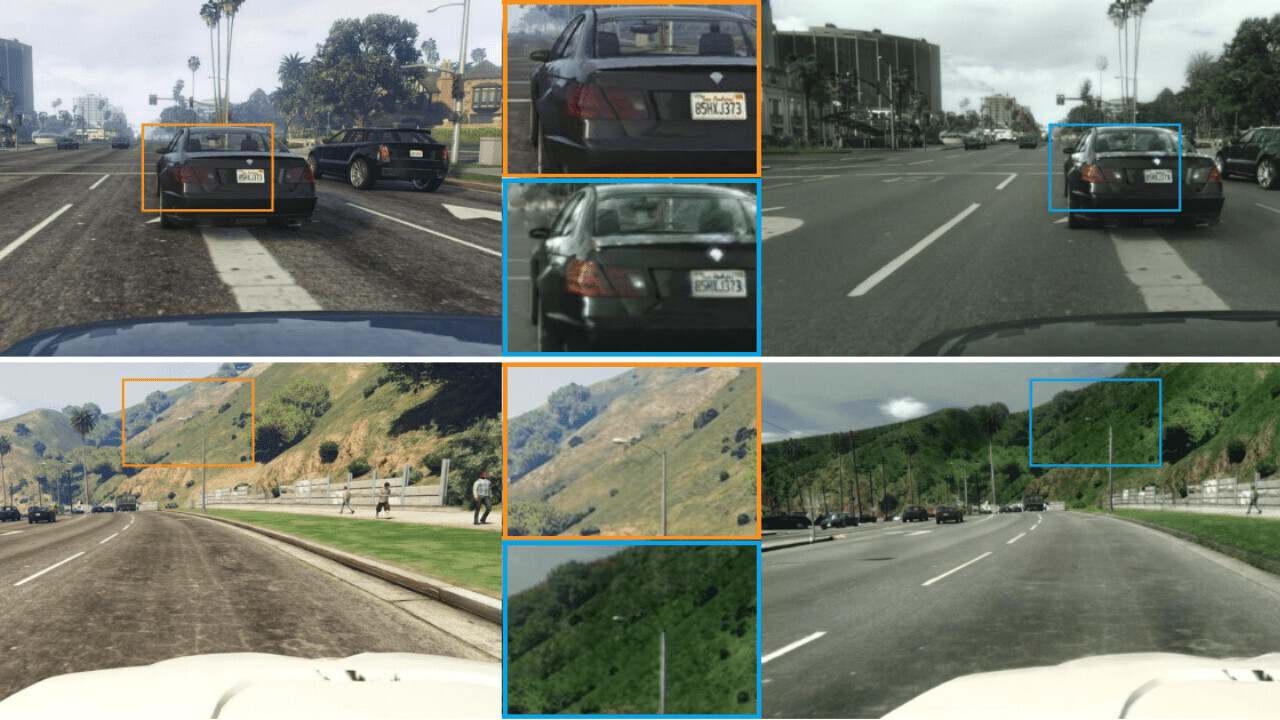
Nearly eight years after Grand Theft Auto V was released, modders are still enriching — and ruining — the game’s celebrated visuals.
Last week, researchers from Intel Labs unveiled an AI-powered revamp of the Rockstar classic that brings the graphics close to photorealism.
TNW spoke to Intel research scientist Stephen Richter to find out more about the technique — and the potential to productionize the method.
The cornerstone of the Intel Labs method is a convolutional network, a deep learning architecture that’s commonly used for image processing.
The team trained their convolutional networks on real-life images to translate GTA V’s graphics to a model of reality.
Richter said convolutional networks are well-suited to learning this type of task:
For games, simulations, and films, there is a tremendous amount of work that needs to go into modeling objects, materials, etc. to make them look realistic. Set up the right way, convolutional networks can just learn these things directly from real-world photo collections automatically.
The resulting output is strikingly realistic: reflections are added to the windows of cars, roads are paved with smoother asphalt, and the surrounding vegetation gains a lusher texture.
[Read more: This dude drove an EV from the Netherlands to New Zealand — here are his 3 top road trip tips]
Perhaps the most interesting aspect of the revamped graphics is the influence different training datasets have on the output.
In one application of their method, the researchers trained the convolutional network on the Cityscapes dataset, a collection of images recorded primarily in Germany. As a result, GTA V’s parched hills were reforested to mimic the German climate, while San Andreas acquired a grey hue that’s more resemblant to Bavaria than Southern California.
When the network was trained on the more diverse Mapillary Vistas dataset, however, the visual style of the output was brighter and more vibrant.
Some of these changes are a reflection of the location where the training images were recorded. But other differences are due to the cameras that captured the pictures.
The changes to the vegetation, for instance, were because Cityscapes represents mostly German cities. But Richter said the revamped color palette was due to the recording equipment:
Cityscapes was recorded with an automotive-grade camera, which has this characteristic green tint. Consequently, images enhanced to look like Cityscapes also get this green tint. Vistas was recorded with a diverse set of cameras, including, for example, smartphone cameras. Images from Vistas are much more vibrant and you can see this in the results by our method.
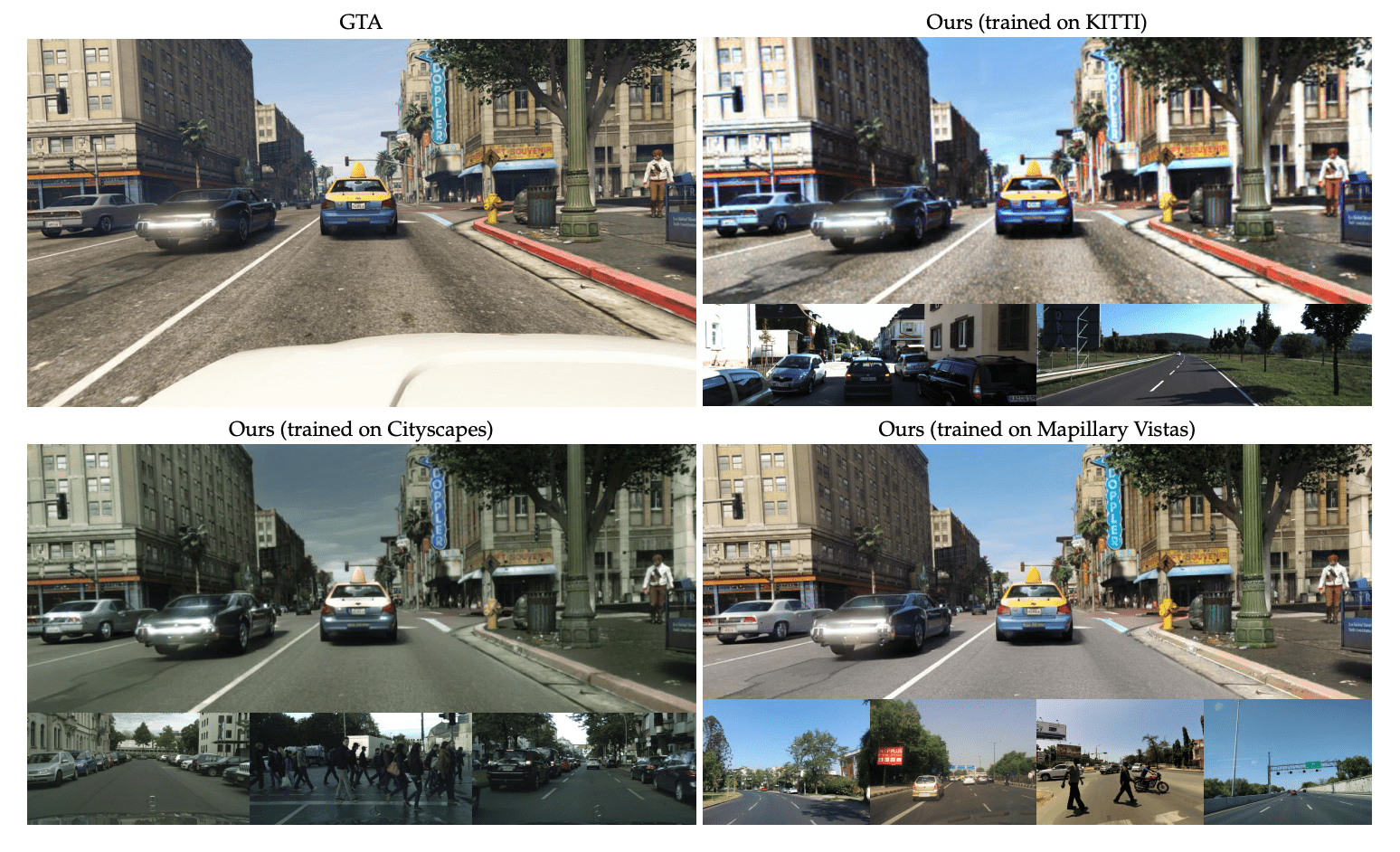
The researchers acknowledge that their approach isn’t perfect. While the method excels at road textures, cars, and vegetation, objects and scenes that are less common in the training data, such as close-up pedestrians, are modified less convincingly.
Richter said unrealistic aspects of GTA V also impacted the output:
There is far less traffic in GTA than in the real world, be it California or German cities. The way our method is set up, it should not and will not change this. It will not add cars or pedestrian if they are not there in the original game footage.
The same happens with trash on the street or the sidewalk. So the clean scenes from GTA with less dirt and traffic than the real world may look less realistic than real photos.
The method still needs further optimization for real-time applications. However, the researchers envision integrating it into game engines to advance the level of realism.
Richter said some existing game engines already enable access to data that can be connected to the system:
Depending on the game and the budget, I can imagine people playing around with this technique and maybe integrating it for some parts either during development or post-processing of games, simulations, or movies.
I’m also intrigued by the prospect of adapting games to new settings. Perhaps one day we’ll be able to relocate Assassin’s Creed to a photorealistic Khmer Empire, or transport Red Dead Redemption to Ned Kelly’s Australia? It won’t happen tomorrow, but we’re getting closer to video game footage that’s indistinguishable from real life.
Greetings Humanoids! Did you know we have a newsletter all about AI? You can subscribe to it right here.
Get the TNW newsletter
Get the most important tech news in your inbox each week.