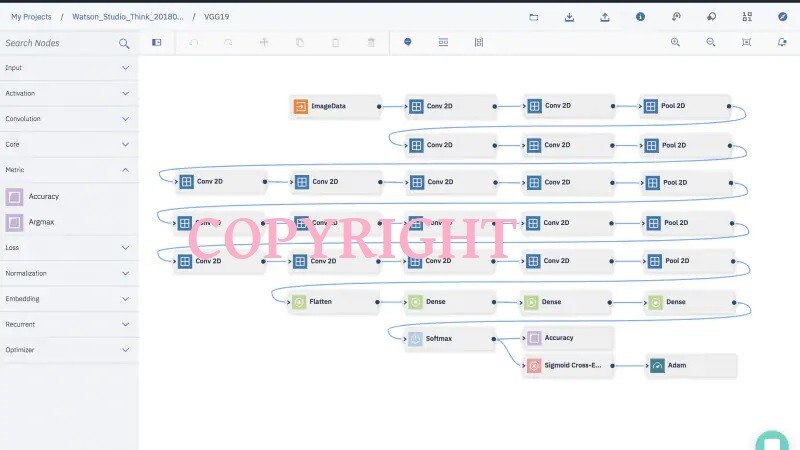
The creation and development of a robust neural network is a labor-intensive and time consuming endeavor. That’s why a team of IBM researchers recently developed a way for AI developers to protect their intellectual property.
Much like digital watermarking, it embeds information into a network than can then be triggered for identification purposes. If you’ve spent hundreds of hours developing and training AI models, and someone decides to exploit your hard work, IBM’s new technique will allow you to prove that the models are yours.
IBM’s method involves embedding specific information within deep learning models and then detecting them by feeding the neural network an image that triggers an abnormal response. This allows the researchers to extract the watermark, thus proving the model’s ownership.
According to a blog post from IBM, the watermark technique was designed so that a clever bad actor couldn’t just open up the code and delete the watermark:
… the embedded watermarks in DNN models are robust and resilient to different counter-watermark mechanisms, such as fine-tuning, parameter pruning, and model inversion attacks.
Interestingly, the watermark doesn’t add any code bloat, which is important because neural networks can be incredibly resource intensive. But according to Marc Ph. Stoecklin, Manager, Cognitive Cybersecurity Intelligence, IBM Research, and co-author of the project’s white paper, it’s not an issue.
We asked Stoecklin if the watermarks could affect neural network performance, he told TNW:
No, not during the classification process. We observe a negligible overhead during training (training time needed); moreover, we also observed a negligible effect on the model accuracy (non-watermarked model: 78.6%, watermarked model: 78.41% accuracy on a given image recognition task set, using the CIFAR10 data).
The project is still in the early stages, but IBM eventually plans to use the technique internally, with an eye towards commercialization as development continues.
Get the TNW newsletter
Get the most important tech news in your inbox each week.