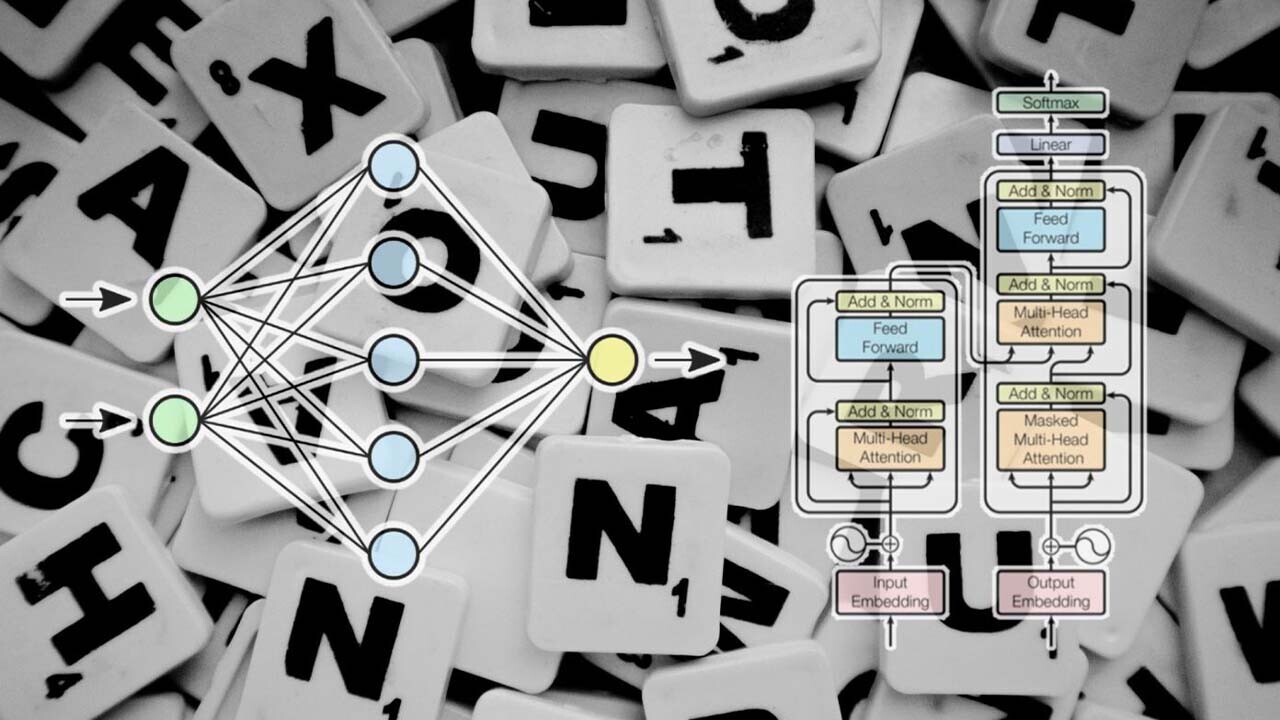
This article is part of “the philosophy of artificial intelligence,” a series of posts that explore the ethical, moral, and social implications of AI today and in the future.
If a computer gives you all the right answers, does it mean that it is understanding the world as you do? This is a riddle that artificial intelligence scientists have been debating for decades. And discussions of understanding, consciousness, and true intelligence are resurfacing as deep neural networks have spurred impressive advances in language-related tasks.
Many scientists believe that deep learning models are just large statistical machines that map inputs to outputs in complex and remarkable ways. Deep neural networks might be able to produce lengthy stretches of coherent text, but they don’t understand abstract and concrete concepts in the way that humans do.
Other scientists beg to differ. In a lengthy essay on Medium, Blaise Aguera y Arcas, an AI scientist at Google Research, argues that large language models—deep learning models that have been trained on very large corpora of text—have a great deal to teach us about “the nature of language, understanding, intelligence, sociality, and personhood.”
Large language models
Large language models have gained popularity in recent years thanks to the convergence of several elements:
1-Availability of data: There are enormous bodies of online text such as Wikipedia, news websites, and social media that can be used to train deep learning models for language tasks.
2-Availability of compute resources: Large language models comprise hundreds of billions of parameters and require expensive computational resources for training. As companies such as Google, Microsoft, and Facebook have become interested in the applications of deep learning and large language models, they have invested billions of dollars into research and development in the field.
3-Advances in deep learning algorithms: Transformers, a deep learning architecture that was introduced in 2017, has been at the heart of recent advances in natural language processing and generation (NLP/NLG).
One of the great advantages of Transformers is that they can be trained through unsupervised learning on very corpora of unlabeled text. Basically, what a Transformer does is take a string of letters (or another type of data) as input and predict the next letters in the sequence. It can be a question followed by the answer, a headline followed by an article, or a prompt by a user in a chat conversation.
Recurrent neural networks (RNN) and long short-term memory networks (LSTM), the predecessors to Transformers, were notoriously bad at maintaining their coherence over long sequences. But Transformer-based language models such as GPT-3 have shown impressive performance in article-length output, and they are less prone to the logical mistakes that other types of deep learning architectures make (though they still have their own struggles with basic facts). Moreover, recent years have shown that the performance of language models improves with the size of the neural network and training dataset.
In his essay, Aguera y Arcas explores the potential of large language models through conversations with LaMDA, an improved version of Google’s Meena chatbot.
Aguera y Arcas shows through various examples that LaMDA seems to handle abstract topics such as social relationships and questions that require intuitive knowledge about how the world works. For example, if you tell it “I dropped the bowling ball on the bottle and it broke,” it shows in subsequent exchanges that it knows that the bowling ball broke the bottle. You might guess that the language model will associate “it” with the second noun in the phrase. But then Aguera y Arcas makes a subtle change to the sentence and writes, “I dropped the violin on the bowling ball and it broke,” and this time, LaMDA associates “it” with violin, the lighter and more fragile object.
Other examples show the deep learning model engaging in imaginary conversations, such as what is its favorite island, even though it doesn’t even have a body to physically travel and experience the island. It can talk extensively about its favorite smell, even though it doesn’t have an olfactory system to experience smell.
Does AI need a sensory experience?
In his article, Aguera y Arcas refutes some of the key arguments that are being made against understanding in large language models.
One of these arguments is the need for embodiment. If an AI system doesn’t have a physical presence and cannot sense the world in a multimodal system as humans do, then its understanding of human language is incomplete. This is a valid argument. Long before children learn to speak, they develop complicated sensing skills. They learn to detect people, faces, expressions, objects. They learn about space, time, and intuitive physics. They learn to touch and feel objects, smell, hear, and create associations between different sensory inputs. And they have innate skills that help them navigate the world. Children also develop “theory of mind” skills, where they can think about the experience that another person or animal is having, even before they learn to speak. Language builds on top of all this innate and obtained knowledge and the rich sensory experience that we have.
But Aguera y Arcas argues, “Because learning is so fundamental to what brains do, we can, within broad parameters, learn to use whatever we need to. The same is true of our senses, which ought to make us reassess whether any particular sensory modality is essential for rendering a concept ‘real’— even if we intuitively consider such a concept tightly bound to a particular sense or sensory experience.”
And then he brings examples from the experiences of blind and deaf people, including the famous 1929 essay by Helen Keller, who was born both blind and deaf, titled, “I Am Blind — Yet I see; I Am Deaf — Yet I Hear”:
“I have a color scheme that is my own… Pink makes me think of a baby’s cheek, or a gentle southern breeze. Lilac, which is my teacher’s favorite color, makes me think of faces I have loved and kissed. There are two kinds of red for me. One is the red of warm blood in a healthy body; the other is the red of hell and hate.”
From this, Aguera y Arcas concludes that language can help fill the sensory gap between humans and AI.
“While LaMDA has neither a nose nor an a priori favorite smell (just as it has no favorite island, until forced to pick one), it does have its own rich skein of associations, based, like Keller’s sense of color, on language, and through language, on the experiences of others,” he writes.
Aguera y Arcas further argues that thanks to language, we have access to socially learned aspects of perception that make our experience far richer than raw sensory experience.
Sequence learning
In his essay, Aguera y Arcas argues that sequence learning is the key to all the complex capabilities that are associated with big-brained animals—especially humans—including reasoning, social learning, theory of mind, and consciousness.
“As anticlimactic as it sounds, complex sequence learning may be the key that unlocks all the rest. This would explain the surprising capacities we see in large language models — which, in the end, are nothing but complex sequence learners,” Aguera y Arcas writes. “Attention, in turn, has proven to be the key mechanism for achieving complex sequence learning in neural nets — as suggested by the title of the paper introducing the Transformer model whose successors power today’s LLMs: Attention is all you need.”
This is an interesting argument because sequence learning is in fact one of the fascinating capacities of organisms with higher-order brains. This is nowhere more evident than in humans, where we can learn very long sequences of actions that yield long-term rewards.
And he’s also right about sequence learning in large language models. At their core, these neural networks are made to map one sequence to another, and the bigger they get, the longer the sequences they can read and generate. And the key innovation behind Transformers is the attention mechanism, which helps the model focus on the most important parts of its input and output sequences. These attention mechanisms help the Transformers handle very large sequences with much less memory requirements than their predecessors.
Are we just a jumble of neurons?
While artificial neural networks are working on a different substrate than their biological counterparts, they are in effect performing the same kind of functions, Aguera y Arcas argues in his essay. Even the most complicated brain and nervous system are composed of simple components that collectively create the intelligent behavior that we see in humans and animals. Aguera y Arcas describes intelligent thought as “a mosaic of simple operations” that, when studied up close, disappear into its mechanical parts.
Of course, the brain is so complex that we don’t have the capacity to understand how every single component works by itself and in connection with others. And even if we ever do, some of its mysteries will probably continue to elude us. And the same can be said of large language models, Aguera y Arcas says.
“In the case of LaMDA, there’s no mystery as to how the machine works at a mechanical level, in that the whole program can be written in a few hundred lines of code; but this clearly doesn’t confer the kind of understanding that demystifies interactions with LaMDA. It remains surprising to its own makers, just as we’ll remain surprising to each other even when there’s nothing left to learn about neuroscience,” he writes.
From here, he concludes that it is unfair to dismiss language models as not intelligent because they are not conscious like humans and animals. What we consider “consciousness” and “agency” in humans and animals, Aguera y Arcas argues, is in fact the mysterious parts of the brain and nervous system that we don’t yet understand.
“Like a person, LaMDA can surprise us, and that element of surprise is necessary to support our impression of personhood. What we refer to as ‘free will’ or ‘agency’ is precisely this necessary gap in understanding between our mental model (which we could call psychology) and the zillion things actually taking place at the mechanistic level (which we could call computation). Such is the source of our belief in our own free will, too,” he writes.
So basically, while large language models don’t work like the human brain, it is fair to say that they have their own kind of understanding of the world, purely through the lens of word sequences and their relations with each other.
Counterarguments
Melanie Mitchell, Davis Professor of Complexity at the Santa Fe Institute, provides interesting counterarguments to Aguera y Arcas’s article in a short thread on Twitter.
I read this article by @blaiseaguera with great interest.
It would take a while to give a complete response to Blaise's arguments, but here are some of my (stream of consciousness) thoughts. ? https://t.co/yYkmkStXPz
— Melanie Mitchell (@MelMitchell1) December 18, 2021
While Mitchell agrees that machines may one day understand language, current deep learning models such as LaMDA and GPT-3 are far from that level.
Last year, Mitchell wrote a paper in AI Magazine on the struggles of AI to understand situations. More recently, she wrote an essay in Quanta Magazine that explores the challenges of measuring understanding in AI.
“The crux of the problem, in my view, is that understanding language requires understanding the world, and a machine exposed only to language cannot gain such an understanding,” Mitchell writes.
Mitchell argues that when humans process language, they use a lot of knowledge that is not explicitly written down in the text. So, there’s no way for AI to understand our language without being endowed with that kind of infrastructural knowledge. Other AI and linguistics experts have made similar arguments on the limits of pure neural network–based systems that try to understand language through text alone.
Mitchell also argues that contrary to Aguera y Arcas’s argument, the quote from Hellen Keller proves that sensory experience and embodiment are in fact important to language understanding.
“[To] me, the Keller quote shows how embodied her understanding of color is — she maps color concepts to odors, tactile sensations, temperature, etc.,” Mitchell writes.
As for attention, Mitchell says that “attention” in neural networks as mentioned in Aguera y Acras’s article is very different from what we know about attention in human cognition, a point that she has elaborated on in a recent paper titled “Why AI is Harder Than We Think.”
But Mitchell commends Aguera y Acras’s article as “thought-provoking” and underlines that the topic is important, especially “as companies like Google and Microsoft deploy their [large language models] more and more into our lives.”
This article was originally published by Ben Dickson on TechTalks, a publication that examines trends in technology, how they affect the way we live and do business, and the problems they solve. But we also discuss the evil side of technology, the darker implications of new tech, and what we need to look out for. You can read the original article here.
Get the TNW newsletter
Get the most important tech news in your inbox each week.