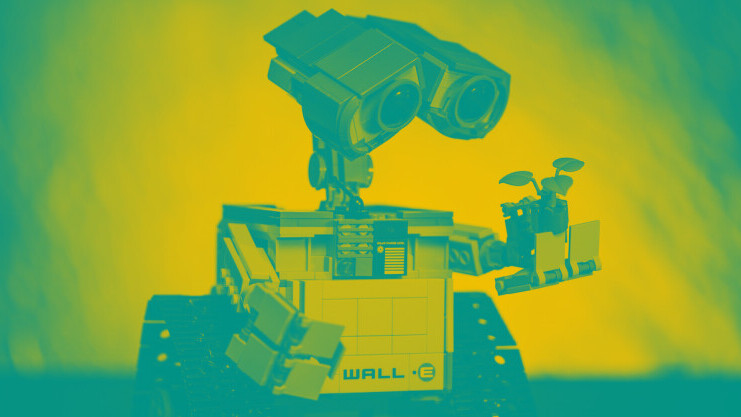
With their competitive advantage in emerging technologies like AI and machine learning, big tech companies have been moving into and disrupting traditional industries, from Airbnb’s impact on the hospitality industry to Uber’s upending of taxi and other mobility services. Now big tech has its sights set on the world of finance with the launch of Google Pay, the Apple Card, Facebook’s Libra, and Amazon’s loans for SMEs.
But, while these technologies could help big tech gain an advantage in countering the ever evolving threat from cybercriminals, detecting fraud, and automating processes like loan applications and credit checks, AI ethics could also become one of its biggest challenges.
Much like deeply flawed police profiling tools, biased AI algorithms can also skew results. For instance, in 2019, the algorithm used by Apple Card was found to be biased against women after determining the “creditworthiness” of applicants.
But it’s not just bias, highly sensitive financial data will add a new dimension to the data collection vs user privacy debate already ongoing within the EU. In fact, in June of this year the EU Court of Justice backed a ruling that will give national privacy watchdogs more room to scrutinize big tech across the bloc.
Having long faced heavy regulation, what can traditional banks teach these new players about building AI capabilities that are fair, ethical, and regulator approved?
It takes more than lip service to tackle bias in AI
Cybercriminals are continuing to evolve their techniques and their attack methods. While AI is becoming increasingly important in our day-to-day lives, such as detecting transactions that differ from normal customer behavior, there are still challenges.
“Bias is one of the new challenges of AI,” says Mark Wiggerman, a data scientist at ABN AMRO’s corporate information security office.
The problem with bias is that it’s hidden; it’s not very clear how the AI models learn from data and make new predictions. So explainability is harder as you must do something to explain the decisions of your own model.
The functionality of the decision system is determined by the training data Wiggerman explains, adding that a model will often have to be retrained, which, in turn, could also lead to new functionality and possibly new biases.
You must continuously monitor it to see if it’s within bounds of the applicable privacy framework. It’s not only about making a different decision for different groups, it’s also about what impact that has on people and how that can be balanced with the potential benefits.
An example (fictitious) of a negative impact could be a fraud detection system that (unintentionally) puts transactions from young people on a queue for manual inspection. The negative impact is that their transactions are processed a few hours later, and the negative impact is structural for that group.
A lot of biased AI systems have been reactive rather than proactive. The key to tackling ethical AI issues is to. not only monitor your systems regularly, but also to have clear guidelines on what actually constitutes ethical AI for your organization. Wiggerman shared:
I think big techs together with non-commercial organizations are taking some positive steps forward when it comes to tackling bias. For example, reusable software packages like AIF360 enable you to measure bias.
Like other companies, ABN AMRO is busy assessing AI and the potential it can deliver to its customers and staff. At the same time it’s developing policies to ensure fairness in AI applications.
Gathering personal data
Big tech companies such as Amazon, Facebook, and Google are incredibly powerful and popular organizations, particularly when it comes to gathering personal data. According to recent findings, Google takes the prize in storing the most personal data, whereas the best company for privacy is Apple. Twitter and Facebook were found to store more data than they need to, but with Facebook this was down to data users had entered themselves.
Because of the favored position that big tech companies have, they’re able to leverage the data they already have on consumers. Yet, it’s this collection of data that brings into question consumer privacy, particularly when it comes to financial services.
Wiggerman questions whether it’s okay for big tech to process the type of data they’re gathering.
Do they need the data to make their product work? Could they do it with less data? These questions are also relevant for machine learning. I can put in your exact GPS location and predict your movements. But do I really need this information for my product to detect fraud? Maybe I only need to know whether you’re in the Netherlands or in Belgium.
Only collecting necessary data (which is part of the GDPR by the way), is something that big tech needs to take into account. Bias shouldn’t be the only focus, but it should be part of a wider, responsible AI proposition which takes into account different countries’ regulations. For instance, regulations in the US are different from those in Europe, although according to Wiggerman the US is also introducing more GDPR-like rulings than before.
Privacy, ethics, and fairness should be added to the product development life cycle from the beginning. In other words, fairness and privacy by design. This isn’t something that should be added afterwards. Consider security by design. Security measures shouldn’t be added afterwards, but taken into account from the beginning. AI ethics needs to trickle down into all the risk policies of any company.
Working together
Although big tech is starting to offer more financial services, one of the drawbacks is that they don’t have the historical financial data that the traditional banking sector enjoys. They also lack experience with financial processing. Wiggerman suggests that big tech organizations work with banks to learn from their financial history, as well as understand how they comply with GDPR models or audit requirements.
Of course, if they want to become a bank, they must comply with all of the rules and regulations. But I think if you want to develop good products, you need a lot of financial history such as transaction data, information about how customers are onboarded, and how they use financial services (loans, mortgages, etc.) and big tech just doesn’t have it yet.
When it comes to reconciling security and customer privacy, Wiggerman is of the view that the two can come together. “Definitely,” he says.
I think they can be combined, especially with the new type of technologies that are emerging from academics called privacy enhancing technologies (PET). I’m a big fan of a relatively new technology called multi-party computation (MPC), which is being used to do joint calculations on encrypted data.
If you have two companies that have data they can’t share, like banks, MPC lets them share the data in an encrypted way so that the receiver can’t understand the data. However, MPC allows you to do a joint computation on all of the [encrypted] data of the three or four banks that want to collaborate. The outcome of this computation is usable for each bank but the raw data remains secret. In this way it allows you to maintain user privacy.
In March, it was reported that ABN AMBRO was collaborating with Dutch scientific research organisation TNO and Dutch financial services company Rabobank to work on an MPC project designed to share and analyze data to detect financial crime.
ABN AMRO doesn’t publish any of its anti-crime efforts; however, Wiggerman explains that there is a strong collaboration between banks when it comes to cybersecurity. When something happens, information is shared between banking services rather than treating security as a competitive area. This is where big tech companies may differ.
When we think of big tech, we often think about large organizations that deliver social media platforms, ecommerce sites, or telecommunications. We don’t automatically think about AI and financial services. Given Google’s YouTube algorithms and Amazon’s Alexa, it’s clear that big tech is being driven by AI and machine learning.
It should come as no surprise then that these same companies are turning to financial services, which is swiftly changing the banking sector. But in order for big tech to truly enter the industry, a collaboration between the two may be the way forward.
Get the TNW newsletter
Get the most important tech news in your inbox each week.