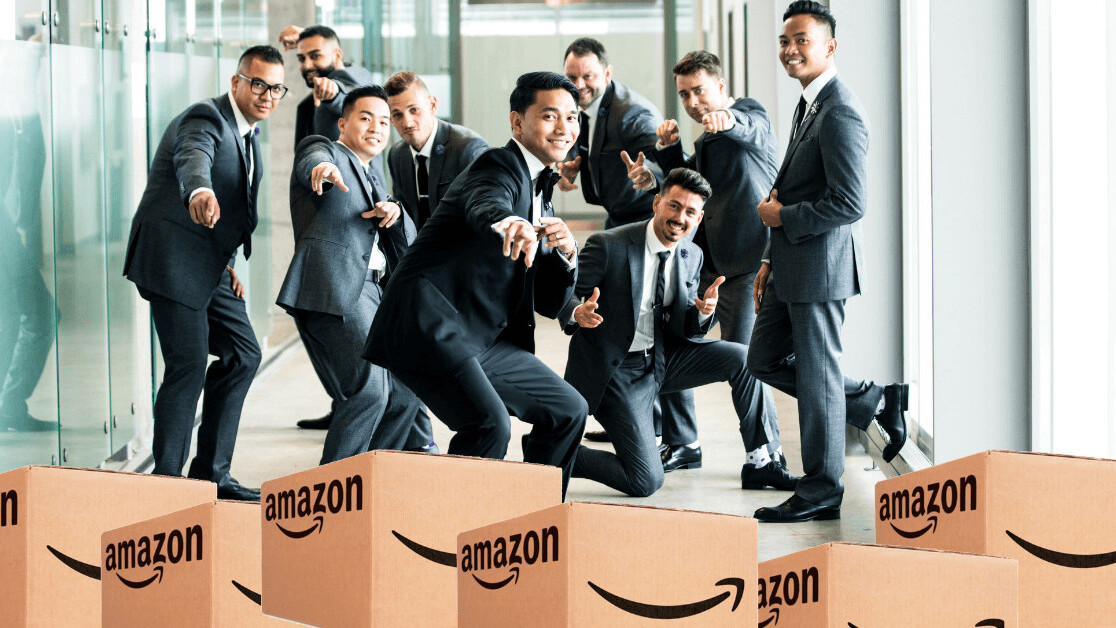
Amazon decided to shut down its experimental artificial intelligence (AI) recruiting tool after discovering it discriminated against women.
The company created the tool to trawl the web and spot potential candidates, rating them from one to five stars. But the algorithm learned to systematically downgrade women’s CV’s for technical jobs such as software developer.
Although Amazon is at the forefront of AI technology, the company couldn’t find a way to make its algorithm gender-neutral. But the company’s failure reminds us that AI develops bias from a variety of sources.
While there’s a common belief that algorithms are supposed to be built without any of the bias or prejudices that color human decision making, the truth is that an algorithm can unintentionally learn bias from a variety of different sources.
Everything from the data used to train it, to the people who are using it, and even seemingly unrelated factors, can all contribute to AI bias.
AI algorithms are trained to observe patterns in large data sets to help predict outcomes. In Amazon’s case, its algorithm used all CVs submitted to the company over a ten-year period to learn how to spot the best candidates.
Given the low proportion of women working in the company, as in most technology companies, the algorithm quickly spotted male dominance and thought it was a factor in success.
Because the algorithm used the results of its own predictions to improve its accuracy, it got stuck in a pattern of sexism against female candidates.
And since the data used to train it was at some point created by humans, it means that the algorithm also inherited undesirable human traits, like bias and discrimination, which have also been a problem in recruitment for years.
Some algorithms are also designed to predict and deliver what users want to see. This is typically seen on social media or in online advertising, where users are shown content or advertisements that an algorithm believes they will interact with. Similar patterns have also been reported in the recruiting industry.
One recruiter reported that while using a professional social network to find candidates, the AI learned to give him results most similar to the profiles he initially engaged with.
As a result, whole groups of potential candidates were systematically removed from the recruitment process entirely.
However, bias also appears for other unrelated reasons. A recent study into how an algorithm delivered ads promoting STEM jobs showed that men were more likely to be shown the ad, not because men were more likely to click on it, but because women are more expensive to advertise to.
Since companies price ads targeting women at a higher rate (women drive 70% to 80% of all consumer purchases), the algorithm chose to deliver ads more to men than to women because it was designed to optimize ad delivery while keeping costs low.
But if an algorithm only reflects patterns in the data we give it, what its users like, and the economic behaviors that occur in its market, isn’t it unfair to blame it for perpetuating our worst attributes?
We automatically expect an algorithm to make decisions without any discrimination when this is rarely the case with humans. Even if an algorithm is biased, it may be an improvement over the current status quo.
To fully benefit from using AI, it’s important to investigate what would happen if we allowed AI to make decisions without human intervention.
A 2018 study explored this scenario with bail decisions using an algorithm trained on historical criminal data to predict the likelihood of criminals re-offending. In one projection, the authors were able to reduce crime rates by 25% while reducing instances of discrimination in jailed inmates.
Yet the gains highlighted in this research would only occur if the algorithm was actually making every decision. This would be unlikely to happen in the real world as judges would probably prefer to choose whether or not to follow the algorithm’s recommendations. Even if an algorithm is well designed, it becomes redundant if people choose not to rely on it.
Many of us already rely on algorithms for many of our daily decisions, from what to watch on Netflix or buy from Amazon. But research shows that people lose confidence in algorithms faster than humans when they see them make a mistake, even when the algorithm performs better overall.
For example, if your GPS suggests you use an alternative route to avoid traffic that ends up taking longer than predicted, you’re likely to stop relying on your GPS in the future.
But if taking the alternate route was your decision, it’s unlikely you will stop trusting your own judgement. A follow-up study on overcoming algorithm aversion even showed that people were more likely to use an algorithm and accept its errors if given the opportunity to modify the algorithm themselves, even if it meant making it perform imperfectly.
While humans might quickly lose trust in flawed algorithms, many of us tend to trust machines more if they have human features. According to research on self-driving cars, humans were more likely to trust the car and believed it would perform better if the vehicle’s augmented system had a name, a specified gender, and a human-sounding voice.
However, if machines become very human-like, but not quite, people often find them creepy, which could affect their trust in them.
Even though we don’t necessarily appreciate the image that algorithms may reflect of our society, it seems that we are still keen to live with them and make them look and act like us. And if that’s the case, surely algorithms can make mistakes too?
This article is republished from The Conversation by Maude Lavanchy, Research Associate, IMD Business School under a Creative Commons license. Read the original article.
Get the TNW newsletter
Get the most important tech news in your inbox each week.