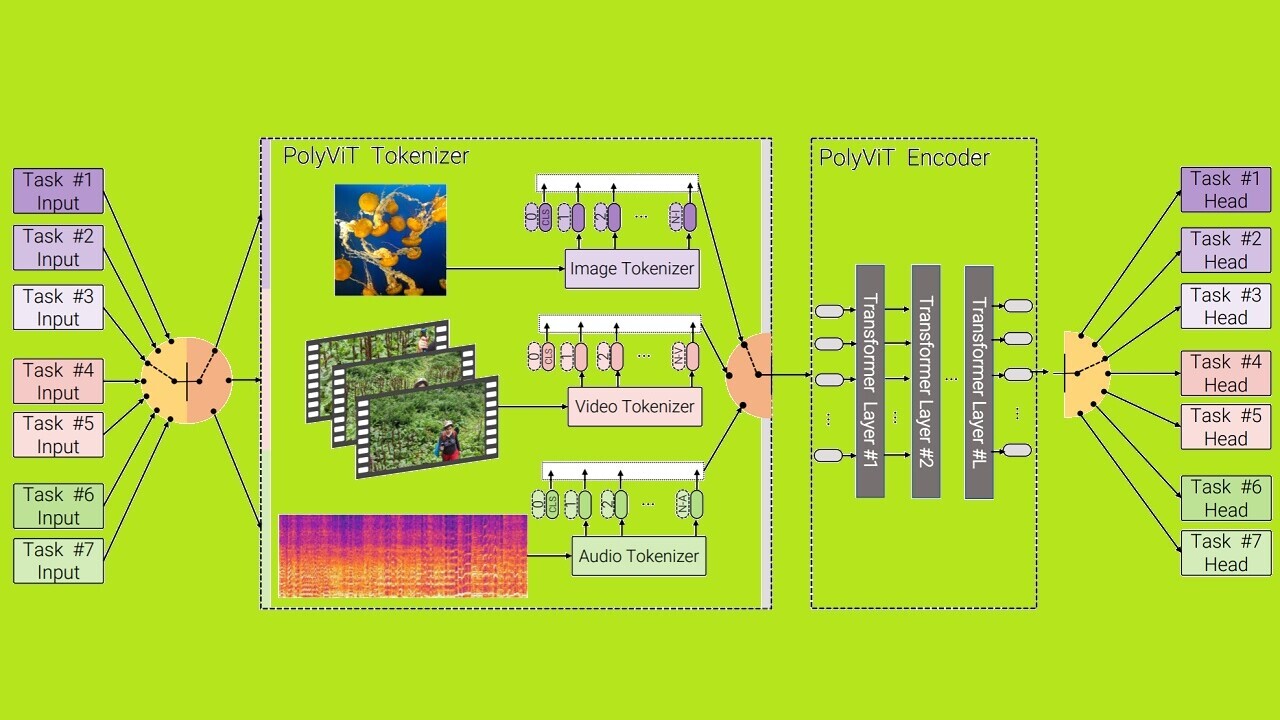
A team of scientists from Google Research, the Alan Turing Institute, and Cambridge University recently unveiled a new state of the art (SOTA) multimodal transformer for AI.
In other words, they’re teaching AI how to ‘hear’ and ‘see’ at the same time.
Up front: You’ve probably heard about transformer AI systems such as GPT-3. At their core, they process and categorize data from a specific kind of media stream.
Under the current SOTA paradigm, if you wanted to parse the data from a video you’d need several AI models running concurrently.
You’d need a model that’s been trained on videos and another model that’s been trained on audio clips. This is because, much like your human ears and eyes are entirely different (yet connected) systems, the algorithms necessary to process different types of audio are typically different than those used to process video.
Per the team’s paper:
Despite recent advances across different domains and tasks, current state-ofthe-art methods train a separate model with different model parameters for each task at hand. In this work, we present a simple yet effective method of training a single, unified model that achieves competitive, or state-of-the-art results for image, video, and audio-classification.
Background: What’s incredible here is that the team was not only able to build a multimodal system capable of handling its related tasks simultaneously, but that in doing so they managed to outperform current SOTA models that are focused on a single task.
The researchers dub their system “PolyVit.” And, according to them, it currently has virtually no competition:
By co-training different tasks on a single modality, we are able to improve the accuracy of each individual task and achieve state-of-the-art results on 5 standard video- and audio-classification datasets. Co-training PolyViT on multiple modalities and tasks leads to a model that is even more parameter-efficient, and learns representations that generalize across multiple domains.
Moreover, we show that co-training is simple and practical to implement, as we do not need to tune hyperparameters for each combination of datasets, but can simply adapt those from standard, single-task training.
Quick take: This could be a huge deal for the business world. One of the biggest problems facing companies hoping to implement AI stacks is compatibility. There are literally hundreds of machine learning solutions out there and there are no guarantees they’ll work together.
This results in monopolistic implementations where IT leaders are stuck with a single vendor for compatibility’s sake or a mix-and-match approach that comes with more headaches than it’s usually worth.
A paradigm where multimodal systems become the norm would be a godsend for weary administrators.
Of course, this is early research from a pre-print paper so there’s no reason to believe we’ll see this implemented widely any time soon.
But it is a great step towards a one-size-fits all classification system, and that’s pretty exciting stuff.
H/t: Synced
Get the TNW newsletter
Get the most important tech news in your inbox each week.