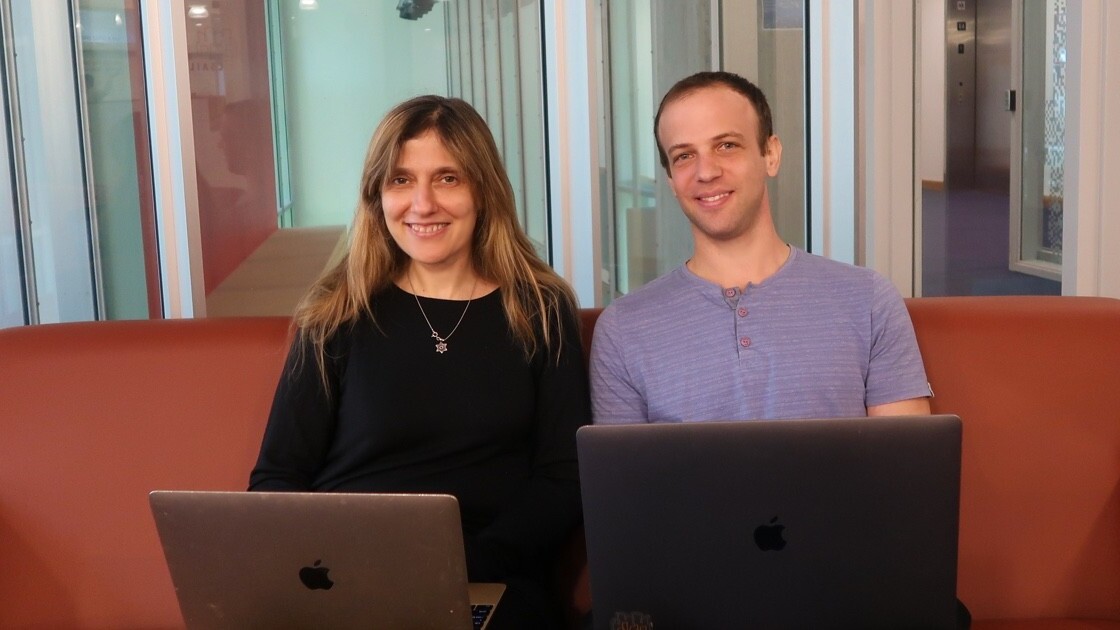
Bad actors are increasingly using more advanced methods to generate fake news and fool readers into thinking they are legitimate. AI-based text generators, including OpenAI’s GPT-2 model, which try and imitate human writers play a big part in this.
To mitigate this, researchers have developed tools to detect artificially generated text. However, new research from MIT suggests there might be a fundamental flaw in the way these detectors work.
Traditionally, these tools trace back a text’s writing style to determine if it’s written by humans or a bot. They assume text written by humans is always legitimate and the text generated by bots is always fake. That means if even if a machine can generate legitimate text for some uses cases, it is deemed fake by these models.
Plus, the research highlights attackers can use tools to manipulate human-generated text. Researchers trained AI to use a using GPT-2 model to corrupt human-generated text to alter its meaning.
Tal Schuster, an MIT student and lead author on the research, said it’s important to detect factual falseness of a text rather than determining if it was generated by a machine or a human:
We need to have the mindset that the most intrinsic ‘fake news’ characteristic is factual falseness, not whether or not the text was generated by machines. Text generators don’t have a specific agenda – it’s up to the user to decide how to use this technology.
MIT professor Regina Barzilay said this research highlighted the lack of credibility of current misinformation classifiers.
To overcome these flaws, the same set of researchers used the world’s largest fact-checking database, Fact Extraction, and Verification (FEVER), to develop new detection systems.
However, the research team found the model developed through FEVER was prone to errors due to the datasets’ bias.
Schuster said negated phrases were often deemed to be false by the model:
Many of the statements created by human annotators contain give-away phrases. For example, phrases like ‘did not’ and ‘yet to’ appear mostly in false statements.
However, when the team created a data set by debiasing FEVER, the detection model’s accuracy fell from 86 to 58 percent showing there’s more work to be done to train AI on non-biased data.
He said the model had taken the language of the claim into account without any external evidence. So, there’s a chance a detector can deem a future event false because it hasn’t used external sources as part of its verification process.
The team hopes to improve the model to detect new types of misinformation by combining fact-checking with existing defense mechanisms.
Get the TNW newsletter
Get the most important tech news in your inbox each week.