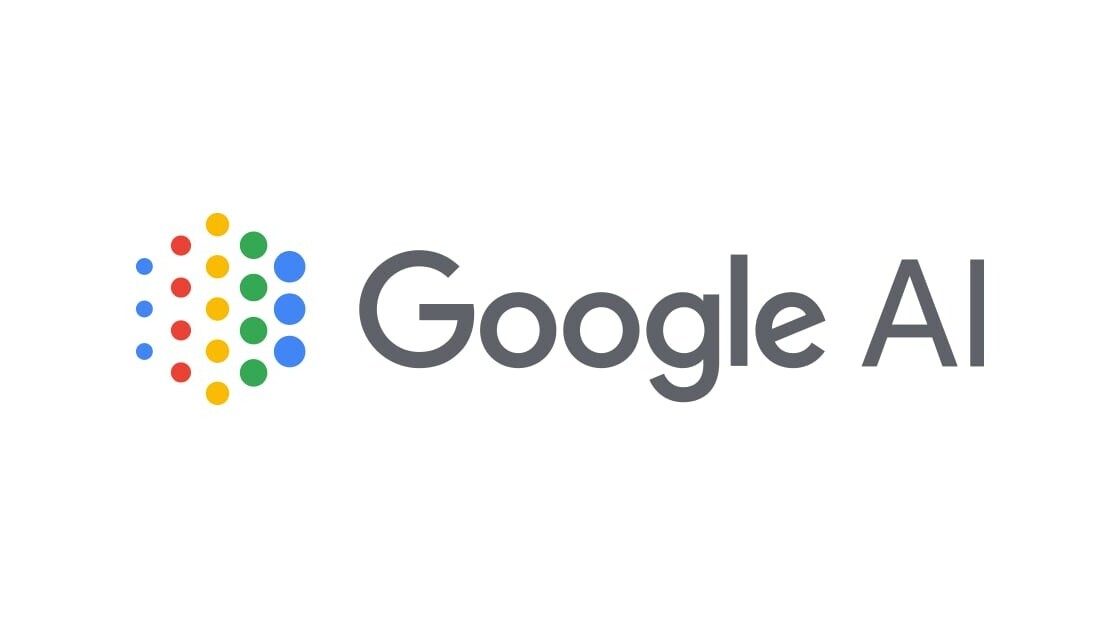
A trio of researchers from the Google Brain team recently unveiled the next big thing in AI language models: a massive one trillion-parameter transformer system.
The next biggest model out there, as far as we’re aware, is OpenAI’s GPT-3, which uses a measly 175 billion parameters.
Background: Language models are capable of performing a variety of functions but perhaps the most popular is the generation of novel text. For example, you can go here and talk to a “philosopher AI” language model that’ll attempt to answer any question you ask it (with numerous notable exceptions).
[Read next: How Netflix shapes mainstream culture, explained by data]
While these incredible AI models exist at the cutting-edge of machine learning technology, it’s important to remember that they’re essentially just performing parlor tricks. These systems don’t understand language, they’re just fine-tuned to make it look like they do.
That’s where the number of parameters comes in – the more virtual knobs and dials you can twist and tune to achieve the desired outputs the more finite control you have over what that output is.
What Google’s done: Put simply, the Brain team has figured out a way to make the model itself as simple as possible while squeezing in as much raw compute power as possible to make the increased number of parameters possible. In other words, Google has a lot of money and that means it can afford to use as much hardware compute as the AI model can conceivably harness.
In the team’s own words:
Switch Transformers are scalable and effective natural language learners. We simplify Mixture of Experts to produce an architecture that is easy to understand, stable to train and vastly more sample efficient than equivalently-sized dense models. We find that these models excel across a diverse set of natural language tasks and in different training regimes, including pre-training, fine-tuning and multi-task training. These advances make it possible to train models with hundreds of billion to trillion parameters and which achieve substantial speedups relative to dense T5 baselines.
Quick take: It’s unclear exactly what this means or what Google intends to do with the techniques described in the pre-print paper. There’s more to this model than just one-upping OpenAI, but exactly how Google or its clients could use the new system is a bit muddy.
The big idea here is that enough brute force will lead to better compute-use techniques which will in turn make it possible to do more with less compute. But the current reality is that these systems don’t tend to justify their existence when compared to greener, more useful technologies. It’s hard to pitch an AI system that can only be operated by trillion-dollar tech companies willing to ignore the massive carbon footprint a system this big creates.
Context: Google’s pushed the limits of what AI can do for years and this is no different. Taken by itself, the achievement appears to be the logical progression of what’s been happening in the field. But the timing is a bit suspect.
FYI @mmitchell_ai and I found out there was a 40 person meeting in September about LLMs at Google where no one from our team was invited or knew about this meeting. So they only want ethical AI to be a rubber stamp after they decide what they want to do in their playground. https://t.co/tlT0tj1sTt
— Timnit Gebru (@timnitGebru) January 13, 2021
H/t: Venture Beat
Get the TNW newsletter
Get the most important tech news in your inbox each week.