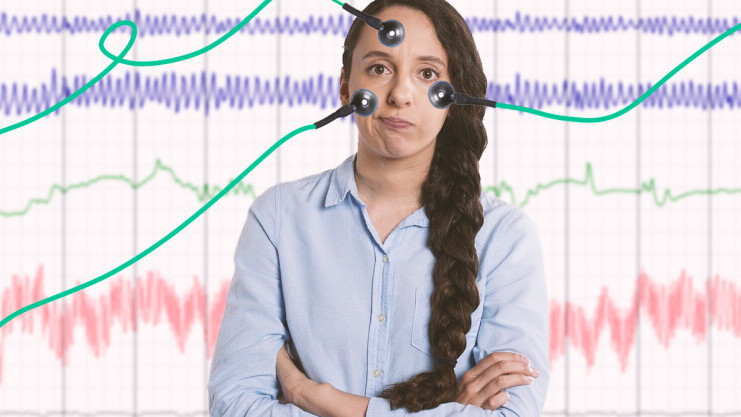
Another day, another problematic AI study. Today’s snake oil special comes via Tel Aviv University where a team of researchers have unveiled a so-called “lie-detection system.”
Let’s be really clear right up front: AI can’t do anything a person, given an equivalent amount of time to work on the problem, couldn’t do themselves. And no human can tell if any given human is lying. Full stop.
The simple fact of the matter is that some of us can tell when some people are lying some of the time. Nobody can tell when anybody is lying all of the time.
The university makes the following claim via press release:
Researchers at Tel Aviv University detected 73% of the lies told by trial participants based on the contraction of their facial muscles – achieving a higher rate of detection than any known method.
That’s a really weird statement. The idea that “73%” accuracy at detecting lies is indicative of a particular paradigm’s success is arguable at best.
What exactly is accuracy?
Base luck gives any system capable of choice a 50/50 chance. And, traditionally, that’s about how well humans perform at guessing lies. Interestingly, they perform much better at guessing truths. Some studies claim humans achieve about the same “accuracy” at determining truth statements as the Tel Aviv team’s “lie-detection system” does at determining truthfulness.
The Tel Aviv University team’s paper even mentions that polygraphs aren’t admissible in courts because they’re unreliable. But they fail to point out that polygraph devices (which have been around since 1921) beat their own system in so-called “accuracy” — polygraphs average about an 80% – 90% accuracy-rate in studies.
But let’s take a deeper look at the Tel Aviv team’s study anyway. The team started with 48 participants, 35 of which were identified as “female.” Six participants were cut because of technical issues, two got dropped for “never lying,” and one participated in “only 40 out of 80 trials when monetary incentives were not presented.”
So, the data for this study was generated from two sources: a proprietary AI system and 39-40 human participants. Of those participants, an overwhelming majority were identified as “female,” and there’s no mention of racial, cultural, or religious diversity.
Furthermore, the median age of participants was 23 and there’s no way to determine if the team considered financial backgrounds, mental health, or any other concerns.
All we can tell is that a small group of people averaging 23-years in age, mostly “female,” paired off to participate in this study.
There was also compensation involved. Not only were they paid for their time, which is standard in the world of academia research, but they were also paid for successfully lying to humans.
That’s a red flag. Not because it’s unethical to pay for study data (it isn’t). But because it’s adding unnecessary parameters in order to intentionally or ignorantly muddy up the study.
The researchers explain this by claiming it was part of the experiment to determine whether incentivization changed people’s ability to lie.
But, with such a tiny study sample, it seems ludicrous to cram the experiment full of needless parameters. Especially ones that are so half-baked they couldn’t possibly be codified without solid background data.
How much impact does a financial incentive have on the efficacy of a truth-telling study? That sounds like something that needs its own large-scale study to determine.
Let’s just move on to the methodology
The researchers paired off participants into liars and receivers. The liars put on headphones and listened for either the word “tree” or “line” and then were directed to either tell the truth or lie about which they’d heard. Their partner’s job was to guess if they were being lied to.
The twist here is that the researchers created their own electrode arrays and attached them to the liars’ faces and then developed an AI to interpret the outputs. The researchers operated under an initial assumption that twitches in our facial muscles are a window to the ground-truth.
This assumption is purely theoretical and, frankly, ridiculous. Stroke victims exist. Bell’s Palsy exists. Neurodiverse communication exists. Scars and loss of muscle strength exist. At least 1 billion people in the world currently live with some form of physical disability and nearly as many live with a diagnosed mental disorder.
Yet, the researchers expect us to believe they’ve invented a one-size-fits-all algorithm for understanding humans. They’re claiming they’ve stumbled across a human trait that inextricably links the mental act of deceit with a singular universal physical expression. And they accomplished this by measuring muscle twitches in the faces of just 40 humans?
Per the aforementioned press release:
The researchers believe that their results can have dramatic implications in many spheres of our lives. In the future, the electrodes may become redundant, with video software trained to identify lies based on the actual movements of facial muscles.
So the big idea here is to generate data with one experimental paradigm (physical electrodes) in order to develop a methodology for a completely different experimental paradigm (computer vision)? And we’re supposed to believe that this particular mashup of disparate inputs will result in a system that can determine a human’s truthfulness to such a degree that its outputs are admissible in court?
That’s a bold leap to make! The team may as well be claiming it’s solved AGI with black box deep learning. Computer vision already exists. Either the data from the electrodes is necessary or it isn’t.
What’s worse, they apparently intend to develop this into a snake oil solution for governments and big businesses.
The press release continues with a quote:
[Team member Dino Levy] predicts: “In the bank, in police interrogations, at the airport, or in online job interviews, high-resolution cameras trained to identify movements of facial muscles will be able to tell truthful statements from lies. Right now, our team’s task is to complete the experimental stage, train our algorithms and do away with the electrodes. Once the technology has been perfected, we expect it to have numerous, highly diverse applications.”
Police interrogations? Airports? What?
Exactly what percentage of those 40 study participants were Black, Latino, disabled, autistic, or queer? How can anyone, in good faith and conscience, make such grandiose scientific claims about AI based on such a tiny sprinkling of data?
If this “AI solution” were to actually become a product, people could potentially be falsely arrested, detained at airports, denied loans, and passed over for jobs because they don’t look, sound, and act exactly like the people who participated in that study.
This AI system was only able to determine whether someone was lying with a 73% level of accuracy in an experiment where the lies were only one word long, meant nothing to the person saying them, and had no real effect on the person hearing them.
There’s no real-world scenario analogous to this experiment. And that “73% accuracy” is as meaningless as a Tarot card spread or a Magic 8-Ball’s output.
Simply put: A 73% accuracy rate over less than 200 iterations of a study involving a maximum of 20 data groups (the participants were paired off) is a conclusion that indicates your experiment is a failure.
The world needs more research like this, don’t get me wrong. It’s important to test the boundaries of technology. But the claims made by the researchers are entirely outlandish and clearly aimed at an eventual product launch.
Sadly, there’s about a 100% chance that this gets developed and ends up in use by US police officers.
Just like predictive-policing, Gaydar, hiring AI, and all the other snake oil AI solutions out there, this is absolutely harmful.
But, by all means, don’t take my word for it: read the entire paper and the researchers’ own conclusions here.
Get the TNW newsletter
Get the most important tech news in your inbox each week.