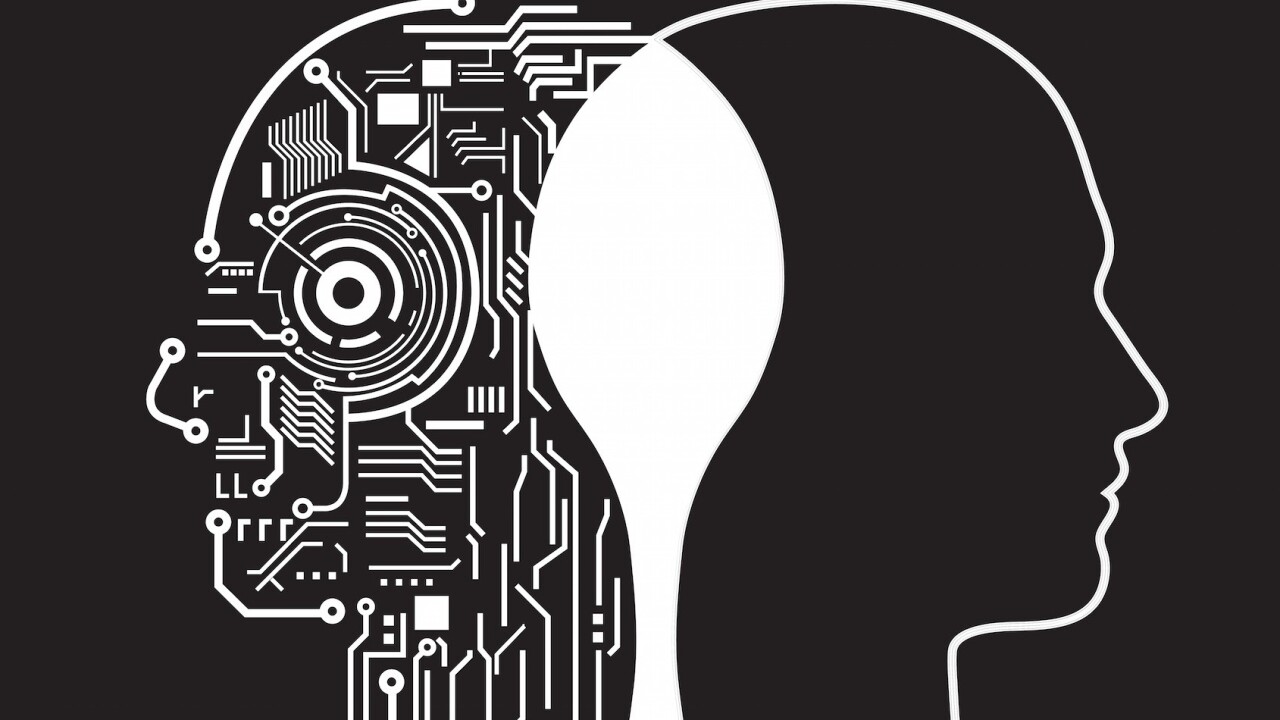
It seems that the more ground-breaking deep learning models are in AI, the more massive they get. This summer’s most buzzed-about model for natural language processing, GPT-3, is a perfect example. To reach the levels of accuracy and speed to write like a human, the model needed 175 billion parameters, 350 GB of memory and $12 million to train (think of training as the “learning” phase). But, beyond cost alone, big AI models like this have a big energy problem.
UMass Amherst researchers found that the computing power needed to train a large AI model can produce over 600,000 pounds of CO2 emissions – that’s five times the amount of the typical car over its lifespan! These models often take even more energy to process in real-world production settings (otherwise known as the inference phase). NVIDIA estimates that 80-90 percent of the cost incurred from running a neural network model comes during inference, rather than training.
To make more progress in the AI field, popular opinion suggests we’ll have to make a huge environmental tradeoff. But that’s not the case. Big models can be shrunk down to size to run on an everyday workstation or server, without having to sacrifice accuracy and speed. But first, let’s look at why machine learning models got so big in the first place.
Now: Computing power doubling every 3.4 months
A little over a decade ago, researchers at Stanford University discovered that the processors used to power the complex graphics in video games, called GPUs, could be used for deep learning models. This discovery led to a race to create more and more powerful dedicated hardware for deep learning applications. In turn, the models data scientists created became bigger and bigger. The logic was that bigger models would lead to more accurate outcomes. The more powerful the hardware, the faster these models would run.
Research from OpenAI proves that this assumption has been widely adopted in the field. Between 2012 and 2018, computing power for deep learning models doubled every 3.4 months. So, that means in a six year time period, the computing power used for AI grew a shocking 300,000x. As referenced above, this power is not just for training algorithms, but also to use them in production settings. More recent research from MIT suggests that we may reach the upper limits of computing power sooner than we think.
What’s more, resource constraints have kept the use of deep learning algorithms limited to those who can afford it. When deep learning can be applied to everything from detecting cancerous cells in medical imaging to stopping hate speech online, we can’t afford to limit access. Then again, we can’t afford the environmental consequences of proceeding with infinitely bigger, more power-hungry models.
The future is getting small
Luckily, researchers have found a number of new ways to shrink deep learning models and repurpose training datasets via smarter algorithms. That way, big models can run in production settings with less power, and still achieve the desired results based on the use case.
These techniques have the potential to democratize machine learning for more organizations who don’t have millions of dollars to invest in training algorithms and moving them into production. This is especially important for “edge” use cases, where larger, specialized AI hardware is not physically practical. Think tiny devices like cameras, car dashboards, smartphones, and more.
Researchers are shrinking models by removing some of the unneeded connections in neural networks (pruning), or by making some of their mathematical operations less complex to process (quantization). These smaller, faster models can run anywhere at similar accuracy and performance to their larger counterparts. That means we’ll no longer need to race to the top of computing power, causing even more environmental damage. Making big models smaller and more efficient is the future of deep learning.
Another major issue is training big models over and over again on new datasets for different use cases. A technique called transfer learning can help prevent this problem. Transfer learning uses pretrained models as a starting point. The model’s knowledge can be “transferred” to a new task using a limited dataset, without having to retrain the original model from scratch. This is a crucial step toward cutting down on the computing power, energy and money required to train new models.
The bottom line? Models can (and should) be shrunk whenever possible to use less computing power. And knowledge can be recycled and reused instead of starting the deep learning training process from scratch. Ultimately, finding ways to reduce model size and related computing power (without sacrificing performance or accuracy) will be the next great unlock for deep learning. That way, anyone will be able to run these applications in production at lower cost, without having to make a massive environmental tradeoff. Anything is possible when we think small about big AI – even the next application to help stop the devastating effects of climate change.
Get the TNW newsletter
Get the most important tech news in your inbox each week.