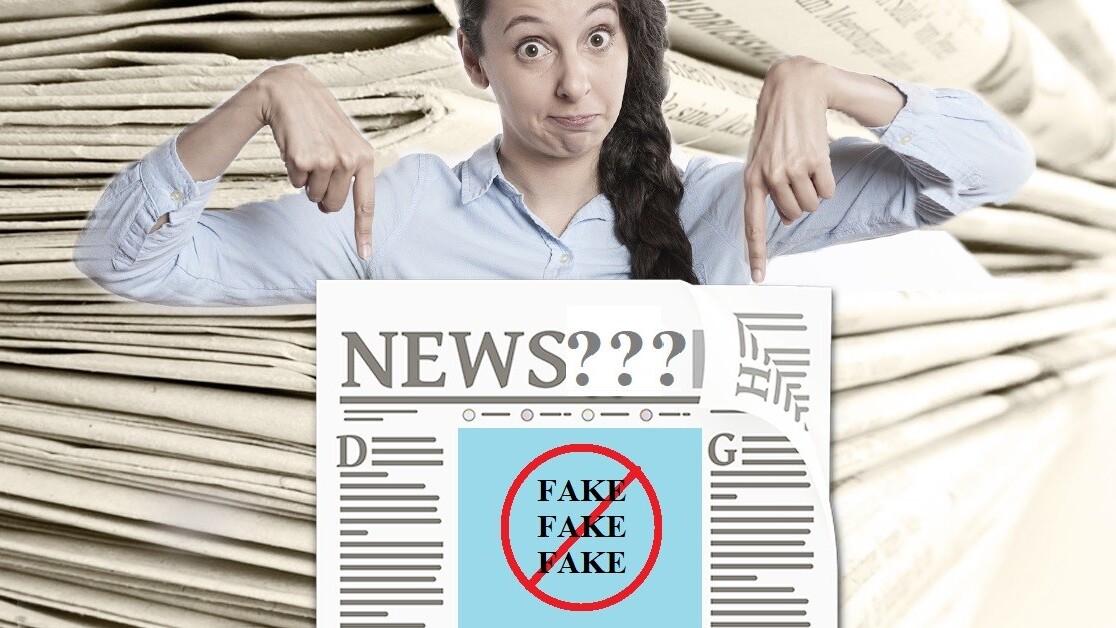
When researchers working on developing a machine learning-based tool for detecting fake news realized there wasn’t enough data to train their algorithms, they did the only rational thing: They crowd-sourced hundreds of bullshit news articles and fed them to the machine.
The algorithm, which was developed by researchers from the University of Michigan and the University of Amsterdam, uses natural language processing (NLP) to search for specific patterns or linguistic cues that indicate a particular article is fake news. This is different from a fact checking algorithm that cross-references an article with other pieces to see if it contains inconsistent information – this machine learning solution could automate the detection process entirely.
But that’s not the cool part. No offense to the Michigan/Amsterdam team but building an NLP algorithm to parse sentence structure and hone in on keywords isn’t exactly the bleeding edge artificial intelligence work that drops jaws. Getting it to detect fake news better than people, however, is.
The problem with that is there just isn’t enough data. You can’t just download the internet and tell an algorithm to figure things out: Machines need rules and examples. The commonly available datasets for this type of training include one called the Buzzfeed dataset, which was used to train an algorithm to detect hyperpartisan fake news on Facebook for a Buzzfeed article. Other datasets focus mainly on training an AI against satire, such as The Onion – unfortunately this method tends to just make an algorithm a satire detector.
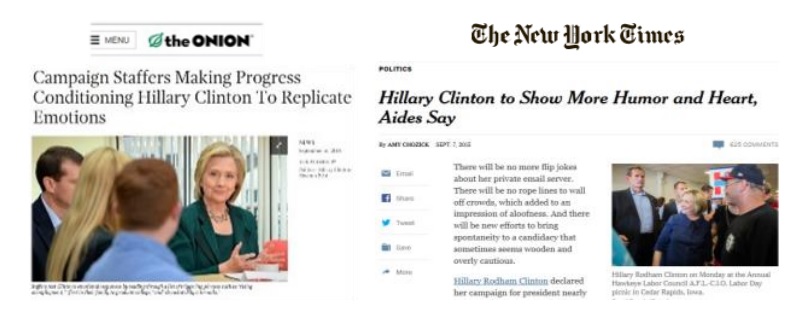
So, first things being first, the researchers had to decide what fake news is. For this, they turned to the “requirements for a fake news corpus” developed by a team of researchers from the University of Western Ontario.
These nine requirements basically indicate that an algorithm to detect fake news needs to be capable of detecting non-fake news, verifying the ground-truth, and accounting for factors such as developing news and language and cultural interpretations. You can read more about it in the Ontario team’s white paper here.
The bottom line is that fake news, despite what the people you’re arguing with on Twitter might claim, is reporting that is intentionally false (called serious fabrication), hoaxes (created for the intent of going viral on social media) or articles intended as humor or satire.
To be clear, no scientists, that we know of, are working on a way to get CNN or Fox News to cover the stories that you want them to, or to cover them the way you think they should. That’s not a fake news problem — unless a particular article also falls into one of the aforementioned categories.
The researchers crowd-sourced their dataset by asking Amazon Mechanical Turk workers to reinterpret 500 real news stories as fake news stories. Participants in the study were asked to imitate the journalistic style of the original article, but fudge the facts and information enough to ensure the result was demonstrably fake.
The team fed both the fake news and the real news to the algorithm and it taught itself how to distinguish between the two. Once it was trained, the team fed it news straight from a dataset containing both real and fake news directly from the web and it did better than humans at figuring out which was which.
It’s not perfect, it gets things wrong about 24 percent of the time, but the humans messed things up 30 percent of the time, so the point goes to the machines.
One of the researchers on the project, Rada Mihalcea, told Michigan News:
You can imagine any number of applications for this on the front or back end of a news or social media site. It could provide users with an estimate of the trustworthiness of individual stories or a whole news site. Or it could be a first line of defense on the back end of a news site, flagging suspicious stories for further review. A 76 percent success rate leaves a fairly large margin of error, but it can still provide valuable insight when it’s used alongside humans.
The global community is in the midst of what future historians will almost certainly refer to as the “fake news era.” It’s likely this problem won’t be solved by simply relying on writers to never lie and news consumers to always verify everything they read with multiple sources.
A more elegant solution involves the implementation of protocols and protections at the publishing, aggregation, and platform level – and until that’s completely automated we’ll continue to see the stream of fake news from disreputable outlets flow unchecked.
At least, according to the research team’s white paper, we’ve reached a performance plateau where the best performing computer models are “comparable to human ability to spot fake content.” We’re not sure if that’s good enough to eliminate the problem entirely, but it’s a great start.
Get the TNW newsletter
Get the most important tech news in your inbox each week.