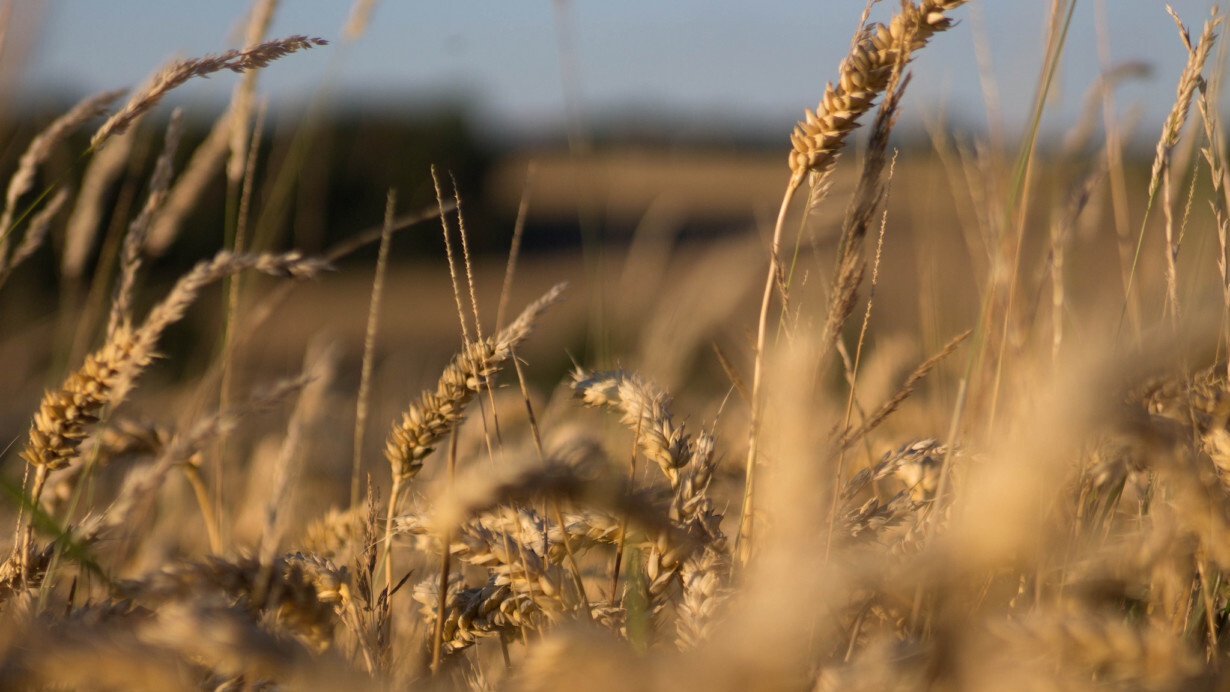
The world is headed toward a major crisis, and it’s not the AI apocalypse. By 2050, the planet’s human population will reach beyond 9 billion, requiring food production to double in order to meet its growing needs. Meanwhile, parameters such as global warming and and the urbanization of life is making it harder to increase crop growth.
While industrial revolutions and technological advances in previous centuries enabled us to tap into (what seemed at the time) the infinite resources of the planet, this time around we’re dealing with a scarcity in land to cultivate and volatility in weather and water.
Technological advances in artificial intelligence and Internet of Things might help us move toward a solution. Here’s how AI can make more efficient and productive use of what resources we have available.
Smart farming
In the past years, artificial intelligence has transformed the way many companies and organizations operate. Today, these algorithms affect a lot of the services you interact with on the internet. This includes Google’s search results, Facebook’s friend suggestions, Amazon’s product suggestions, and more.
The innovation behind these transformations is machine learning, a kind of algorithm that ingests and analyzes tons of data to find common patterns, and turn those patterns into predictions and actions. When applied to agriculture, the same technology can help prevent damage and waste.
The practice, known as “precision farming,” uses real-time and historical data along with machine learning algorithms to take specific actions for smaller areas and time increments instead of performing the same thing for a very large area in a routine-based manner. So, for instance, instead of applying large amounts of pesticide to a vast area, you’ll be able to apply smaller amounts to specific trees, bushes or even leaves, reducing the costs and waste while avoiding damage that the chemical substance can cause when applied in mass.
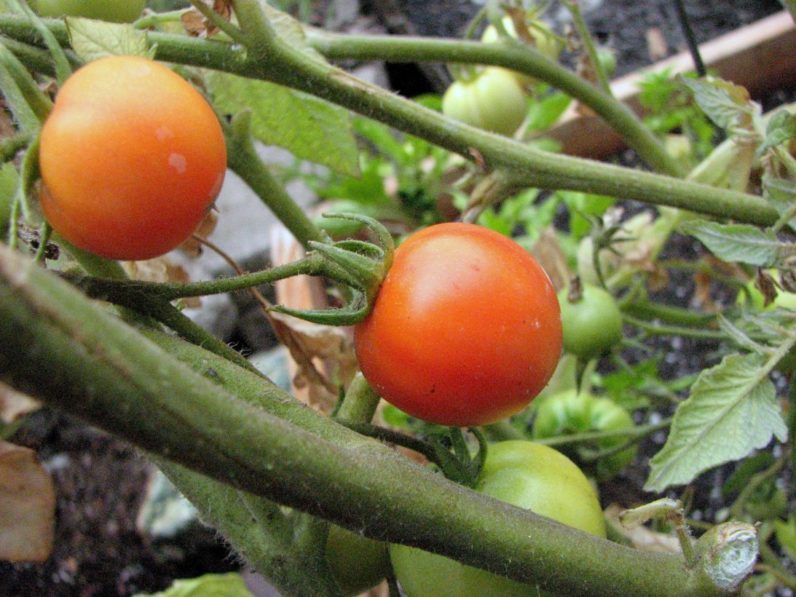
An example of precision farming is Prospera, a company that wants to turn agriculture into a data-driven practice. Prospera uses in-field cameras, sensors and micro weather data to monitor and provide real-time insights about crops. Deep learning and computer vision algorithms analyze the collected data to learn and report when something important is happening. This helps in a number of endeavors such as finding and addressing early signs of diseases to prevent damage and maximize crop yield. One of the benefits of using computer vision to analyze images is that, if well-trained, the algorithms can often see things that go undetected to the eye of trained human experts. A similar approach is being used in medicine for diagnosing cancer.
Arable, another company that works in the precision agriculture domain, uses smart sensors that collect various types of data from the field such as rainfall, humidity, crop water demand, water stress, microclimate data, canopy biomass and chlorophyll. The data can help farmers keep a close eye on their crop and automate decisions and predictions based on real measurements rather than intuition. These tasks can be as easy as controlling irrigation on different parts of the field based on humidity data obtained from sensors, or notifying distribution partners based on the amount and time of yield expected.
Others players in the domain are trying to perform precision farming tasks without the need for hardware. ConserWater uses NASA satellite imagery and weather data along with deep learning algorithms to predict water needs for crops. While the results won’t be as precise as having in-field sensors and cameras, the considerable reduction in costs will lower the entry barrier for farmers with lower budgets.
One of the main challenges in the deployment of AI technologies in farming is the poor connectivity coverage in fields. This can limit the collection of data, which is the meat and potatoes of machine learning algorithms. However, as interest in the field increases and more companies move, these hurdles are being overcome.
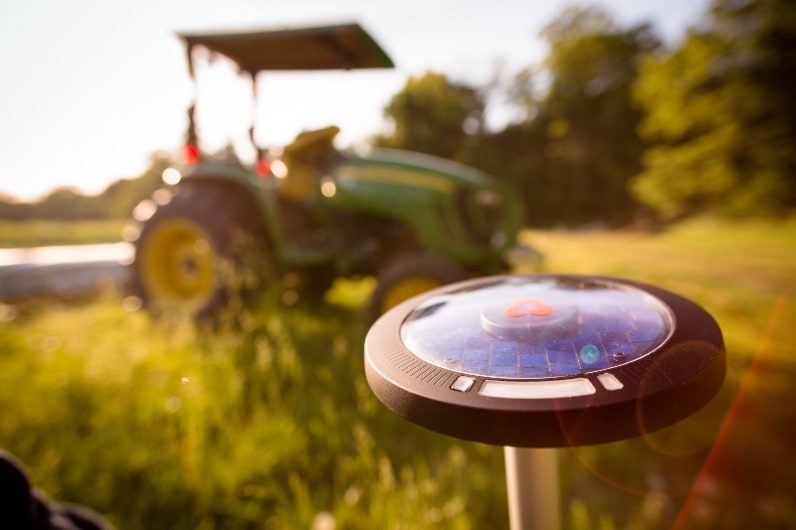
Beyond farms
The field is not the only place where AI can help improve agriculture productivity and reduce waste. In labs and research centers, machine learning algorithms can help in breeding better plant genes, creating safer and more effective crop protection products and fertilizers, and develop other agrichemical products. In fact, these fields are more ripe for AI disruption, since data is more abundant and results can be obtained much quicker.
Also, much of the waste and damage to farming yields can happen during harvesting and transportation. For instance, unsuitable terrain status can result in damage to crops during harvest. Also, the usability of many of the routes that farming products are transported through can be affected by weather conditions such as rain, which will cause delays and detours, both of which can negatively affect the cargo.
This is a problem that soil sensors and weather analytics can help address. Machine learning algorithms can use the data to predict when would be the best time to harvest, taking into account field moisture, upcoming weather conditions and possible impact on routes. Automating and coordinating planting, harvesting and distribution can help ensure that farming yields spend less time traveling between farm to storage facilities, thus reducing damage.
As connectivity becomes more and more ubiquitous, and AI-driven technologies continue to progress, we can expect agriculture to become more automated and data-driven. Whether these innovations and others not covered in this piece will be enough to feed the growing population of the planet is yet to be seen. But for the moment, it seems that AI is our best bet.
Get the TNW newsletter
Get the most important tech news in your inbox each week.