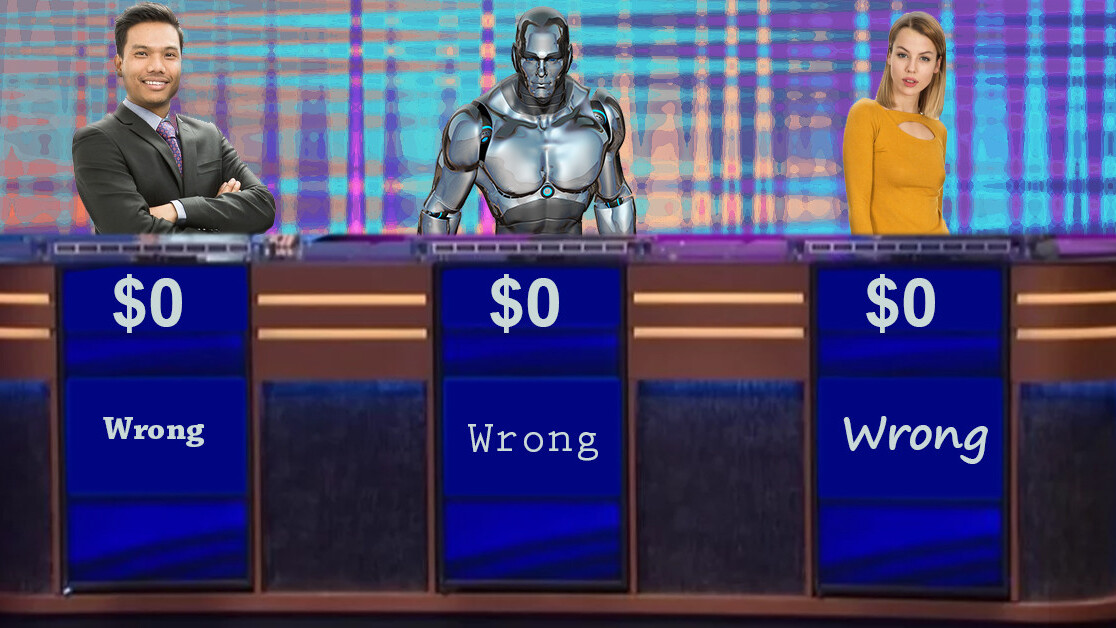
The cold, calculating ‘mind’ of a machine has no capacity for emotion. Alexa doesn’t care if you call it names. DeepMind’s AlphaGo will never actually taste the sweet joy of victory. Despite this, they’re more like humans than you might think. They’re nearly always wrong and virtually incapable of being rational.
British statistician George Box famously stated “all models are wrong, but some are useful” in a research paper published in 1976. While he was referring to statistical models, it’s well-accepted that the aphorism applies to computer models as well, and thus it makes sense from an AI point of view.
The reason why all models are wrong is simple: they’re based on limited information. Human perception through our five senses isn’t powerful enough to pick up on all available data in a given situation. Worse, our brains couldn’t process all the available information even if we were able to gather it.
Tshilidzi Marwala, Vice Chancellor at the University of Johannesburg, recently published a research paper discussing the possibility of rational AI. He explains the problem:
AI models are not physically realistic. They take observed data and fit sophisticated yet physically unrealistic models. Because of this reality they are black boxes with no direct physical meaning. Because of this reason they are definitely wrong yet are useful.
If in our AI equation y=f(x), f the model is wrong as stipulated by Box then despite the fact that this model is useful in the sense that it can reproduce reality, it is wrong. Because it is wrong, when used to make a decision such a decision cannot possibly be rational. A wrong premise cannot result in a rational outcome! In fact the more wrong the model is the less rational the decision is.
Imagine falling out of an airplane and plummeting 15,000 feet without a parachute. You’re simply not going to be capable of understanding the gazillions (not a technical measurement) of tiny details – like air speed, or a million adjustments-per-second to optimize trajectory, or whatever – necessary to ensure your survival.
But, a bird’s brain understands the nuances of air currents in ways humans cannot. Granted, they have hollow bones and wings. But even without the physical advantages they have a better mind for flight than our advanced human brain. So, theoretically, you’d be better off with a tiny bird brain than your big old human mind, in this particular scenario.
Still, birds aren’t rational. Just like humans they’re trying to avoid making fatal mistakes, not optimize their systems for maximum utility.
The point is, no matter how advanced a system becomes, if it operates on the same principles as the human brain (or any other organic mind), it’s flawed.
The human brain is a wrong-engine, because it’s more useful to apply Occam’s Razor (ie., reduce the potential solutions to either fight or flight) than it is to parse a slightly less-limited set of variables.
AI, currently, isn’t any different. It has to either be fed information (thus limiting its access) or be taught how to find information for itself (thus limiting its parameters for selecting relevant data). Both scenarios make AI as much of a ‘wrong-engine’ as the human brain.
Of course, the only solution is to build rational AI right? Not according to Marwala. His research didn’t have a happy ending:
This paper studied the question of whether machines can be rational. It examined the limitations of machine decision making and these were identified as the lack of complete and perfect information, the imperfection of the models as well as the inability to identify the global optimum utility. This paper concludes that machines can never be fully rational and that the best they can achieve is to be bounded rationally. However, machines can be more rational than humans.
Marwala believes that, with the exception of a few convex problems, we’ll never have unbounded rationalism — in people or in machines — because it’s impossible to know if a given decision is globally optimized or not.
Whether he’s correct or just hasn’t met the solution yet, an interesting byproduct of his thinking is that artificial general intelligence (AGI) isn’t very important in the grand scheme of things, unless he’s right. If he’s right, then AGI is the ultimate goal of machine learning.
We’ll need machines that can imitate or beat human-level general intelligence to arrive as quickly as possible so that we can then spend the rest of our species’ existence tweaking the formula.
But, if he’s wrong: AGI is a MacGuffin. It’s a means to get people working on a problem they can’t attack just yet: rational AI.
And if you think the idea of sentient robots is a radical one, try wrapping your head around one that’s borderline omniscient. A machine capable of unbounded-rationality would, by definition, be a near-perfect decision-making machine.
What do you think? Is rational AI achievable or will our future overlords need to evolve like their creators?
Get the TNW newsletter
Get the most important tech news in your inbox each week.