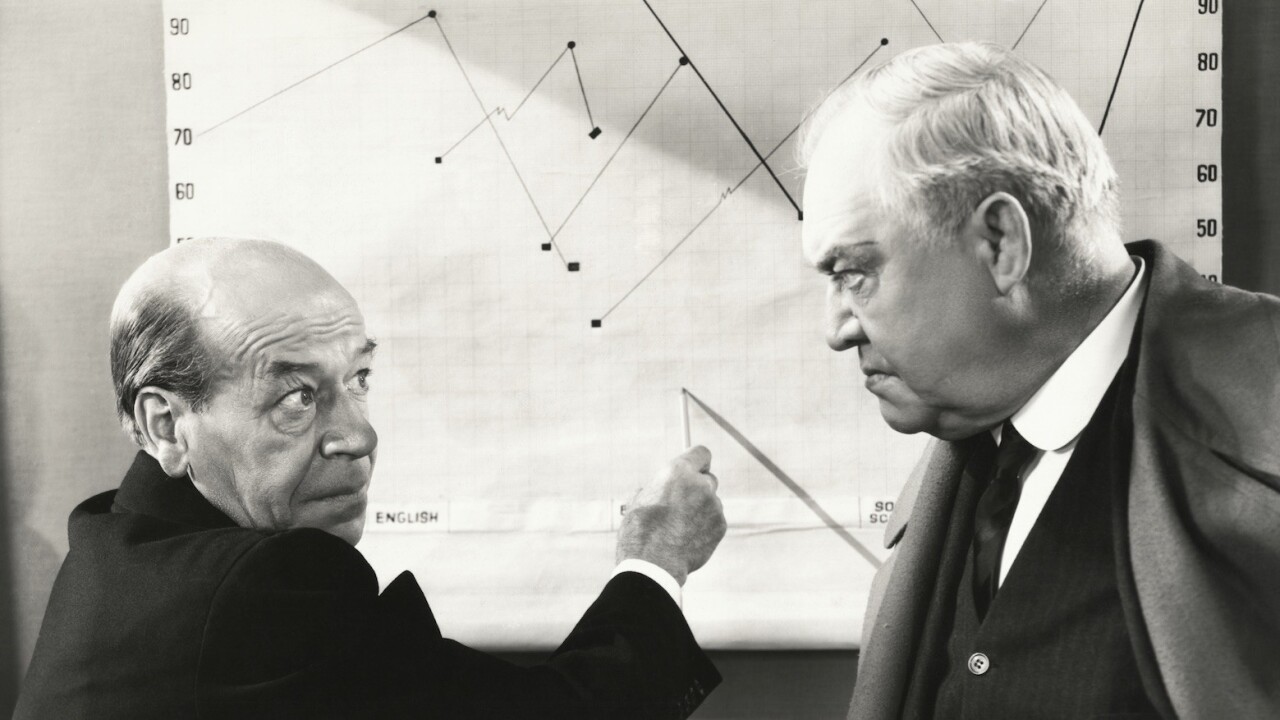
Recent figures estimate that in 2015, the number of “big data” jobs will grow to 4.4 million, with 1.9 million in the United States.
Leaders in these positions will be responsible for building intricate algorithms and crafting stories out of the massive amounts of data that today’s companies are generating. On marketing teams, data scientists will be responsible for connecting the dots between cross-platform audience experiences, optimizing customer lifetime value, building predictive analytics capabilities and guiding expansion into new markets.
According to Accenture’s 2013-2014 CMO Insights survey, 78 percent of CMOs say that analytics, digital media, and mobile technologies will drive major changes within the next five years, but only 21 percent believe that their organizations will be equipped to keep up.
One in four CMOs cite a lack of critical technologies and tools as the chief barrier to digital integration. Marketers need data scientists to help businesses connect the dots between disparate data sources, organizational silos and complex technologies.
But there’s a big problem. There aren’t enough people to fill these multifaceted data science roles that require a rare combination of statistical knowledge, programming chops and storytelling expertise.
“I tend to call the perfect data scientist a ‘unicorn,’” says George Sadler, global leader of CRM and loyalty analytics at eBay. “You’ve heard about them but never really seen one in the wild.”
Why the Term “Data Science” Needs to Change
CMOs can’t find the people they need to navigate today’s data avalanche — and they probably never will. That’s because data science is an all-hands rather than a solo practitioner’s job. As researchers Jeanne G. Harris, Nathan Shetterley, Allan E. Alter, and Krista Schnell point out, data science involves more than just number crunching.
Data science is about about exploring numbers to identify business challenges and identify solutions — initiatives that require cross functional teams of storytellers, programmers, statisticians, designers and accountants. Think about it from the perspective of a marketer — you wouldn’t ask your creative director to build out your marketing automation campaigns.
“Typically data science skillsets come from specialties in academic fields — hard sciences, computer science and business,” says Sadler. “So you have to ‘grow them’ yourself. Often it’s best to mix up among team so they can learn from each other.”
Soon enough, what was once a solo practitioner’s job will evolve to become a cross-functional team. As Harris, Shetterly, Alter, and Schnell put it: “When physicists take on a big project, they bring together a team to design the equipment, run experiments and analyze the data. Likewise, it makes sense to divide the labor of a data scientist rather than search for one person who can do it all.”
The Role of Higher Ed
Rather than preparing students for today’s data science roles, higher ed must cultivate skills for cross-functional teams. Teach students to become expert programmers, designers and storytellers — with statistics as the common thread that connects these seemingly disparate disciplines. Here is how universities can lead this change:
1 – Teach ‘”data science” from multiple perspectives
Data scientists frequently come from computer science and engineering backgrounds. These graduates are highly skilled programmers who become expert developers and technologists. But engineering is only part of the data science equation.
To be impactful, data science projects need to tell an overarching business story. At the end of the day, metrics are tools that help organizations solve problems — which is why every organization is going to need a team of data storytellers.
These roles, while grounded in technology, will require little to no programming experience. They will, however, require proficiency with statistics, communication with developers and writing about data.
Without even realizing it, schools are already preparing this next generation of storytellers. The Demographics and Social Analysis (DASA) master’s program at the University of California at Irvine, for instance, teaches concepts in machine learning and statistical modeling from the perspective of social science.
DASA students can take courses related to the analysis of social network data, multivariate analysis and geographic information systems (GIS) to gain proficiency in working with and writing about data. Graduates of this program are unlikely to be developers — but they’ll be strong complements to teams where data engineers are present.
2 – Teach the greater context
With data science comes non-technical questions related to privacy, compliance and ethics. Because the field is so new, “best practices” are yet to be defined — it’s the next generation of data scientists and marketers that will be designating standards. These leaders will need a clear understanding of how their actions are affecting the world around them.
The University of Virginia’s department of systems and information engineering is tackling this challenge head-on. Last winter, a $10 million gift established an endowment to support the university’s new Data Science Institute. The institute has established a cross-functional faculty team to tackle society’s most pressing “big data” challenges from multiple perspectives including the humanities, engineering and sciences.
“Our program takes students through the major computational and analytic topics in data science as well as the ethical, legal and policy issues involved,” says the institute’s founding director Don Brown.
UVa has built its center to serve as the blueprint for scientific and engineering research in which data science plays a role. Ethics, law and policy are a key part of the center’s educational vision.
3 – Teach comfort with risk and ambiguity
With data science comes risk, uncertainty, and ambiguity. Business analysts and marketing teams are navigating completely new terrain. Success, to an extent, will come from throwing darts in the dark.
Universities can prepare grads by helping them become comfortable with uncertain situations, in which answers will be challenging to derive — if there are answers at all. It’s important to expose students to messy data sets with less-than-intuitive paths forward because, in the real-world, data will never take the form of a neatly packaged “problem set.”
Part of this process should require students to work in teams — to draw from the diversity of skills and perspectives that each person brings to the table. It’s this approach that will help students become cross-functional collaborators.
“An important part of an effective learning experience is feeling perplexed and not knowing what to do,” explains Michel Ballings, assistant professor of business analytics and statistics at The University of Tennessee.
“Therefore it is important to work in groups. Trying to solve the many problems that will be encountered in doing such projects will help students learn a lot more than when I simply give them all the solutions. Being able to handle highly ambiguous tasks like data mining and work in teams in a very valuable skill.”
Ballings encourages universities to design their programs, from the ground up, with the evolving needs of the marketing industry. He encourages data science programs to team up with real-world practitioners.
“Build close relationships with industry,” he recommends. “Provide industry leaders a venue for coming to campus and interacting with students. Provide students lots of hand-on industry experience via class projects, capstone projects, seminars with industry speakers and summer internships.”
He encourages professors to continue their education as well.
“Provide faculty opportunities to work with industry in order to develop their experience, and hire adjunct faculty having industry experience,” Ballings says.
Read Next: How to hire data scientists
Image credit: Shutterstock
This post first appeared on dnb.com
Get the TNW newsletter
Get the most important tech news in your inbox each week.