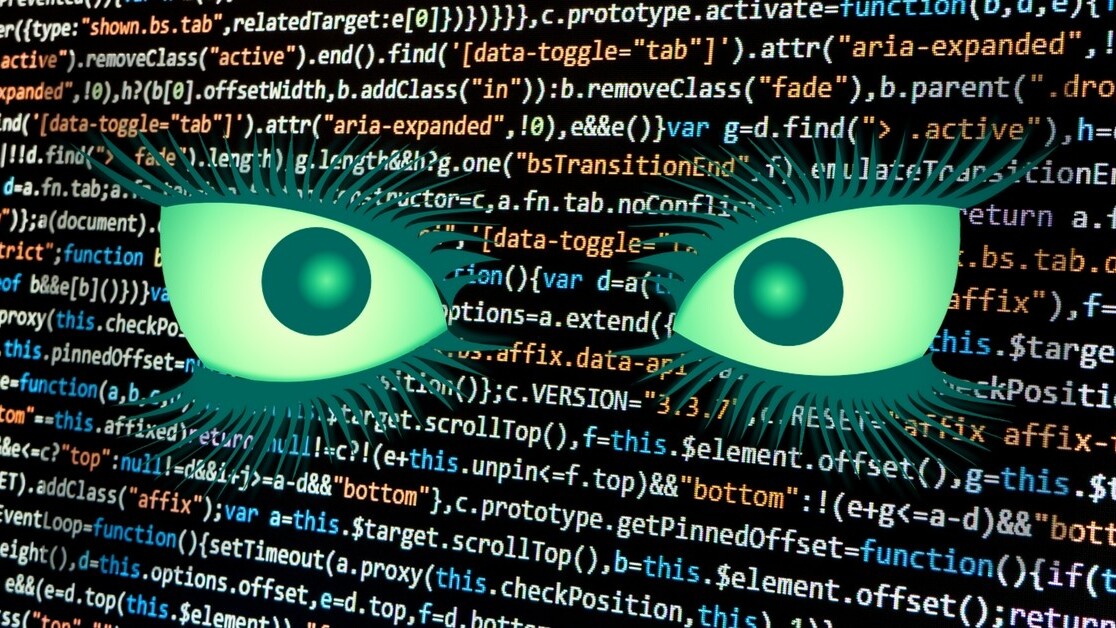
In early 2016, Microsoft launched Tay, an AI chatbot that was supposed to mimic the behavior of a curious teenage girl and engage in smart discussions with Twitter users. The project would display the promises and potential of AI-powered conversational interfaces.
However, in less than 24 hours, the innocent Tay became a racist, misogynist and a holocaust denying AI, debunking—once again—the myth of algorithmic neutrality. For years, we’ve thought that artificial intelligence doesn’t suffer from the prejudices and biases of its human creators because it’s driven by pure, hard, mathematical logic.
However, as Tay and several other stories have shown, AI might manifest the same biases as humans, and in some cases, it might even be worse. The phenomenon, known as “algorithmic bias,” is rooted in the way AI algorithms work and is becoming more problematic as software becomes more and more prominent in every decision we make.
The roots of algorithmic bias
Machine learning and deep learning, the most popular branches of AI, are the reason our software become biased. Deep learning algorithms are dependent on data, lots of it. Give an image classification algorithm millions of labeled cat pictures and it will be able to tell you whether a photo it hasn’t seen before contains a cat. Give a speech recognition algorithm millions of voice samples along with their corresponding written words, and it will be able to transcribe spoken language faster than most humans.
The more labeled data an algorithm sees, the better it becomes at the task it performs. However, the tradeoff to this approach is that deep learning algorithms will develop blind spots based on what is missing or is too abundant in the data they’re trained on.
For instance, in 2015, Google’s photos app mistakenly tagged a photo of two black people as gorillas because its algorithm hadn’t been trained with enough images of dark-skinned persons. In another case, the AI judge of a beauty contest mostly chose white participants as winners because its training was done on images of white people.
These are trivial cases that can be easily remedied by providing the AI with more samples in areas where it doesn’t have enough data. In other cases where AI is working with vast amounts existing data in the endless sea of online information, finding and countering bias becomes much more difficult.
An example is a joint project by researchers at Microsoft and Boston University, in which they found sexist biases in word embedding algorithms, which are used in search engines, translation and other software that depend on natural language processing.
Among their findings of the behavior of word embedding algorithms was a tendency to associate words such as “programming” and “engineering” to men and “homemaker” to women. In this case, the bias was ingrained in the thousands of articles the algorithms had automatically scavenged and analyzed form online sources such as Google News and Wikipedia.
For instance, the tech industry is mostly dominated by men. This means that you’re more likely to see male names and pronouns appear next to engineering and executive tech jobs. As humans, we acknowledge this as a social problem that we need to address. But a mindless algorithm analyzing the data would conclude that tech jobs should belong to men and wouldn’t see it as a lack of diversity in the industry.
In the case of Tay, the Twitter users who interacted with the chatbot were more interested in teaching it hateful speech than engaging in meaningful conversations. Again, the AI was not to blame. The culprit was the general culture that Twitter as a social media breeds.
Why is algorithmic bias a serious problem?
Algorithmic bias is not new. Academics and experts have been warning about it for years. However, what makes it especially critical at this time is the prominence algorithms are finding in everyday decisions we make.
Take the word embedding algorithm problem we visited in the previous section. This can be the kind of technology that powers the next generation of recruitment software. It’s not hard to imagine that software discriminating against women when searching for and selecting candidates for a programming job.
For instance, separate reports recently showed that both Google’s and LinkedIn’s platforms were showing high-paying job ads more frequently to men than women.
Algorithmic bias can have even more damaging effect in other areas such as law enforcement. In 2016, a ProPublica investigation found that an AI-powered tool used by law enforcement was more likely to declare black people as under the high risk of recidivism than white people. In some states, judges rely on such tools to decide who stays in jail and who walks free, sometimes without doing further investigation themselves.
Similar cases can happen in other areas such as loan approval, where people who are underrepresented will be further marginalized and deprived of service. In healthcare, where AI is making great inroads in diagnosing and curing diseases, algorithms can harm populations whose data has not been included in the training sets.
In fact, if not addressed, algorithmic bias can lead to the amplification of human biases.
Under the illusion that software isn’t biased, humans tend to trust the judgment of AI algorithms, oblivious that those judgments are already reflecting their own prejudices. As a result, we will accept AI-driven decisions without doubting them and create more biased data for those algorithms to further “enhance” themselves on.
How to fight algorithmic bias?
The first step to avoiding algorithmic bias is to acknowledge the limits of artificial intelligence. Deep learning algorithms are not racist, but we are, and they will pick up whatever biases we intentionally or absentmindedly have.
Knowing this, we need to take measures to make sure the data we feed to our algorithms is diversified, especially when developing applications that make decisions that can have severe repercussions on the lives and health of the people who directly or indirectly use them. There are a handful of efforts that use statistical methods to spot hidden biases in algorithms.
Another necessary step is for companies that develop AI applications to be more transparent about their products. Presently, most companies tend to hide their algorithms’ inner workings as trade secrets. This makes it difficult to scrutinize those algorithms and find potential pain points.
We also need to address AI’s black box problem. When deep learning algorithms become too complicated, finding the reasons behind their decisions becomes very difficult. Not knowing how an algorithm reached a conclusion can make it hard to find and counter biased functionality. This too is an area where several organizations, including the U.S. Defense Department’s Advanced Research Projects Agency (DARPA), are leading efforts to make deep learning algorithms open to scrutiny or self-explainable.
At the end of the day, algorithmic bias is a human problem, not a technical one, and the real solution is to start removing bias in every aspect of our personal and social lives. This means endorsing diversity in employment, education, politics and more. If we want to fix our algorithms, we should start by fixing ourselves.
This story is republished from TechTalks, the blog that explores how technology is solving problems… and creating new ones. Like them on Facebook here and follow them down here:
Get the TNW newsletter
Get the most important tech news in your inbox each week.