Conejo explained,
“Right now is when we’re starting to spot these patterns in algorithms, in particular in AI, and if we don’t start fixing them now, it’s going to be very hard in the future as they’ll already be deeply embedded into these algorithms.”
Gapsquare’s team also faced this challenge. The interesting thing they’ve found is that, after you take away the parts of a gap which can be explained by performance, length of service, and educational level, you’ll then still have about 60 percent unexplained which can be directly attributed to bias and societal norms impacting women’s career progression.
Their team is now working with experts from Harvard and the University of Exeter to introduce different parameters which take bias into account, essentially filtering out past discrimination. This will take time but:
“The way we see our work is that, yes, we’re now using AI to see how we can specifically unpick bias in recruitment and career progression but, at the same time, we want to make sure that what we develop is then applicable to other spheres where we will start to be more aware and conscious about bias and the need to control for it,” Nanu shared.
Using data to finally close the pay gap
If the WEF’s prediction is true, it means that young promising engineers like Conejo still won’t see the gender pay gap close within their lifetime. As Conejo explained,
“It’s not really in our control and that’s why, when I speak at conferences and other events, I really try to bring this up. If someone is doing the same job, they should be paid equally. But I tend to see that the people who are there don’t really have much interest in what I’m saying, both because I’m very young and also a female.”
Perhaps, with more women leveraging AI and machine learning to filter bias out of our data, we’ll have the insights we need to finally start narrowing the gap.
“If I walk into a board room and see there’s one woman standing in a crowd of 20 men it’s obvious there isn’t diversity and we need to encourage it. I think having the data can really reinforce that,” Conejo concluded.
By combining a passion for people with the power of today’s intelligent machines, Randstad supports people and organizations in realizing their true potential. Learn more about their innovative HR solutions here.
Get the TNW newsletter
Get the most important tech news in your inbox each week.