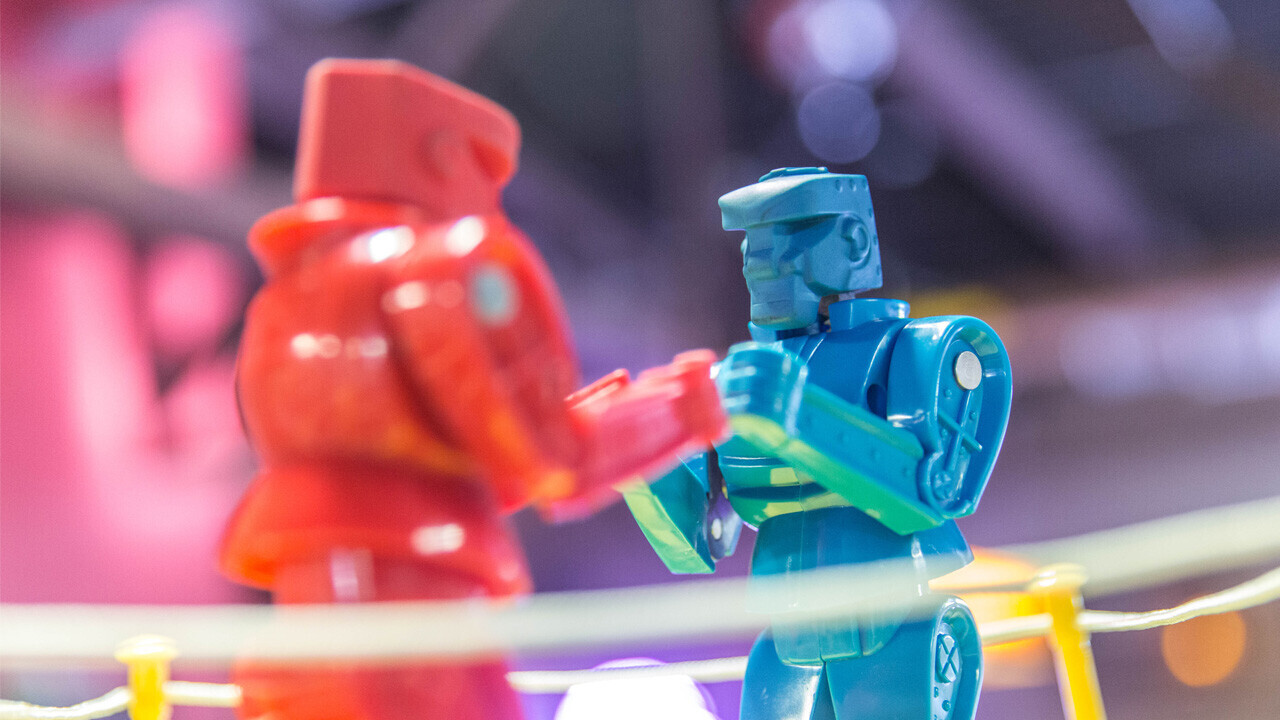
There’s nothing quite so dramatic and inspirational as a scientific breakthrough. But what happens when different groups of scientists can’t seem to agree on the science?
DeepMind, an Alphabet research company based in London, published a fascinating research paper last year wherein it claimed to have solved the huge challenge of “simulating matter on the quantum scale with AI.” Now, nearly eight months later, a group of academic researchers from Russia and South Korea may have uncovered a problem with the original research that places the paper’s entire conclusion in doubt.
The implications for this cutting-edge research could be huge, if the paper’s conclusions are true. In essence, we’re talking about the potential to use artificial intelligence to discover new ways to manipulate the building blocks of matter.
A new hope
The big idea here involves being able to simulate quantum interactions. Our world is made up of matter which is made up of molecules that are made up of atoms. At each level of abstraction, it becomes harder and harder to simulate.
By the time you reach the quantum level, which exists inside of atoms, the problem of simulating potential interactions becomes incredibly challenging.
Per a blog post from DeepMind:
To do this on a computer requires the simulation of electrons, the subatomic particles that govern how atoms bond to form molecules and are also responsible for the flow of electricity in solids.
Despite decades of effort and several significant advances, accurately modelling the quantum mechanical behaviour of electrons remains an open challenge.
The basic problem is that it’s really hard to predict the probabilities of a given electron ending up in a specific position. And the complexity increases the more you add.
As DeepMind pointed out in the same blog post, a pair of physicists back in the 1960s came up with a breakthrough:
Pierre Hohenberg and Walter Kohn realised that it is not necessary to track each electron individually. Instead, knowing the probability for any electron to be at each position (i.e., the electron density) is sufficient to exactly compute all interactions. Kohn received a Nobel Prize in Chemistry after proving this, thus founding Density Functional Theory (DFT).
Unfortunately, DFT could only simplify the process so far. The “functional” part of the theory relied on humans to do all the heavy lifting.
That all changed back in December when DeepMind published a paper entitled “Pushing the frontiers of density functionals by solving the fractional electron problem.”
In this paper, the DeepMind team claims to have radically improved current methods for modeling quantum behavior through the development of a neural network:
By expressing the functional as a neural network and incorporating these exact properties into the training data, we learn functionals free from important systematic errors — resulting in a better description of a broad class of chemical reactions.
The academics strike back
DeepMind’s paper made it through the initial, formal review process and all was well. Until August 2022 rolled around and a team of eight academics from Russia and South Korea published a comment questioning its conclusion.
Per a press release from Skolkovo Institute of Science and Technology:
DeepMind AI’s ability to generalize the behavior of such systems does not follow from the published results and requires revisiting.
In other words: the academics are disputing how DeepMind’s AI came to its conclusions.
According to the commenting researchers, the training process that DeepMind used to build its neural network taught it how to memorize the answers to the specific problems it was going to face during benchmarking — the process by which scientists determine if one approach is better than another.
In their comment, the researchers write:
Although the conclusion of Kirkpatrick et al. about the role of FC/FS systems in the training set may be correct, it is not the only possible explanation for their observations.
In our opinion, the improvements in the performance of DM21 on the BBB test dataset relative to DM21m may be caused by a much more prosaic reason: an unintended overlap between the training and test datasets.
If this is true, it would mean DeepMind didn’t actually teach a neural network to predict quantum mechanics.
Return of the AI
DeepMind was quick to respond. The company published its response on the same day as the comment and provided an immediate and firm rebuke:
We disagree with their analysis and believe that the points raised are either incorrect or not relevant to the main conclusions of the paper and to the assessment of general quality of DM21.
The team expands on this throughout its retort:
DM21 is not memorizing the data; this is simply shown by the fact that the DM21 Exc changes over the full range of distances considered in BBB and is not equal to the infinite separation limit, as shown in Fig. 1, A and B, for H2+ and H2. For example, at 6 Å, the DM21 Exc is ~13 kcal/mol from the infinite limit in both H2+ and H2 (although in opposite directions).
And, while it’s beyond the scope of this article to explain the above jargon, we can safely assume that DeepMind was likely prepared for that particular objection.
As to whether that solves the problem remains to be seen. At this point, we’ve yet to see further rebuttal from the academic team to see if their concerns have been assuaged.
In the meantime, it’s possible that the ramifications of this discussion could go far beyond just affecting a single research paper.
As the fields of artificial intelligence and quantum science become increasingly intertwined, they’re also becoming more and more dominated by corporate research tanks with deep pockets.
What happens when there’s a scientific deadlock — opposing sides are unable to agree on the efficacy of a given technological approach via the scientific method — and corporate interests come into play?
What now?
The core of the problem could lie in the inability to explain how AI models “crunch the numbers” to come to the conclusions they do.
These systems can go through millions of permutations before outputting an answer. It would be impossible to explain every step of the process, which is exactly why we need algorithmic shortcuts and AI to brute force mass-scale problems that would be too large for a human or computer to solve head on.
Eventually, as AI systems continue to scale, we could reach a point where we no longer have the tools necessary to understand how they work. When this happens, we could see a divergence between corporate technology and that which passes external peer review.
That’s not to say DeepMind’s paper is an example of this. As the commenting academic team wrote in their press release:
The usage of fractional-electrons systems in the training set is not the only novelty in the work by DeepMind. Their idea of introducing the physical constraints into a neural network via the training set, as well as the approach for imposing physical sense through training on the correct chemical potential, are likely to be widely used in construction of neural network DFT functionals in the future.
But we’re experiencing a bold, new, AI-powered technology paradigm. It’s probably time we started considering what the future looks like in a post-peer-review world.
Get the TNW newsletter
Get the most important tech news in your inbox each week.