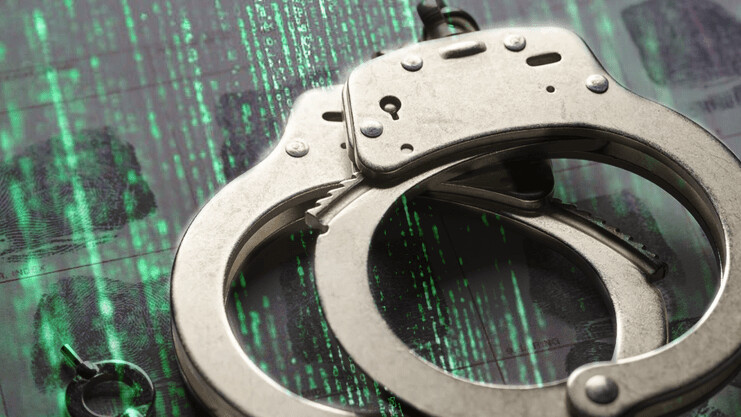
‘Phrenology’ has an old-fashioned ring to it. It sounds like it belongs in a history book, filed somewhere between bloodletting and velocipedes. We’d like to think that judging people’s worth based on the size and shape of their skull is a practice that’s well behind us. However, phrenology is once again rearing its lumpy head.
In recent years, machine-learning algorithms have promised governments and private companies the power to glean all sorts of information from people’s appearance. Several startups now claim to be able to use artificial intelligence (AI) to help employers detect the personality traits of job candidates based on their facial expressions. In China, the government has pioneered the use of surveillance cameras that identify and track ethnic minorities. Meanwhile, reports have emerged of schools installing camera systems that automatically sanction children for not paying attention, based on facial movements and microexpressions such as eyebrow twitches.
Perhaps most notoriously, a few years ago, AI researchers Xiaolin Wu and Xi Zhang claimed to have trained an algorithm to identify criminals based on the shape of their faces, with an accuracy of 89.5 per cent. They didn’t go so far as to endorse some of the ideas about physiognomy and character that circulated in the 19th century, notably from the work of the Italian criminologist Cesare Lombroso: that criminals are underevolved, subhuman beasts, recognisable from their sloping foreheads and hawk-like noses.
However, the recent study’s seemingly high-tech attempt to pick out facial features associated with criminality borrows directly from the ‘photographic composite method’ developed by the Victorian jack-of-all-trades Francis Galton – which involved overlaying the faces of multiple people in a certain category to find the features indicative of qualities like health, disease, beauty and criminality.
Technology commentators have panned these facial-recognition technologies as ‘literal phrenology’; they’ve also linked it to eugenics, the pseudoscience of improving the human race by encouraging people deemed the fittest to reproduce. (Galton himself coined the term ‘eugenics’, describing it in 1883 as ‘all influences that tend in however remote a degree to give to the more suitable races or strains of blood a better chance of prevailing speedily over the less suitable than they otherwise would have had’.)
In some cases, the explicit goal of these technologies is to deny opportunities to those deemed unfit; in others, it might not be the goal, but it’s a predictable result. Yet when we dismiss algorithms by labelling them as phrenology, what exactly is the problem we’re trying to point out? Are we saying that these methods are scientifically flawed and that they don’t really work – or are we saying that it’s morally wrong to use them regardless?
There is a long and tangled history to the way ‘phrenology’ has been used as a withering insult. Philosophical and scientific criticisms of the endeavour have always been intertwined, though their entanglement has changed over time.
In the 19th century, phrenology’s detractors objected to the fact that phrenology attempted to pinpoint the location of different mental functions in different parts of the brain – a move that was seen as heretical, since it called into question Christian ideas about the unity of the soul. Interestingly, though, trying to discover a person’s character and intellect based on the size and shape of their head wasn’t perceived as a serious moral issue.
Today, by contrast, the idea of localising mental functions is fairly uncontroversial. Scientists might no longer think that destructiveness is seated above the right ear, but the notion that cognitive functions can be localised in particular brain circuits is a standard assumption in mainstream neuroscience.
Phrenology had its share of empirical criticism in the 19th century, too. Debates raged about which functions resided where, and whether skull measurements were a reliable way of determining what’s going on in the brain. The most influential empirical criticism of old phrenology, though, came from the French physician Jean Pierre Flourens’s studies based on damaging the brains of rabbits and pigeons – from which he concluded that mental functions are distributed, rather than localised. (These results were later discredited.) The fact that phrenology was rejected for reasons that most contemporary observers would no longer accept makes it only more difficult to figure out what we’re targeting when we use ‘phrenology’ as a slur today.
Both ‘old’ and ‘new’ phrenology have been critiqued for their sloppy methods. In the recent AI study of criminality, the data were taken from two very different sources: mugshots of convicts, versus pictures from work websites for nonconvicts. That fact alone could account for the algorithm’s ability to detect a difference between the groups. In a new preface to the paper, the researchers also admitted that taking court convictions as synonymous with criminality was a ‘serious oversight’.
Yet equating convictions with criminality seems to register with the authors mainly as an empirical flaw: using mugshots of convicted criminals, but not of the ones who got away introduces a statistical bias. They said they were ‘deeply baffled’ at the public outrage in reaction to a paper that was intended ‘for pure academic discussions’.

Notably, the researchers don’t comment on the fact that conviction itself depends on the impressions that police, judges and juries form of the suspect – making a person’s ‘criminal’ appearance a confounding variable. They also fail to mention how the intense policing of particular communities, and inequality of access to legal representation, skews the dataset.
In their response to criticism, the authors don’t back down on the assumption that ‘being a criminal requires a host of abnormal (outlier) personal traits’. Indeed, their framing suggests that criminality is an innate characteristic, rather than a response to social conditions such as poverty or abuse. Part of what makes their dataset questionable on empirical grounds is that who gets labelled ‘criminal’ is hardly value-neutral.
One of the strongest moral objections to using facial recognition to detect criminality is that it stigmatises people who are already overpoliced. The authors say that their tool should not be used in law-enforcement, but cite only statistical arguments about why it ought not to be deployed. They note that the false-positive rate (50 per cent) would be very high, but take no notice of what that means in human terms. Those false positives would be individuals whose faces resemble people who have been convicted in the past. Given the racial and other biases that exist in the criminal justice system, such algorithms would end up overestimating criminality among marginalised communities.
The most contentious question seems to be whether reinventing physiognomy is fair game for the purposes of ‘pure academic discussion’. One could object on empirical grounds: eugenicists of the past such as Galton and Lombroso ultimately failed to find facial features that predisposed a person to criminality. That’s because there are no such connections to be found. Likewise, psychologists studying the heritability of intelligence, such as Cyril Burt and Philippe Rushton, had to play fast and loose with their data to manufacture correlations between skull size, race and IQ. If there were anything to discover, presumably the many people who have tried over the years wouldn’t have come up dry.
The problem with reinventing physiognomy is not merely that it has been tried without success before. Researchers who persist in looking for cold fusion after the scientific consensus has moved on also face criticism for chasing unicorns – but disapproval of cold fusion falls far short of opprobrium. At worst, they are seen as wasting their time. The difference is that the potential harms of cold fusion research are much more limited. In contrast, some commentators argue that facial recognition should be regulated as tightly as plutonium, because it has so few nonharmful uses.
When the dead-end project you want to resurrect was invented for the purpose of propping up colonial and class structures – and when the only thing it’s capable of measuring is the racism inherent in those structures – it’s hard to justify trying it one more time, just for curiosity’s sake.
However, calling facial-recognition research ‘phrenology’ without explaining what is at stake probably isn’t the most effective strategy for communicating the force of the complaint. For scientists to take their moral responsibilities seriously, they need to be aware of the harms that might result from their research. Spelling out more clearly what’s wrong with the work labelled ‘phrenology’ will hopefully have more of an impact than simply throwing the name around as an insult.
This article was originally published at Aeon by Catherine Stinson and has been republished under Creative Commons.
Get the TNW newsletter
Get the most important tech news in your inbox each week.