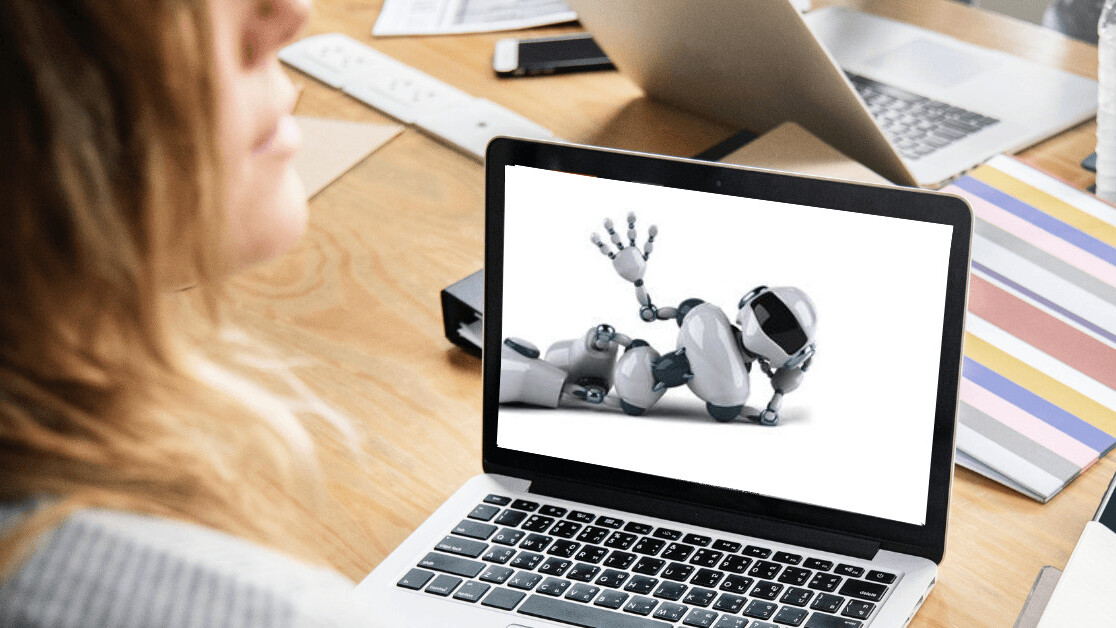
The race for chatbot supremacy certainly doesn’t produce the heightened tension of the space race, but there’s definitely an unofficial international competition going on and it’s fascinating to watch.
For those keeping score, the United States is hanging onto a slim lead, China is gaining fast ground, and most of Europe is close behind.
Among industries, financial services continues to hold a commanding advantage over telecom in terms of sophistication, breadth, and usefulness due to the transactional nature of the majority of banking transactions. Enterprise, banks, and financial institutions use Chatbots to reduce cost, improve speed, and improve success rate. Chatbots also gives enterprises a change to gather valuable information and experiment with new changes.
What these companies don’t yet realize is each Chatbot conversation should be considered a “transaction” or “business deal” even if no sale comes out of it for a variety of reasons, including: 1. The company obtains data they can use; 2. A great/bad experience can influence future sale and the customer’s opinion; and 3. Customers view chatbots as an extension of your company.
Slow data processing and management can ruin someone’s experience with chatbots within seconds. Companies who are able to store, process, and analyze data quickly and efficient will usher in a new generation of chatbots that can respond seamlessly and intelligently, providing recommendations rather than just reacting.
The best news, particularly from my perspective of being deeply involved in big data, artificial intelligence (AI), machine learning (ML), and analytics, is that advancements in chatbot technology continue to be arriving at a breakneck pace. This is making the accuracy and speed of chatbot technology greater all the time.
Chatbots: a technologist’s – and customer’s – dream
For those of us into such things, it’s a thrilling technology, combining the continually advancing fields of AI, ML, natural language processing (NLP), computational linguistics, big data, analytics, and bot logic and botflow making.
Obtaining data and training your chatbot to correctly process a conversation/situation is essential to be successful. Companies who are releasing chatbots early will give their product an advantage due to the amount of data they’re getting, which means enterprises should start adopting chatbots now while consumers don’t have very high expectations.
When done right, chatbots eliminate repetitive tasks – making workloads lighter, easier, and faster – while promoting greater customer satisfaction for the organizations that deploy them.
No real progress in chatbot technology can be made. However advancements in NLP, and chatbot technology advancements based on NLP, simply can’t happen without the ability to save, store, access, integrate with other customer data and analyze massive amounts of data in a cost-effective way. It is here where the technological foundation of all highly functional chatbots resides. You need a highly developed data management platform to run very complex analytics at scale and churn through a range of NLP models quickly and efficiently.
After all, it’s not very useful if it takes 12 hours to process the amount of data it takes to come up with a functional chatbot interaction. But if you can churn through that same amount of data in five minutes, then you’re more likely than not to develop a successful chatbot interaction.
Chatbots in finance
Built on processing massive amount of information, finance is an ideal industry for automation and reduction of salary expenditure, two issues chatbots addresses. However, a new study from PwC showed that two-thirds of U.S. financial services respondents surveyed said they’re limited by operations, regulations, budgets, or resources to make the investment in such innovative development.
Fintech companies such as Plum, Digit and Cleo use chatbots to drive microsaving by putting small amounts of money into saving accounts each day for their users. These companies’ chatbot is their core product, unlike legacy banks that use this function to supplement a core product.
More finance applications beyond just providing automated savings are being worked on every day, including using chatbots to detect and notify of fraudulent behavior, become a wealth management advisor, underwrite loans and insurance, and provide data analysis and advanced analytics.
Chatbots in banking
Chatbots in banking is relatively inexpensive to develop and maintain since they require less coding than standalone banking apps. Banks save the cost of developing their own channels thanks to the current growth in the popularity of messaging platforms, as well as save on data storage thanks to cloud-based systems.
Companies such as Cleo, Stripe, and Wealthfront are disrupting the market, but it’s more difficult for them to meet the demand of key bank products (such as loans) due to less restricted regulations that force their customers to spend heavily on compliance and maintain large capital cushions.
DBS Bank uses Kasisto’s KAI to allow customers to conduct transactions such as transfers and bill paying. In addition, customers can ask about their personal finances using messaging applications and through the banks platform. DBS’ digibank, India’s first digital-only bank, uses KAI to process 82 percent of customer requests, meaning digibank handles less than a fifth of all customer interactions.
Striving for the elusive 10 on a 1-to-10 scale
On a scale of 1-to-10, with 10 being a perfect state, chatbots are functioning at a 6.5 (a year ago, I’d say we were below 6.0). They’re actually very useful for a specific set of tasks such as asking for the balance in your bank account – but they’re still not very good in unstructured situations that are out of the realm of what they’ve been programmed to do.
As for the future, I think we can get to 9.0 in the next 18 to 36 months. We’re going to see very quick improvements in NLP in that short of a timespan. But then we’re going to hit a bump, because that last 10 percent will involve solving extremely challenging NLP issues such as nuances in language and style, and providing meaningful contexts in unstructured interactions.
This is all from the perspective of the English language, of course. Chatbot technology is predominantly English-centric because the amount of research and tradition in the field has been conducted in the United States. Plus, looking at the applications for NLP, English has been the de facto language – and more specifically, the global financial services industry is generally conducted in English.
So, if you’re developing a broad chatbot application, English is the obvious starting point. But we’re seeing China coming up to speed in terms of sophistication very, very quickly. They’ve made chatbot technology a national priority. The government is sponsoring it, funding it and has even exhibited control over it following an unexpected embarrassment last summer.
No secret: Chatbots will only get better and more prevalent
Ironically, even though financial services had some of the earliest successful chatbot adopters, the industry as a whole didn’t throw its support behind the technology. That’s because the more successful a project is, the less likely you are to hear about it. For obvious reasons, banks don’t want to tell other banks how to compete against them.
But, as they say, that train has already left the station. Financial services businesses understand that chatbot initiatives are important – and this is increasingly true in other sectors. They also know that achieving scale without increasing overhead is a key business component.
If they aren’t getting involved in this, they’re probably going to be at a disadvantage. Without chatbots, they won’t be able to compete. And without continual research and development into optimizing NLP, AI/ML and other emerging technologies, they won’t have the type of chatbots that will help them compete.
Get the TNW newsletter
Get the most important tech news in your inbox each week.