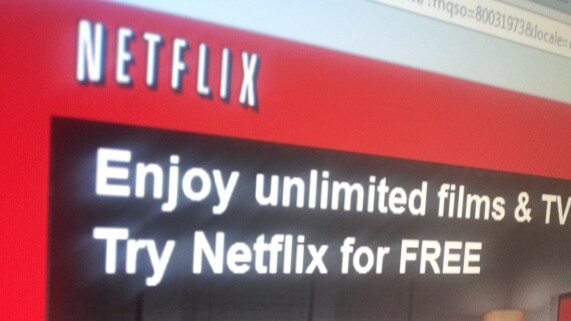
Netflix was founded in the US in 1997, starting initially as a DVD-rental delivery service before finally morphing into more of a VoD streaming service. Today, it serves the US and Canada, Latin America, the Caribbean, and, as of January this year, the UK and Ireland, where it finally arrived to exchange blows with LoveFilm.
Cast your mind back to when the company launched the Netflix Prize. Announced in 2006, the prize sought to reward clever people for developing a recommendation algorithm. The company offered $1 million to whoever could improve the accuracy of its existing system, Cinematch, by 10%.
On September 21, 2009 the mammoth prize was given to team BellKor’s Pragmatic Chaos, who produced an algorithm which apparently improved the search by 10.06%.
Whatever happened to that $1m algorithm?
As pointed out by TechDirt’s Mike Masnick, last week Netflix launched a two-part blog post on its recommendations system, one of which was interesting if only for what it revealed about the outcome of this supposed winning formula which it coughed up big bucks for. Indeed, by the time the algorithm was good to go, Netflix as a business had moved on.
Whilst they acknowledge that the work that went into the final product was immense, Xavier Amatriain and Justin Basilico, Personalization Science and Engineering at Netflix, say that the engineering effort required to achieve the accuracy gains they measured, wasn’t entirely justified.
“This is a truly impressive compilation and culmination of years of work, blending hundreds of predictive models to finally cross the finish line,” they say. “We evaluated some of the new methods offline but the additional accuracy gains that we measured did not seem to justify the engineering effort needed to bring them into a production environment. Also, our focus on improving Netflix personalization had shifted to the next level by then.”
Basically, streaming changed the way its members interacted with Netflix, as well as the type of data available in its algorithms. “For DVDs our goal is to help people fill their queue with titles to receive in the mail over the coming days and weeks; selection is distant in time from viewing, people select carefully because exchanging a DVD for another takes more than a day, and we get no feedback during viewing,” they say. “For streaming members who are looking for something great to watch right now; they can sample a few videos before settling on one, they can consume several in one session, and we can observe viewing statistics such as whether a video was watched fully or only partially.”
But this is an interesting point, in that it highlights the differences between ‘watch now’ and ‘watch later’ behaviours. As TechDirt’s Masnick says:
“I also find it interesting that there’s a clear distinction in the kinds of recommendations people that work if people are going to ‘watch now’ vs. ‘watch in the future’.
I think this is an issue that Netflix probably has faced on the DVD side for years: when people rent a movie that won’t arrive for a few days, they’re making a bet on what they want at some future point. And, people tend to have a more… optimistic viewpoint of their future selves. That is, they may be willing to rent, say, an “artsy” movie that won’t show up for a few days, feeling that they’ll be in the mood to watch it a few days (weeks?) in the future, knowing they’re not in the mood immediately.
But when the choice is immediate, they deal with their present selves, and that choice can be quite different. It would be great if Netflix revealed a bit more about those differences, but it is already interesting to see that the shift from delayed gratification to instant gratification clearly makes a difference in the kinds of recommendations that work for people.”
Netflix also says that another big change to come about was the move from a single platform onto hundreds of devices, for example when it integrated with Roku and Xbox in 2008, which was already a couple of years into the competition. Then Netflix streaming made it to the iPhone, before hitting Android and range of other connected devices. In short, a one-algorithm approach clearly won’t work across such a wide range of platforms.
It’s also worth noting the global availability of Netflix. It’s now extended beyond the US and in to Canada, 43 Latin-American countries plus the UK and Ireland. It has 23 million subscribers in 47 countries, who streamed 2 billion hours from multiple devices in Q4 2011 alone. Every day 2 million movies and TV shows are queued, and 4 million ratings are generated. So how does personalization cope with such a massive multifaceted jump in usage?
“We have adapted our personalization algorithms to this new scenario in such a way that now 75% of what people watch is from some sort of recommendation,” says Netflix. “We reached this point by continuously optimizing the member experience and have measured significant gains in member satisfaction whenever we improved the personalization for our members.”
Well, $1m may sound like a lot of money for Netflix to pay for something that wasn’t really used, but we’re sure Bob Bell, Martin Chabbert, Michael Jahrer, Yehuda Koren, Martin Piotte, Andreas Töscher and Chris Volinsky from BellKor’s Pragmatic Chaos don’t mind too much.
Get the TNW newsletter
Get the most important tech news in your inbox each week.