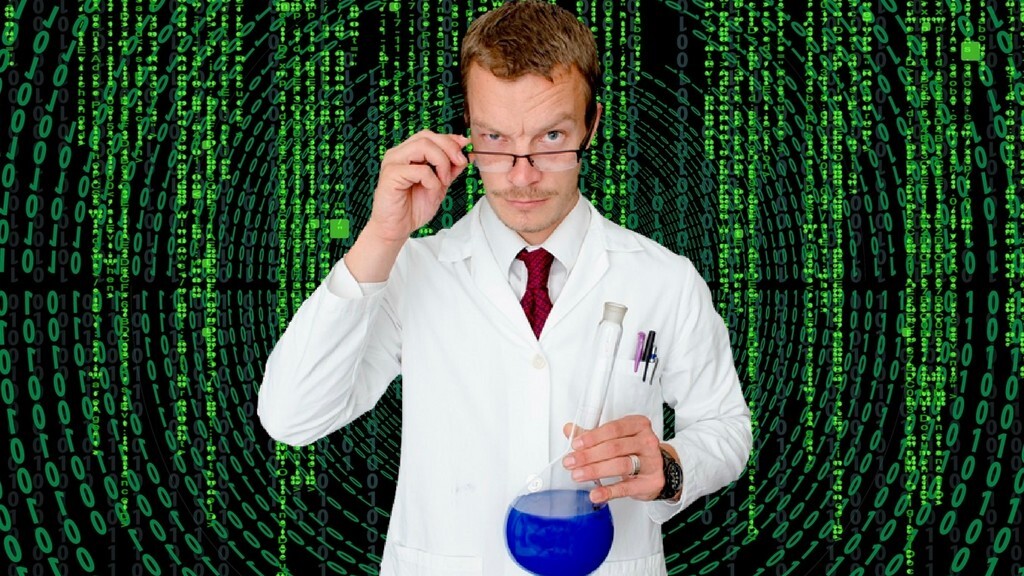
This is an excerpt from a long interview between an anonymous data scientist and Logic Magazine about AI, deep learning, FinTech, and the future, conducted in November 2016.
LOGIC: Alright, let’s get started with the basics. What is a data scientist? Do you self-identify as one?
DATA SCIENTIST: I would say the people who are the most confident about self-identifying as data scientists are almost unilaterally frauds.
They are not people that you would voluntarily spend a lot of time with. There are a lot of people in this category that have only been exposed to a little bit of real stuff — they’re sort of peripheral.
You see actually a lot of this with these strong AI companies: companies that claim to be able to build human intelligence using some inventive “Neural Pathway Connector Machine System,” or something. You can look at the profiles of every single one of these companies. They are always people who have strong technical credentials, and they are in a field that is just slightly adjacent to AI, like physics or electrical engineering.
And that’s close, but the issue is that no person with a PhD in AI starts one of these companies, because if you get a PhD in AI, you’ve spent years building a bunch of really shitty models, or you see robots fall over again and again and again. You become so acutely aware of the limitations of what you’re doing that the interest just gets beaten out of you. You would never go and say, “Oh yeah, I know the secret to building human-level AI.”
So I think a lot of the strong AI stuff is like that. A lot of data science is like that too. Another way of looking at it is that it’s a bunch of people who got PhDs in the wrong thing, and realized they wanted to have a job.
Another way of looking at it — I think the most positive way, which is maybe a bit contrarian — is that it’s really, really good marketing.
As someone who tries not to sell fraudulent solutions to people, it actually has made my life significantly better because you can say “big data machine learning,” and people will be like, “Oh, I’ve heard of that, I want that.” It makes it way easier to sell them something than having to explain this complex series of mathematical operations.
The hype around it — and that there’s so much hype — has made the actual sales process so much easier.
I’m curious about the origins of the term “data science” — do you think that it came internally from people marketing themselves, or whether it was a random job title used to describe someone, or what?
As far I know, the term “data science” was invented by Jeff Hammerbacher at Facebook.
The Cloudera guy?
Yeah, the Cloudera guy. As I understand it, “data science” originally came from the gathering of data on his team at Facebook.
If there was no hype and no money to make, essentially what I would say data science is, is the fact that the data sets have gotten large enough where you can start to consider variable interactions in a way that’s becoming increasingly predictive. And there are a number of problems where the actual individual variables themselves don’t have a lot of meaning, or they are kind of ambiguous, or they are only very weak signals.
There’s information in the correlation structure of the variables that can be revealed, but only through really huge amounts of data.
So essentially: there are N variables, right? So there’s N-squared potential correlations, and N-cubed potential cubic interactions or whatever. Right? There’s a ton of interactions. The only way you can solve that is by having massive amounts of data.
For people who are less familiar with these terms, how would you define data science, machine learning, and artificial intelligence? Because as you mentioned, these are terms that float around a lot in the media and that people absorb, but it’s unclear how they fit together.
I’m friends with a venture capitalist who became famous for coining the phrase “machine intelligence,” which is pretty much just the first word of “machine learning” with the second word of “artificial intelligence,” and as far as I can tell is essentially impossible to distinguish between either of those applications.
I would say, again, “data science” is really shifty. If you wanted a pure definition, I would say data science is much closer to statistics. “Machine learning” is much more predictive optimization, and “artificial intelligence” is increasingly hijacked by a bunch of yahoos and Elon Musk types who think robots are going to kill us.
I think artificial intelligence has gotten too hot as a term. It has a constant history since the dawn of computing of over-promising and substantially under-delivering.
So do you think when most people think of artificial intelligence, they think of strong AI?
They think of the film Artificial Intelligence level of AI, yeah. And as a result, I think people who are familiar with bad robots falling over shy away from using that term, just because they’re like, “We are nowhere near that.” Whereas a lot of people who are less familiar with shitty robots falling over will say, “Oh, yeah, that’s exactly what we’re doing.”
You can find the full interview here. Logic is a magazine about technology that comes out three times a year. To learn more, visit logicmag.io. You can also find them on Facebook, or follow them on Twitter here:
Get the TNW newsletter
Get the most important tech news in your inbox each week.