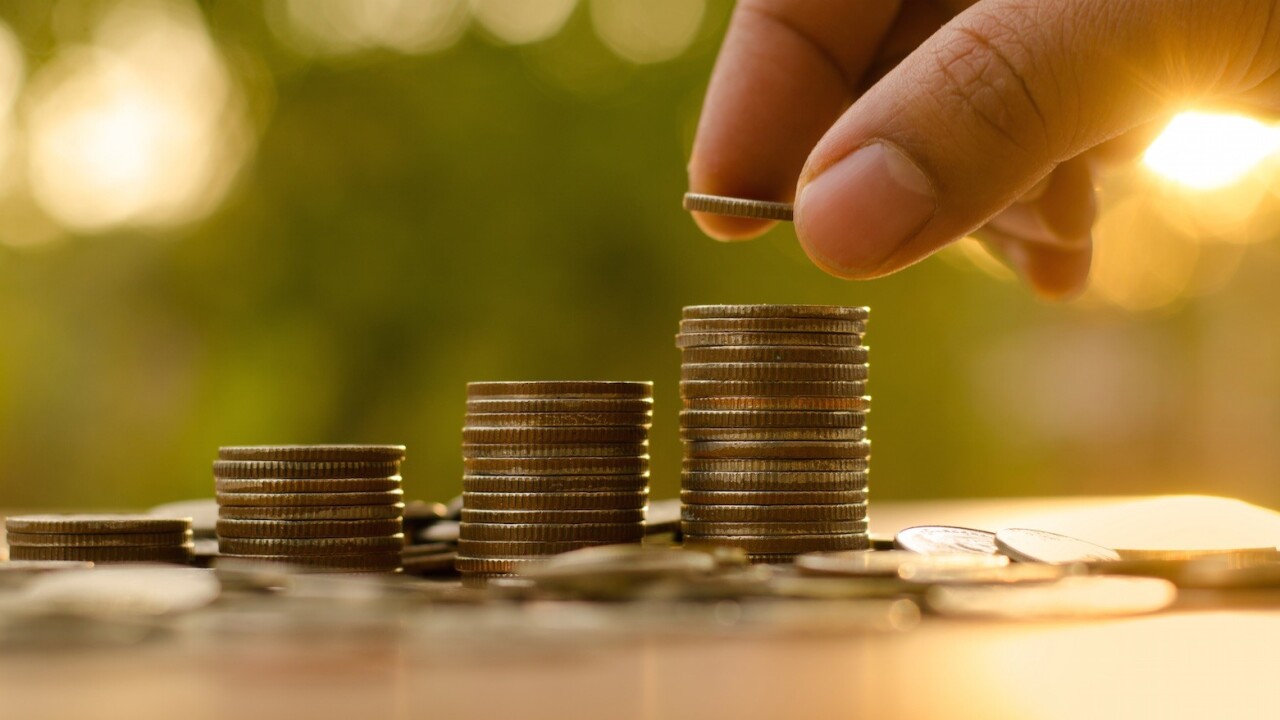
“All models are wrong, but some are useful.” This is a famous quote from 20th century statistical thinker, George E.P. Box.
This might seem like a strange message- shouldn’t all the models we build be as correct as possible? However, as a data scientist myself, I see great wisdom in this statement. After all, businesses don’t buy AI for model accuracy, they buy it to drive business value. Many businesses are investing in AI today without fully realizing its potential to deliver business impact. It’s time to shift the conversation.
The problem? Most groups embark on building their AI solution by discussing what they want to predict, and quickly shift to a discussion about model accuracy. This strategy often leads data scientists into the doldrums of model metrics that have no connection to business KPIs. Instead, we must focus on desired business outcomes, and what actions AI can prescribe we take in order to achieve those goals.
Let’s illustrate this with an example of a software company. The accounts receivable team of this company might use AI to predict if an invoice will be paid on time. In isolation this prediction has limited business value — an accurate prediction of each customer paying on time doesn’t quite meet the goal of shrinking the cash revenue cycle. Instead, this team should think about the AI solution holistically: how can they align their prediction with key recommendations and actions that will help the user focus their time.
So how do we achieve this? We need to break down the silos between business leaders and data scientists. Critically, let’s get business leaders and data scientists to follow four key pillars together, which will align organizations around a smarter, core approach:
- MEASURE the KPI. What is the business outcome we are tracking and used as the measure to track impact for your model?
- INTERVENE based on what the AI prescribes. What organizational levers and limitations exist and how can your AI provide guidance?
- EXPERIMENT to measure impact. Build models and deploy these in controlled experiments to attribute impact to the use of AI.
- ITERATE by constantly monitoring, optimizing and experimenting. Data changes, opportunities arise, no model lives in perpetuity.
These four pillars will help data scientists surface more valuable questions to their business counterparts and give said business leaders a deeper understanding of the power of AI within the organization. Too often it’s difficult or time consuming for technologists to educate their business counterparts on AI or ask them why a particular predictive model is being suggested. AI can be more than the datasets that power it. Adopting these four pillars and having honest conversations early and often can lead to more agility and resilience — critical as local to global events shift the business landscape around us, from temporary anomalies to black swan events.
Let’s return to the business outcome we were discussing – receiving payment on invoices.
Typically, businesses will build a predictive model to flag which customers may be at risk of not paying on time. But if we focus on a better way of measuring impact, we’d turn that predictive flag into a prescriptive solution and train the model to increase the expected revenue received within 30 days of sending the invoice.
Today, the staff in accounts receivable may have several tools at their disposal to ensure that payment is collected within 30 days. Each have their own effectiveness, from phone calls to email nudges, automatic payment suggestions or texts about suspending service. Staff can choose from any number of these actions in order to try to hit a target, however they may be constrained on where to spend their time. A model that predicts outcomes alone fall short of helping the staff choose what action to take. Instead, try building models that predict outcomes given those interventions, therefore influencing actions that yield optimal results.
We’ve now turned our predictive flag program into prescribed interventions. Models are not meant to be static, however, and so running tests, tracking real-time interactions, getting access to temporal data (in sequence) and monitoring your KPI is critical step to making sure your models don’t crumble when facing unforeseen events. Models will not live in perpetuity, so be agile and know how to deploy new models. Iteration is not only about fixing problem; it is also about opportunity. Yes, it will let you respond quickly to problems like data drift, but it also lets you experiment – constantly moving your business forward.
This mind shift from predictive to prescriptive is a natural evolution in how we understand and harness AI within their business. And it is more important in today’s highly-unpredictable economic and competitive business climate, where the ability to make real-time decisions and quickly deliver value can separate the winners from the losers.
Get the TNW newsletter
Get the most important tech news in your inbox each week.