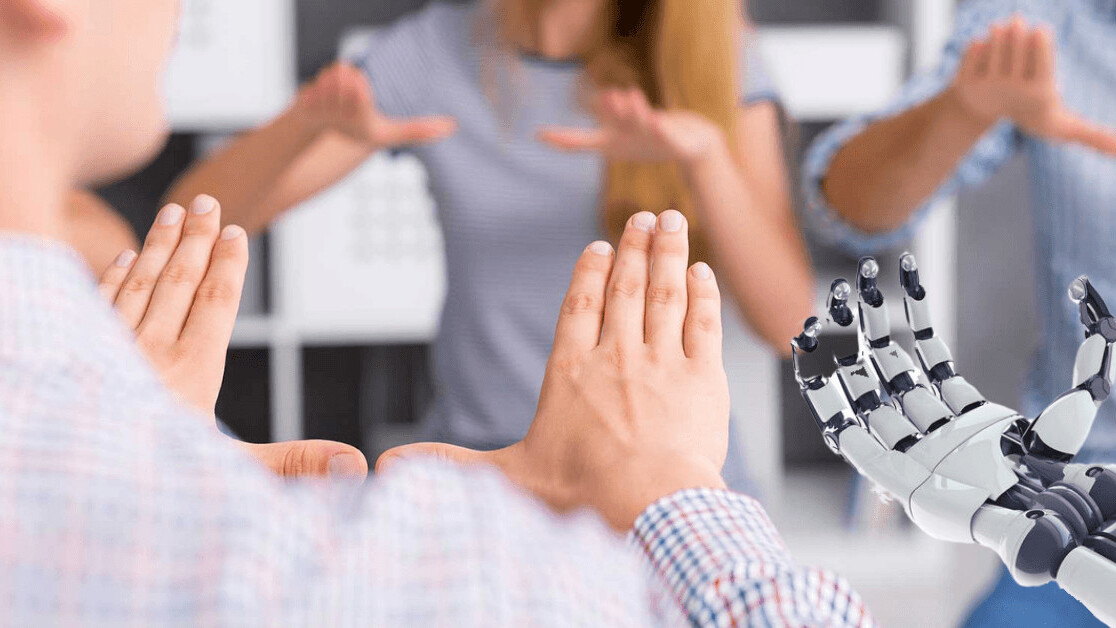
Sign languages aren’t easy to learn and are even harder to teach. They use not just hand gestures but also mouthings, facial expressions and body posture to communicate meaning. This complexity means professional teaching programs are still rare and often expensive. But this could all change soon, with a little help from artificial intelligence (AI).
My colleagues and I are working on software for teaching yourself sign languages in an automated, intuitive way. Currently, this tool can analyze the way a student performs a sign in Swiss-German sign language and provide detailed feedback on how to improve the hand shape, motion, location and timing. But our hope is that we can use the AI behind the tool to create software that can teach various sign languages from around the world, and take into account more intricate features of the languages, such as sentence grammar and the non-hand elements of communication.
AI has previously been used for the recognition, translation or interpretation of sign language. But we believe we are the first to actually attempt to assess the signs a person makes. More importantly, we want to leverage the AI technology to provide feedback to the user about what they did wrong.
Practicing and assessing sign language is hard because you can’t read or write it. Instead, we have created a computer game. To practice a sign, the game shows you a video of that sign being performed, or gives you the nearest spoken word that describes it (or both). It then records your attempt to recreate the sign using a video camera and tells you how you can do better. We’ve found that making it a game encourages people to compete to get the best score and improve their signing along the way.
Artificial intelligence is used at all stages of performance assessment. First, a convolutional neural network (CNN) extracts information from the video about the pose of your upper body. A CNN is a type of AI loosely based on the processing done by the visual cortex in your brain. Your skeletal pose information and the original video is then sent to the hand shape analyzer, where another CNN looks at the video and pulls out hand shape information at each point in the video.
The skeletal information and hand shapes are then sent to a hand motion analyzer, which uses something called a Hidden Markov model (HMM). This type of AI allows us to model the skeleton and hand shape information over time. It then compares what it has seen to a reference model which represents the perfect version of that sign, and produces a score of how well it matches.
The results of both the hand shape analyzer and the hand motion analyzer are then scored and presented to you as feedback. So all the AI is hidden behind a simple-to-use interface, letting you focus on the learning. Our hope is that the automatic, personal feedback will make students more engaged with the process of learning to sign.
Bringing AI to the classroom
So far, the software only works for Swiss-German sign language. But our research suggests that the “architecture” of the system wouldn’t need to change to deal with other languages. It would just need more video recordings of each language to act as data to train it with.
An area of research we would like to explore is how we could use what the AI already knows to help it learn new languages. We’d also like to see how we can add other aspects of communication while using sign language, such as facial expressions.
At the moment, the software works best in a simple environment such as a classroom. But if we can develop it to tolerate more variation in the background of the video footage it is assessing it could become like many popular apps that allow you to learn a language wherever you are without the help of an expert. With this sort of technology being developed, it will soon be possible to make learning sign languages just as accessible to everyone as learning their spoken siblings.
This article is republished from The Conversation by Stephanie Stoll, PhD Candidate in Computer Vision, University of Surrey under a Creative Commons license. Read the original article.
Get the TNW newsletter
Get the most important tech news in your inbox each week.