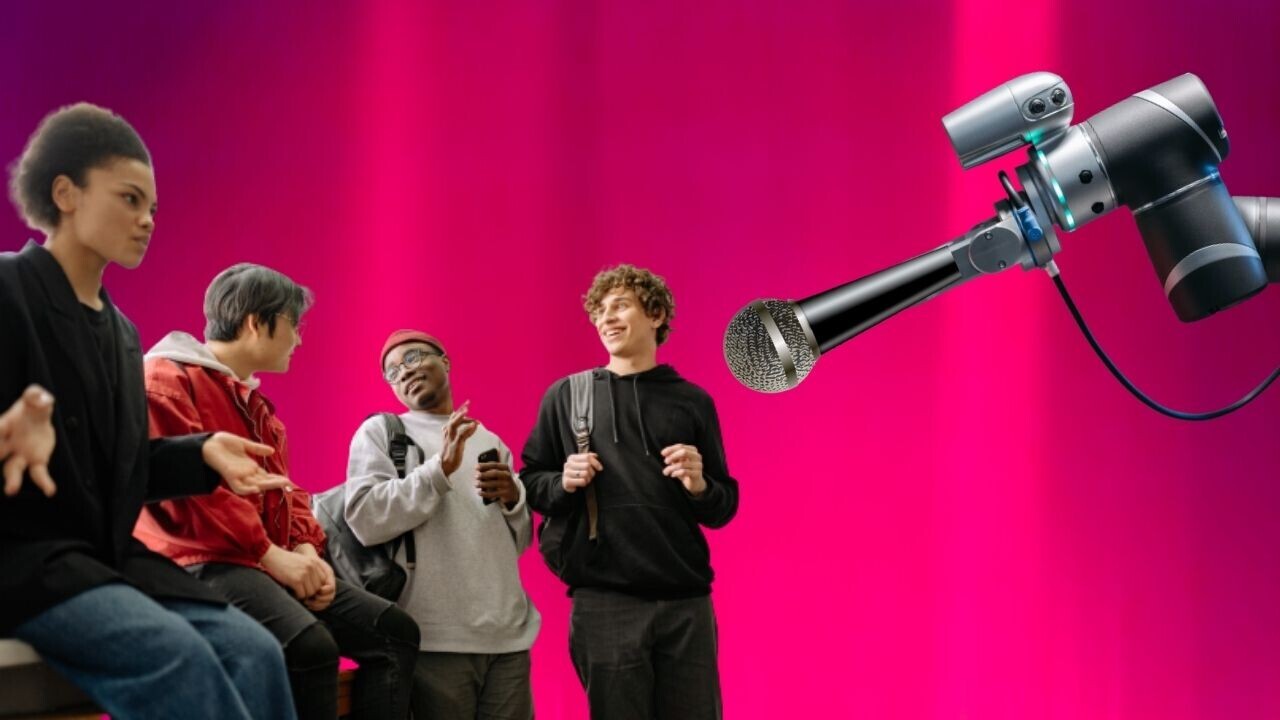
Speech recognition systems struggle to understand African American Vernacular English (AAVE). In a 2020 study by Stanford University researchers, the software performed so poorly for AAVE that some leading systems made correct transcriptions for barely half the words spoken.
The researchers speculated that the systems had a common flaw: “insufficient audio data from Black speakers when training the models.”
A startup called Speechmatics has developed a technique that appears to reduce this data gap.
The company announced last week that its software had “an overall accuracy of 82.8% for African American voices” based on datasets used in the Stanford study. In comparison, the systems developed by Google and Amazon both recorded an accuracy of only 68.6%.
Speechmatics attributed much of its performance to a technique called self-supervised learning.
Training school
The advantage of self-supervised models is that they don’t require all their training data to be labeled by humans. As a result, they can enable AI systems to learn from a much larger pool of information.
This helped Speechmatics increase its training data from around 30,000 hours of audio to around 1.1 million hours.
Will Williams, the company’s VP of machine learning, told TNW that the approach improved the software’s performance across a variety of speech patterns:
What we’re looking to do is build scalable methods that let us attack a broad range of accents at once.
Learning like a child
One of the technique’s benefits was closing Speechmatics’ age understanding gap.
Based on the open-source project Common Voice, the software had a 92% accuracy rate on children’s voices. The Google system, by comparison, had an accuracy of 83.4%.
Williams said enhancing the recognition of kids’ voices was never a specific objective:
We’re training on millions of hours of audio, and just like how a child learns, we’re exposing our learning systems to all this online audio… Inside those millions of hours, there will be children’s voices, so it will learn how to deal with them — but without them being labelled.
That doesn’t mean that self-supervised learning alone can eliminate AI biases. Allison Koenecke, the lead author of the Stanford study, noted that other issues also need to be addressed:
We also strongly believe that achieving fair outcomes is as much a ‘people problem’ as a ‘data problem.’ That is, we hope that ASR [automatic speech recognition] developers themselves understand the need to be broadly inclusive.
Get the TNW newsletter
Get the most important tech news in your inbox each week.