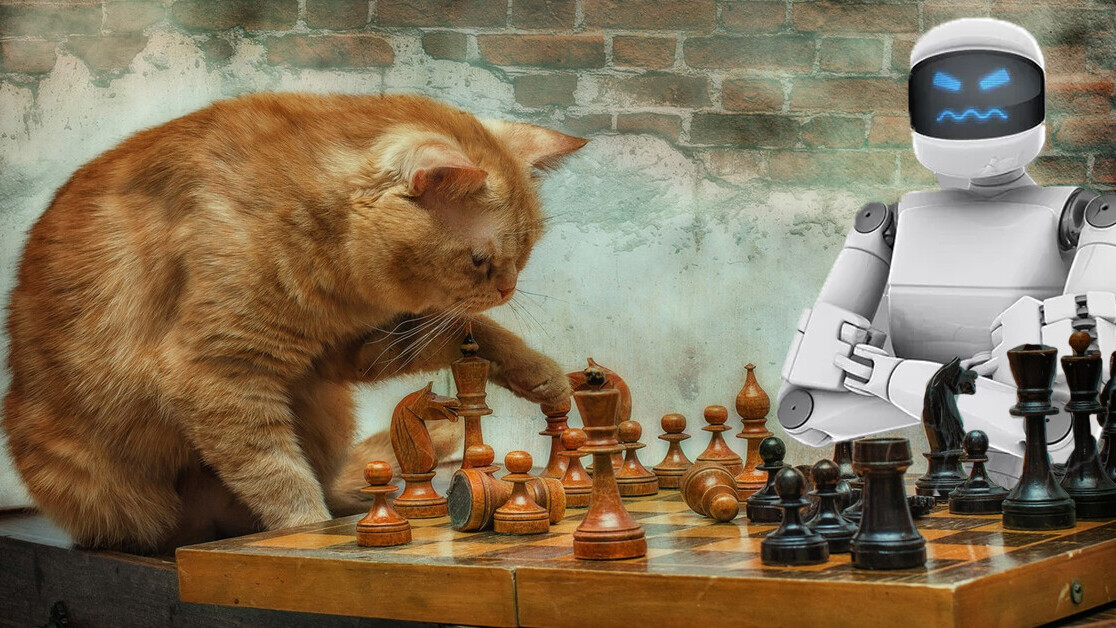
If you share your home with a dog or a cat, look at it carefully and you will get a good overview of everything we don’t know how to do in artificial intelligence.
“But my cat does nothing all day except sleep, eat and wash herself,” you may think. And yet your cat knows how to walk, run, jump (and land on her feet), hear, see, watch, learn, play, hide, be happy, be sad, be afraid, dream, hunt, eat, fight, flee, reproduce, educate her kittens – and the list is still very long.
Each of these actions requires processes that are not directly “intelligence” in the most common sense but are related to cognition and animal intelligence. All animals have their own cognition, from the spider that weaves its web to the guide dogs that help people find their way. Some can even communicate with us. Not by speech, of course, but cats and dogs don’t hesitate to use body language and vocalization – meowing, barking, wagging their tails – to get what they want.
Let’s look again at your cat. When she comes carelessly to rub up against you or sits in front of her bowl or in front of a door, the message is quite clear. She is looking for a caress, is hungry or wants to go out (then get in, then out, then in…). She has learned to interact with you to achieve her goals.
Walking, a complex problem
Among all these cognitive skills, there is only a handful that we are beginning to know how to reproduce artificially. For example, bipedal locomotion – walking with two legs. It might be easy and natural to us, but it is actually something extremely complicated for robotics and it took decades of intensive research to build and program a robot that more or less walks properly on its own two legs. That is, without falling because of small pebble under its foot or when a person simply walked by a little too close.
Remember that it takes a baby an average of one full year to learn how to walk, demonstrating the complexity of what may seem like a “simple” problem. And I’m only talking about walking, not hopscotch or, say, soccer.
Today, one of the biggest challenges in autonomous robotics is to design and built two-legged robots that can successfully play one of the most popular human team sports. The Robocup 2020, which brings together nearly 3,500 researchers and 3,000 bipedal robots, will take place next year in Bordeaux, France. There you’ll be able to observe them playing soccer, and while great strides have been made (literally), they remain distinctly clumsy and a long way from the thrills of the human World Cup.
Identifying isn’t understanding
What about object recognition? Today we know how to create computer algorithms that can do that, don’t we? While it is true that some can now name the content of almost any image, this does not relate to intelligence or cognition.
To understand this, you have to look at how these algorithms work. Supervised learning, which remains the most popular method, consists of presenting images and a label describing the content of the image to the program. The total number of images is generally much higher than the number of labels. Each label is associated with a very large number of images representing the object in different situations, under different angles of view, under different lights, etc.
For example, for an AI program to be able to recognize cats, up to one million images must be presented. By doing so, it will build an internal visual representation of the object by calculating a kind of average of all the images. But this representation is ultimately only a simple description that is not anchored in any reality. Humans can recognize a cat from its purr, the feel of its fur against the leg, the delicate scent of a litter box that’s overdue for a cleaning. All these and a hundred more say “cat” to us, but mean nothing to even the most sophisticated AI program.
To do so, an algorithm would need a body that allows it to experience the world. But then, could it understand what a drink is if it’s never thirsty? Could it understand fire if it’s never been burned? Could it understand the cold if it never shudders? When an algorithm “recognizes” an object, it doesn’t understand at all – really, not at all – the nature of that object. It only proceeds by cross-checking with examples previously presented. This explains why there have been a number of autonomous-car crashes. While roadways are a highly constrained form of the world, they remain visually and functionally complex – vulnerable users such as pedestrians and cyclists can too easily be overlooked, or one street element mistaken for another. And the consequences of AI’s shortcomings have sometimes been fatal.
The sensible experience of the world
What about humans? Try the experience of showing a real puppy to a child and she will be able to recognize any other puppy (even if she doesn’t know the word yet). Parents, by designating and naming things, will help the child develop language based on concepts that she has experienced before. But this learning, which may seem easy, even obvious, to us is not.
This is beautifully illustrated by the life of Helen Keller, who lost her hearing, sight and power of speech at the age of two. Her educator, Anne Sullivan, tried for a long time to teach her the words by drawing signs on the palm of Helen’s hand and then touching the corresponding object. Anne Sullivan’s efforts were initially unsuccessful because Helen did not have the entry points for this strange dictionary. Until the day that Anne took Helen to a well, let water run over her hand and…
“Suddenly I felt a misty consciousness as of something forgotten – a thrill of returning thought; and somehow the mystery of language was revealed to me. I knew then that “w-a-t-e-r” meant the wonderful cool something that was flowing over my hand. That living word awakened my soul, gave it light, hope, joy, set it free! … Everything had a name, and each name gave birth to a new thought. As we returned to the house every object which I touched seemed to quiver with life.“
Those are the words of Helen Keller herself. She wrote them a few years later in her book The Story of My Life (1905). For her, on that precise day, the symbols were forever grounded in reality.
While spectacular progress has been made in the field of machine learning, grounding the digital symbols into the real world remains completely unresolved. And without the resolution of this problem, which is necessary but probably not a sufficient, there will be no general artificial intelligence. So there are still a lot of things that we are far from knowing how to do with artificial intelligence. And remember, “elephants don’t play chess.”
This article is republished from The Conversation by Nicolas P. Rougier, Chargé de Recherche en neurosciences computationelles, Université de Bordeaux under a Creative Commons license. Read the original article.
Get the TNW newsletter
Get the most important tech news in your inbox each week.