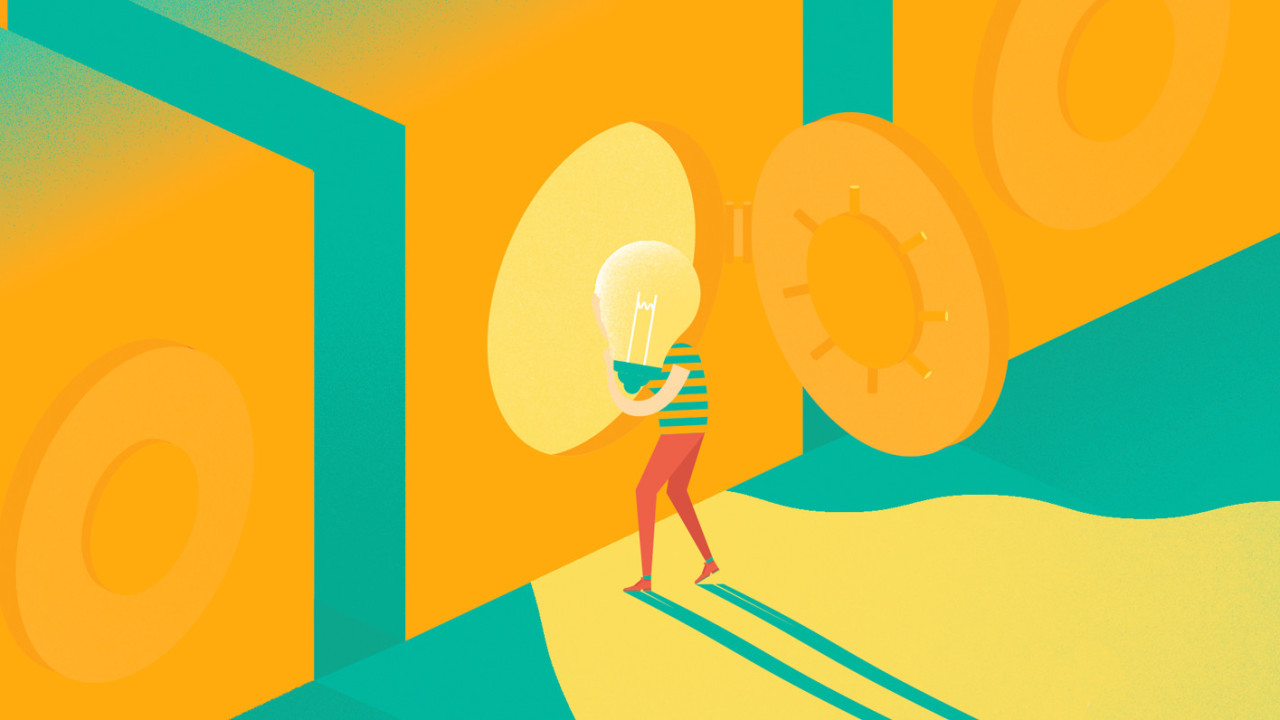
What if you had a personal banking bot that warns you when your funds are getting low? Or that you usually buy flowers at a certain time of the year for your mother’s birthday and that you haven’t this year? With cognitive computing, this scenario might soon become a reality. Cognitive computing broadly refers to technology platforms which leverage artificial intelligence (AI) and signal processing to mimic human behavior. They are usually self-learning systems that use a variety of data, predictive analytics and natural language processing.
These days, customers expect their financial services institutions to treat them like retailers do – with personalized interactions and unique customer experiences. With the addition of cognitive computing to the company’s technology arsenal, the way in which banking works can be dramatically transformed.
Personal banking
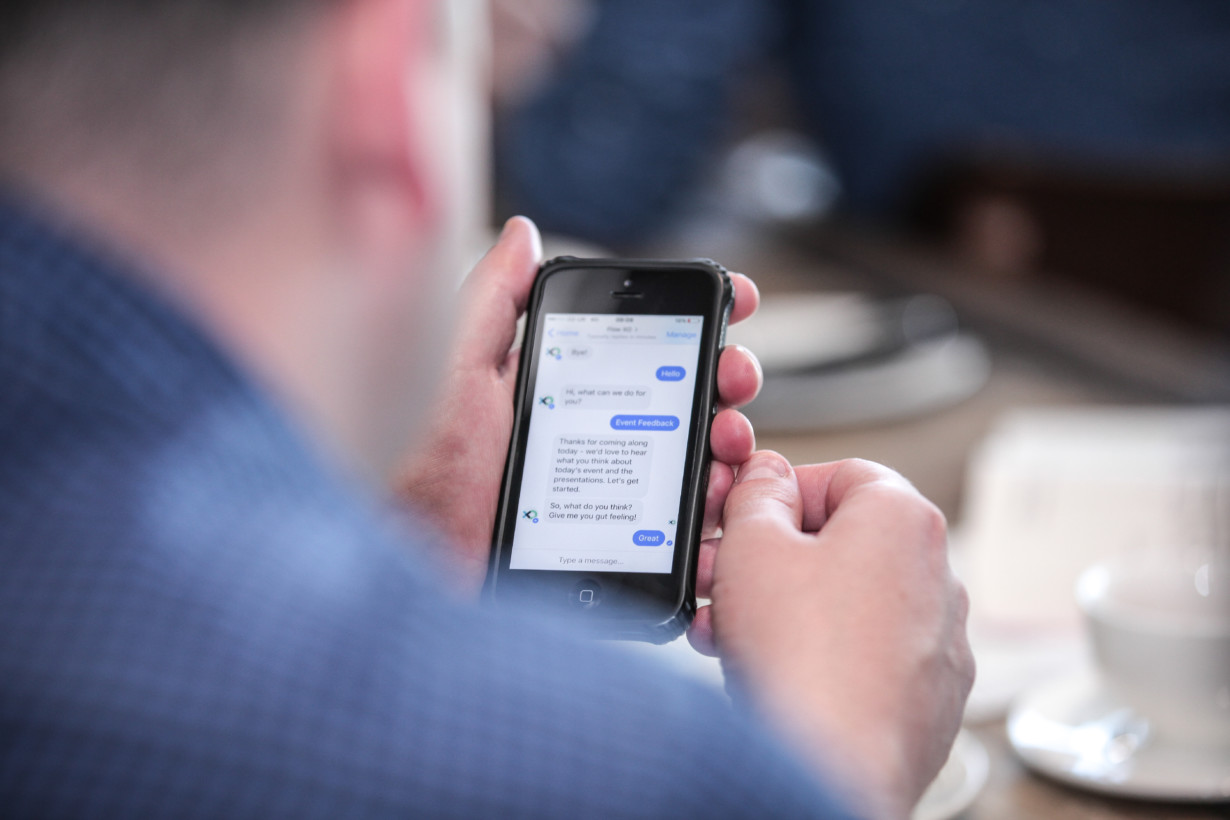
Payment Services Directive (PSD2), a revised key payments-related legislation in Europe due to take effect in 2018, is affecting the way retail banking operates in terms of payments. The revision is extending the scope of regulation to all payments where at least one party is in the EEA and tightening required security measures. However, most disruptive of all, it is introducing third party providers (TPPs) who can connect to bank accounts, retrieve information and in some cases initiate transactions.
With this directive mandating banks to ‘open up the bank account’ to external parties, a couple of banks have gone one step further and are implementing AI powered chatbots that are developed either in house or in partnership with third parties. The idea is that you can have a virtual assistant who knows all your banking data and will be able to service your everyday banking needs.
The servicing includes answering queries on your account balance and making transactions as well as give your more insight into your spending habits faster than a human will be able to. However, these bots could potentially go beyond what human personal bankers do and notify you if you spend more than usual on a certain product or category of products, suggest opportunities in real-time to reduce debt or save money, and alert you immediately if your credit score dips.
These bots can sit within a banking mobile application or as a standalone application in one of the bot supported messaging systems like Facebook, Slack, Telegram, WeChat etc. and dramatically enhance the customer experience with the bank because they don’t need to wait for a human customer service representative or personal banker to become available. As a result of this, the customer service arm of the business should see a shift from answering frequently asked questions to receiving more complex questions that would be a result of edge cases.
Banks need to account for this shift by providing specialized training and handing down more decision power to lower levels of customer service staff. Resolution times should in theory also be much faster because they won’t be bogged down by answering frequently asked questions. The caveat here is that whilst the customers get used to talking to chatbots, the customer service team might need to double as an educational team teaching their customers how to self-service via chat bot as well.
Wealth management advice (including robo advice)
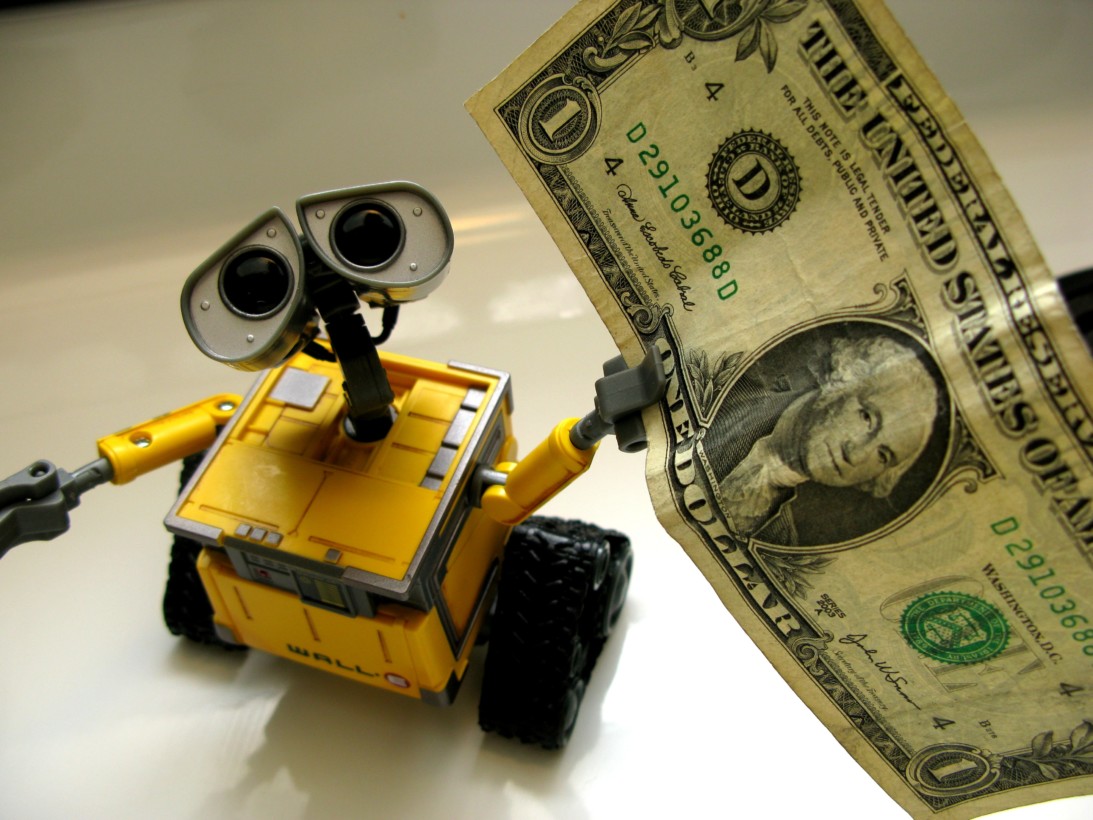
Wealth management has traditionally been a people oriented business, but with millennials preferring to interact with services on a digital level, it’s no surprise that wealth management has to go digital as well.
Robo advice, online financial advice or portfolio management requiring minimal human intervention emerged in 2008, typically allocate based on individual investment, risk preferences, and desired target return but are mostly directed towards exchange traded fund (ETF) portfolios. This type of product and advice method caters to the mass market audience as the minimum investment levels are affordable and in the range of thousands of dollars as opposed to tens of thousands of dollars. As ETFs are cheaper to run than active funds, the fees charged are also lower.
The problem with robo advice is that it is targeted to people who are already inclined to invest. It doesn’t offer the wealth manager’s personal touch of trying to educate and answer questions of a worried first time anxious customer or providing rationale for why a particular recommendation was made.
However, if cognitive capabilities such as artificial intelligence and natural language processing are deployed together, deploying a chat bot or voice bot could potentially mimic the interactions you’d have with a wealth manager much better. An example would be the robo advice bot telling you that you have an amount in cash that you haven’t touched for the past two years and seeing if you’d like to take a look at their investment products.
IBM research said that a large bank focused on investigating how cognitive capabilities can help customers select higher-value investments discovered that many of their customers make cash deposits because they are either unaware or uneducated about alternative investment options. By facilitating artificially intelligent personalized dialogue, the bank can help customers make better investment decisions based on their individual situations.
This gives consumers access to cheap or potentially free tailored digital wealth management advice. For banks and fund managers, this widens up their potential consumer base but also comes with an expectation of banks and fund managers to provide digital real-time client servicing.
Trading
It’s not a new concept to rely on computer algorithms to make trades. Preqin claims that around 1,360 hedge funds make the majority of their trades with the help of computer models. This is nine percent of all funds totaling a staggering $197 billion in combined assets under management. High frequency trading is even fully automated where computer algorithms have taken over human interaction.
Data scientists may apply traditional machine learning algorithms to solve complex problems. However, these models don’t adapt to new circumstances as they are somewhat static. This means that as the market changes, the models may not work as brilliantly, because they failed to account for the market change. However, with new techniques such as Deep Learning combined with cognitive computing, models are moving towards an artificially intelligent system that not only analyzes large amounts of data at speed, but also uses that data and it’s outcomes to improve themselves.
IBM did research into a European bank whose sales teams follow detailed approval processes to fully vet potential trades for complex transactions involving institutional clients. Since the process requires a lot of approvals relating to credit and market risk constraints, compliance issues and regulatory policies, it is very time consuming. Sometimes, the market changes in the middle of the process, rendering the initial recommendation obsolete. Utilizing a cognitive system can speed up information digestion of the policies and regulatory documents, enabling more opportune trade recommendations based on the latest information and market conditions.
The impact for customers will be performance related. Either the AI enhanced product will be better or worse than the traditional model. This will obviously depend on the AI and the fund. In theory, customers would also be served at a faster speed since AI could speed up much of the decision making process because of better outputs (results). Data prep and processing remains as it was. It’s not so that a recurrent neural network will gather the data by itself, that’s something humans still need to build. Although, in 20 years or so, there may be some automated feature extraction, we’re still a long way away from AI that does all this by itself. However, banks would be experiencing a huge change in their trading process and tread a fine line between determining what’s to be done by an expert and what’s to be done by an AI crafted by the expert.
In summary, cognitive computing has the potential to transform personal banking, wealth management and trading. Consumers can expect benefits to quality of information, products and services and speed of service if the banks choose to utilize AI enhanced digital services like bots.
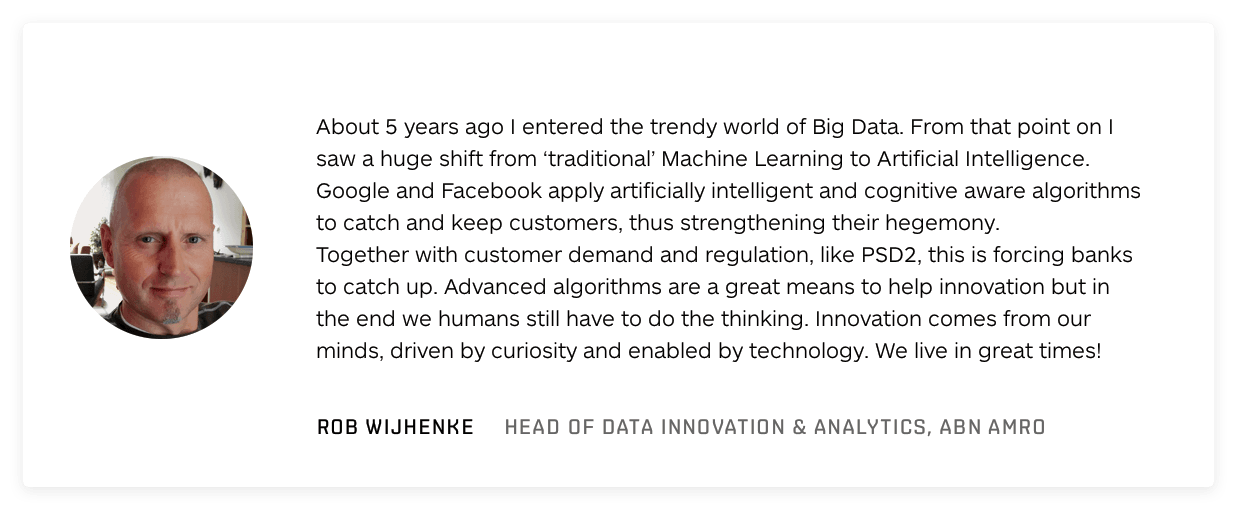
Get the TNW newsletter
Get the most important tech news in your inbox each week.
Whatever your specialism, with ABN AMRO your talent and creativity will help build the bank of the future. Find out what it’s like to work for ABN AMRO and learn more about their exciting job opportunities.
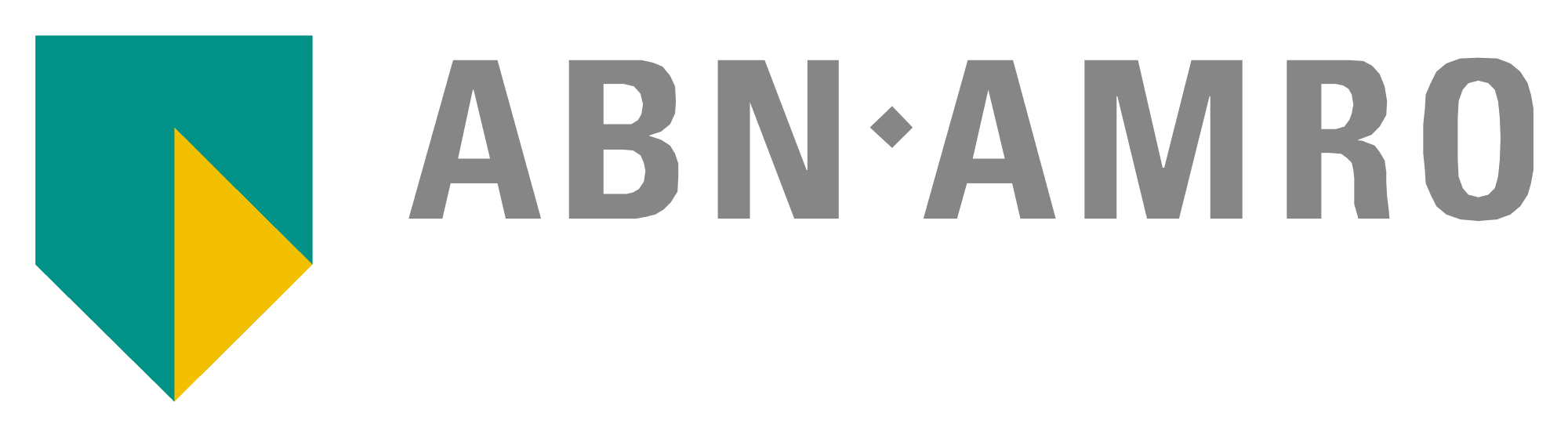