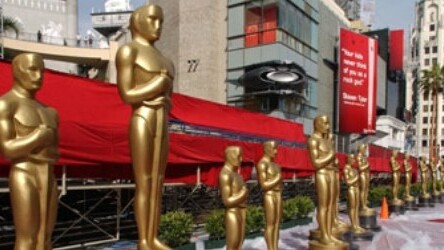
When a company called Meltwater Group released an infographic predicting which Oscar nominees would take home prizes, it was quick to issue a caveat that it wasn’t really making a prediction at all. “The most talked-about nominees are not necessarily going to be the ones who are named the winners by the Academy,” it told Mashable, noting that the company’s methodology simply measured online buzz about the nominees while the Oscars are voted on by a small, select group.
Meltwater is one of several companies that use software to measure what is called social media sentiment analysis. It creates several “fire hoses” of data, pulling thousands of tweets, blog posts, and comments from the social web to measure not only the volume of mentions of a company or person, but also break them down into “positive,” “negative,” and “neutral” sentiments. The idea being that a client can measure its public perception over time and pinpoint when its brand is under attack and, more importantly, why.
But even though Meltwater distanced itself from its Oscars predictions, you can’t blame people for searching for signs that this kind of sentiment analysis can predict future markets (for the record, two out of three of Meltwater’s predictions turned out correct). There is something very alluring about the idea of mining the massive amounts of the data social media users produce every day and using it to foretell the future.
Mining for meaning
In 2010, Science published an article asking, “Can Google Predict the Stock Market?” It detailed the work of several scientists who used Google Trends to detect correlations between search queries and market performance over time. “The Google data could not predict the weekly fluctuations in stock prices,” the article concluded. “However, the team found a strong correlation between Internet searches for a company’s name and its trade volume, the total number of times the stock changed hands over a given week.” Earlier that year, another group tried to use the same idea with millions of tweets, producing similarly murky results.
In both cases, there seemed to be a slight correlation found in hindsight, but no magic formula to actually detect future market fluctuations. Perhaps the most promising example of data mining to predict future trends was when Google began finding flu outbreaks nearly two weeks ahead of federal agencies. By monitoring geographic regions for increases in certain search queries — “flu symptoms,” “where to buy a thermometer,” “flu treatments” — the search giant was able to hone in on these trends much quicker than the doctors who lived within those same regions.
The future of prediction
Will we ever be able to collect a ream of social media data and use it to measure sales of an Apple product before it releases its quarterly earnings reports? Will tweets ever be able to predict who will become president? Late last week I spoke to John Rehling, NLP Expert and Senior Software Engineer for Meltwater Group, about the strengths and weaknesses of the company’s analysis.
“I think in terms of predictions, there are a couple of sources of why if you just look at the raw numbers you can’t get a prediction,” he told me. “One would be who’s talking isn’t necessarily a completely representative sample of the universe. For example, maybe people under 18 will talk about something a bit more while people over 18 spend more money.”
Rehling gave the example of a new car’s introduction to the market. While there may be quite a bit of buzz before the car’s release, it’s the actual experience of driving the car that will determine whether customers purchase it en masse. A simple glitch or overlooked imperfection can make all the pre-release social media buzz irrelevant. “So I think with all these things it will be translated, and people will say, ‘Well in our market, here’s what that buzz means.’ It might mean, ‘Hey, this really is a focus group, and it’s really telling us what sales are going to be like.’ And in another case it might indicate here’s what people will think the week our car comes out, and then after that it’s going to be a matter of the driver experience.”
The engineer said that this kind of analysis is much better at mapping long-term trends. A client can view charts detailing volume of discussion and then break these down into sub-categories that measure the varying levels of negative and positive discussion over time. If there’s an increase in negative sentiment, then you can drill down and see the negative keywords that are triggering this response. Rather than acting as a predictor, the tool allows the company to spot problem areas in its public perception that might not be immediately obvious without this aggregate data.
The limitations of data mining
There are certainly blind spots in the data, however, depending on the client and its demographic. Obviously senior citizens are less represented online than young adults, so a product that caters to them might receive a lower volume of mention. There’s also the fact that the largest social network in the world, Facebook, is at least partially a walled garden. Rehling told me that Meltwater’s software can scan public Facebook pages for comments, but a status update on one of the millions of private walls would be blocked off.
“Volume is also going to be important,” he said. “Depending on how big the business is, it’s going to be across the spectrum from a completely overwhelming volume of conversation to basically none. If it’s a small independent bed and breakfast that has one room and is booked two nights a week, obviously there’s not going to be much on the internet about that.”
One question I had was whether social media users are more likely to write about a negative experience than a positive one. We’ll rarely tweet about the calls that go through, but if our cell phone keeps dropping them then we may feel more motivated to complain. Rehling told me this is why it’s important to monitor long-term trends. By creating a baseline of negative feedback it’s much easier to tell when there’s a sudden spike.
Of course this is a far cry from being able to predict the stock market or even the future sales of a single product. In the meantime, we can derive pleasure from this social media sentiment analysis of popular entertainment. Who needs to know the future price of oil when we can focus on more important things, like who is going to win the next American Idol?
Get the TNW newsletter
Get the most important tech news in your inbox each week.