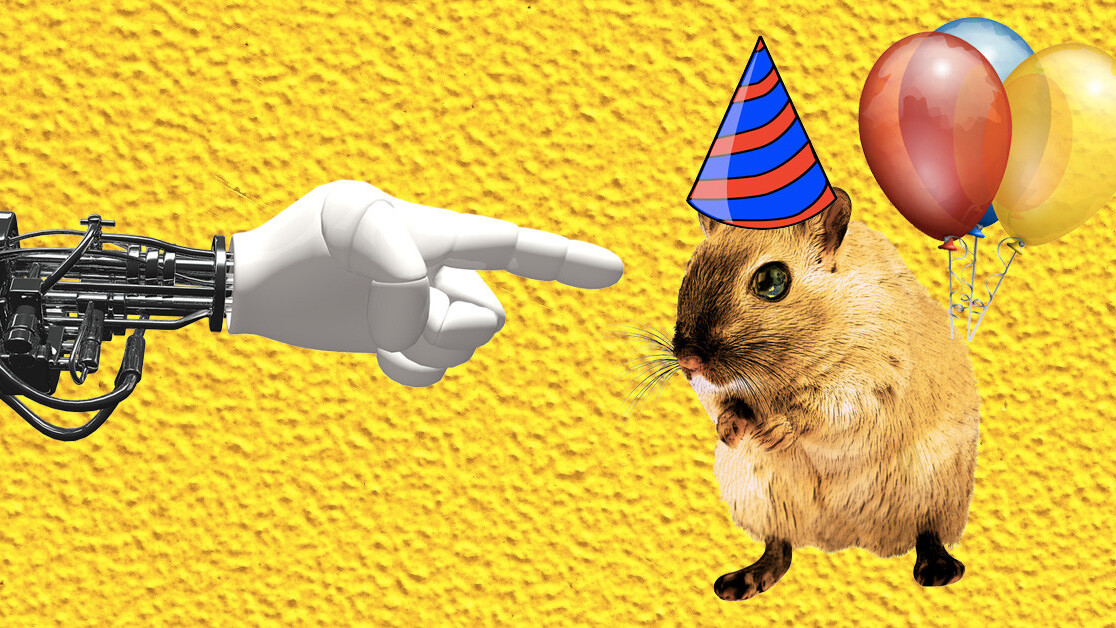
As cold-blooded and inhuman as it may sound, animal tests are an integral part of modern-day drug and chemical compounds development and approval procedures. And with good reasons. Scientists can’t still reliably predict the properties of new chemicals, let alone how these compounds might interact with living cells.
But a new paper published in the research journal Toxicological Sciences shows that it is possible to predict the attributes of new compounds using the data we already have about past tests and experiments. The artificially intelligent system was trained to predict the toxicity of tens of thousands of unknown chemicals, based on previous animal tests, and the results are, in some cases, more accurate and reliable than real animal tests.
Using AI in the drug development process is nothing new. In fact with 28 pharma companies and 93 startups already spending hundreds of millions to apply machine learning and other AI techniques to drug discovery, the costly and time consuming process of identifying and testing new drugs, it seems that the industry is ripe for an artificially intelligent disruption.
According to Andrew Hopkins, CEO of Exscientia, artificial intelligence makes “better designs and better decisions about what compounds to make and test”, ultimately leading to fewer experiments and “fewer experiments means you’re saving time and money.”
He continues that people think we can’t use AI in this field because biology is complex and messy, “but it’s precisely because of the complexity of the decision-making that we should use AI. For example, Bayesian approaches are particularly applicable to messy data, where you can embrace uncertainty in the data. AI doesn’t require perfect data for perfect predictions. It’s actually about how you use it in these imperfect, messy, complicated situations to find a signal amid all the noise.”
Thomas Hartung, the toxicologist at Johns Hopkins University in Baltimore, Maryland, who leads the research to predict drug properties without live animal tests says computer models could replace some standard safety studies conducted on millions of animals each year, such as dropping compounds into rabbits’ eyes to check if they are irritants, or feeding chemicals to rats to work out lethal doses. “The power of big data means we can produce a tool more predictive than many animal tests.”
His team made this predictive approach possible through feeding a vast amount of data to their AI that was harnessed from a huge dataset that was originally collected by the European Chemicals Agency (ECHA) under a 2007 law called REACH (registration, evaluation, authorization and restriction of chemicals).
The data gathered in ECHA’s database is publicly available but the format is not easily readable for computers. In 2014, Hartung’s crew started to reformat the data in a way that is easily feedable to machines, compromising information about 10 thousand chemicals and their properties that was gathered in roughly 800 thousand animal tests.
And the results are amazing. Their system is able to predict the toxicity for tens of thousands of chemicals covering nine types of tests, covering everything from harm to aquatic ecosystems to inhalation damage.
Bringing animal tests in drug development to a minimum is not only a noble cause in light of animal rights and humanity, but makes the drug development process way shorter and less expensive, sparing researchers much of the bulk tests that are currently carried out on animals. In February, the Interagency Coordinating Committee on the Validation of Alternative Methods (ICCVAM), which represents 16 US federal agencies’ combined efforts to develop methods to replace animal tests, came up with a strategic roadmap for replacing animal use in toxicity testing.
In April, ICCVAM invited research groups and academics to the National Institutes of Health in Bethesda, Maryland to let each group show how their software is capable of predicting the toxicity of 40 thousand chemicals already tested on animals.
Combining the prediction of these methods, that also included Hartung’s artificial intelligence, leads to results that are “as well as the animal tests”, according to Nicole Kleinstreuer, Deputy Director of the NTP Interagency Center for the Evaluation of Alternative Toxicological Methods, who also leads NICEATM’s computational toxicology work.
While computer systems and artificial intelligence seem ripe to gradually enter and replace a vast majority of standard safety tests that are annually carried out on animals, there are more complex and long-term effect tests like a drug’s impact on fertility or increase in cancer. Nonetheless, the prospects have never been looking better for animal rights activists on the one hand and consumers on the other to reap the benefits of artificial intelligence and machine learning in yet another field.
This story is republished from TechTalks, the blog that explores how technology is solving problems… and creating new ones. Like them on Facebook here and follow them down here:
Get the TNW newsletter
Get the most important tech news in your inbox each week.