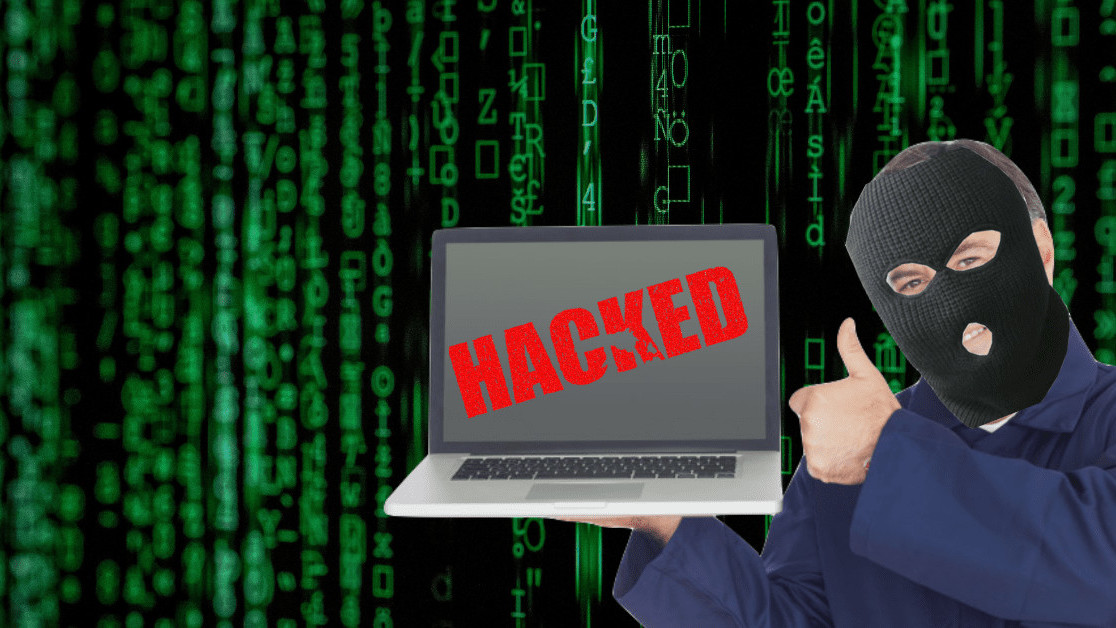
Artificial intelligence is captivating tech news audiences. Unfortunately, growing expectations for AI’s impact on legitimate business have spawned a distracting narrative about potential AI-powered cyberattacks. Is this really a threat that needs to be on our radar?
Based on my work examining dark web markets and the techniques cybercriminals use to collect, resell or commit fraud with stolen data, I question the usefulness of AI to run-of-the-mill cybercrime.
Most AI still falls short of “intelligent”
The first problem with supposed “AI hacking” is that AI tools as a whole are limited in actual intelligence. When we talk about AI, we mostly mean data science – using massive data sets to train machine learning models. Training machine learning models is time consuming and takes an enormous amount of data, and the results are models still limited to binary actions.
To be useful to hackers, machine learning tools need to be able to take an action, create something or change themselves based on what they encounter when deployed and how they’ve been trained to react. Individual hackers may not have enough data on attacks and their outcomes to build creative or flexible, self-adjusting models.
For example, threat actors today use machine learning models to bypass CAPTCHA challenges. By taking CAPTCHA codes – the oddly-shaped numbers and letters you re-type to prove you’re human – and splitting them into images, image-recognition models can learn to identify the images and enter the correct sequence of characters to pass the CAPTCHA test. This type of model lets the automated credential stuffing tools actors use pass as human, so attackers can gain fraudulent access to online accounts.
This technique is clever, but it’s less an example of an intelligent model than effective data science. The CAPTCHA crackers are essentially matching shapes, and the fix for this CAPTCHA vulnerability is to create a more delicate test of real intelligence, like asking users to identify parts of an image containing a car or storefront.
To crack these more difficult challenges, a threat actor’s model would need to be trained on a data set of categorized images to apply its “knowledge” of what a car, storefront, street sign or other random item is, then carefully select partitioned pieces of that item as being part of the whole – which would probably require another level of training on partial images. Obviously, this display of artificial intelligence would require more data resources, data science expertise and patience than the average threat actor may have. It’s easier for attackers to stick with simple CAPTCHA crackers and accept that in credential stuffing attacks, you win some and you lose some.
What AI can hack
A 2018 report titled “The Malicious Use of Artificial Intelligence,” pointed out that all known examples of AI hacking used tools developed by well-funded researchers who are anticipating the weaponization of AI. Researchers from IBM created evasive hacking tools last year, and an Israeli team of researchers used machine learning models to spoof problematic medical images earlier this year, to name a few examples.
The report is careful to note that there is some anecdotal evidence of malicious AI, but it “may be difficult to attribute [successful attacks] to AI versus human labor or simple automation.” Since we know that creating and training machine learning models for malicious use requires a lot of resources, it’s unlikely there are many, if any, examples where machine learning played a major role in cybercrime.
Machine learning may be deployed by attackers in years to come, as malicious applications designed to disrupt legitimate machine learning models become available for purchase on dark web networks. (I’m doubtful someone with resources to develop malicious AI would need to generate income from the type of petty cybercrime that’s our biggest problem today; they’ll make their money selling software).
As the 2018 report on malicious AI noted, spear phishing attacks might be an early use case for this so-far-hypothetical breed of malicious machine learning. Attackers would name their target and let the program vacuum up public social media data, online activity and any available private information to determine an effective message, “sender,” and attack method to accomplish the hacker’s goal.
Evasive malware like what the IBM team developed last year might, in the future, be deployed against networks or used to create botnets. The malware could infect many connected devices on corporate networks, staying dormant until a critical mass was reached that would make it impossible for security pros to keep up with the infection. Similarly, AI tools might analyze system and user information from infected IoT devices to find new ways to forcibly recruit machines into a worldwide botnet.
However, because spear phishing and malware propagation are already both effective given a large enough attack surface, it still seems that a determined hacker would find it more cost-effective to do the work using simple automation and their own labor, rather than purchasing or creating a tool for these attacks.
So, what can AI models hack today? Not much of anything. The problem is, business is booming for hackers anyway.
Why AI just isn’t necessary
Somewhere, someone has your information. They might only have an email address, or your Facebook username, or maybe an old password that you’ve recently updated (you have updated it, right?).
Over time, these pieces get put together into a profile of you, your accounts, your interests and whether or not you take any security steps to prevent unauthorized account access. Then your profile gets sold off to several buyers who stick your email and password into automated tools that try your credentials on every banking, food delivery, gaming, email or other service the attacker wants to target – perhaps even software you use at work that will get them into corporate systems.
This is how the vast majority of hacks evolve. Because internet users can’t seem to beat bad passwords, stop clicking malicious links, recognize phishing emails, or avoid insecure websites. Machine learning is an overly complicated solution to the easily automated task of taking over accounts or duping victims into infecting their systems.
Sure, that’s a little bit of victim-shaming, but it’s important for the digital public to understand that before we worry about artificially-intelligent hacking tools, we need to fix the problems that let even technically-unskilled attackers make a living off of our personal information.
Get the TNW newsletter
Get the most important tech news in your inbox each week.